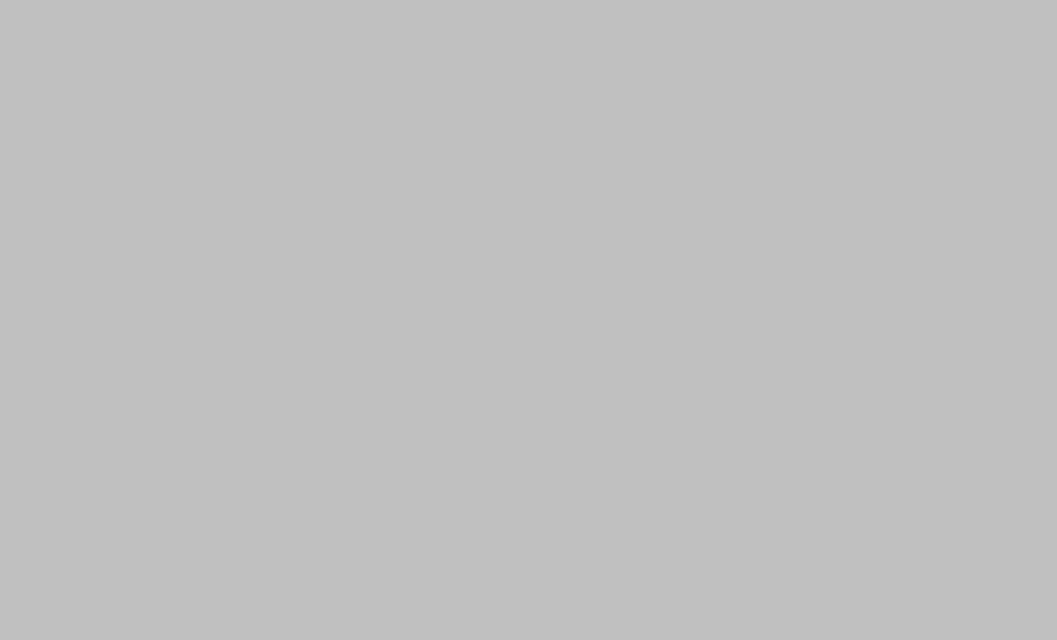
theguardian.com
AI: A Tool for Racial Justice?
AI's potential to reduce racism lies in its auditability, revealing hidden biases in data and allowing for systematic improvements, although careful attention to both technical and social considerations is crucial for equitable outcomes.
- What are the key technical and social challenges in developing and deploying AI systems that actively work to correct historical racial disparities?
- The inherent auditability of AI systems contrasts with the difficulty of addressing human bias directly. Systematic audits of AI decisions expose race-based disparities, forcing a confrontation with uncomfortable truths about societal biases embedded in historical data. This allows for the development of context-specific fairness metrics, moving beyond simplistic approaches.
- What specific steps can organizations take to ensure that AI systems are not only free from bias but also actively promote racial justice and equity?
- Future success hinges on both technical advancements and inclusive social processes. Technically, sophisticated fairness metrics are needed that account for the context (low vs. high stakes decisions) and historical inequities. Socially, diverse teams and community engagement are crucial in developing and deploying equitable AI systems.
- How can the inherent auditability of AI systems be leveraged to expose and mitigate racial biases in high-stakes decisions like healthcare and criminal justice?
- AI systems, while sometimes exhibiting bias, offer an unprecedented opportunity to address racial disparities by providing auditable records of decision-making, revealing hidden biases in data and institutions. This auditability allows for systematic testing and improvement of AI algorithms, leading to more equitable outcomes.
Cognitive Concepts
Framing Bias
The article frames AI's role in addressing racial bias as an opportunity rather than a threat. While acknowledging the existence of AI bias, the emphasis is on its potential to reveal and correct existing societal biases. The headline, although not explicitly provided, would likely contribute to this framing, emphasizing the positive potential. The introductory paragraphs clearly set this optimistic tone.
Language Bias
The language used is largely neutral and objective. While terms like "backlash against social justice initiatives" might carry some implicit connotations, the overall tone remains analytical and avoids loaded language. The article uses precise language to explain complex concepts and avoids inflammatory or emotional terms.
Bias by Omission
The article focuses primarily on the potential of AI to mitigate racial bias, but it could benefit from explicitly mentioning limitations or potential downsides of using AI in this context. For example, the data used to train AI systems could reflect existing societal biases, leading to perpetuation of inequalities. Additionally, the article doesn't discuss the possibility of AI being used to *increase* racial bias, either intentionally or unintentionally. While the article acknowledges the need for diverse voices in AI development, it doesn't delve into the potential for biased outcomes if these voices aren't adequately represented.
Sustainable Development Goals
The article highlights how AI, despite potential biases, can be used as a tool to identify and mitigate racial inequalities in various sectors like healthcare and criminal justice. AI's auditability allows for the detection and quantification of existing biases, paving the way for more equitable systems. The involvement of diverse voices in AI development is also emphasized, ensuring that solutions address the needs of affected communities.