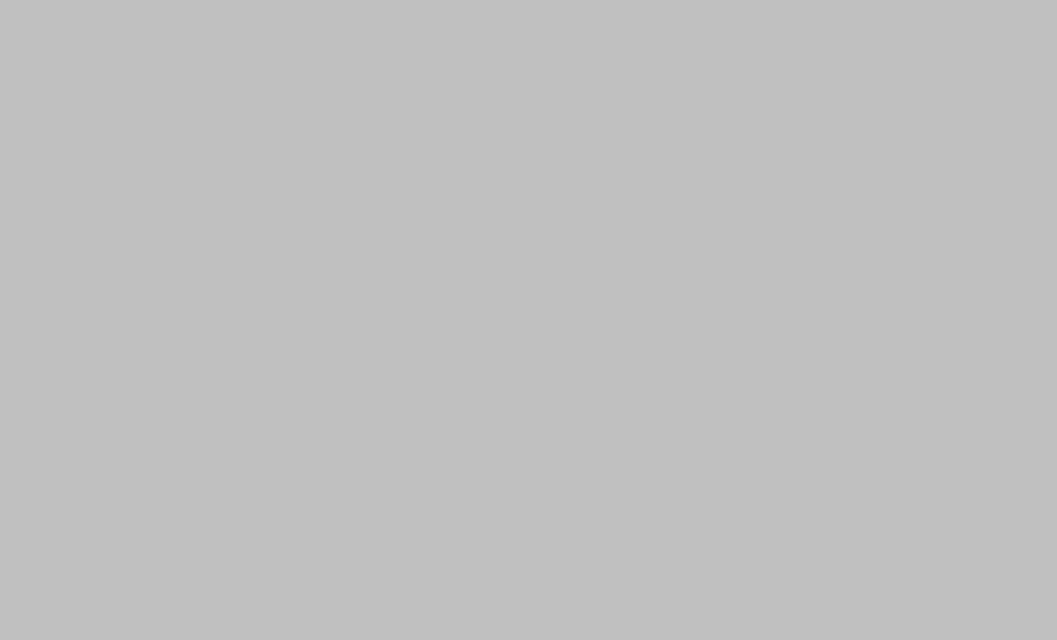
forbes.com
AI Advances Healthcare Diagnosis, but Challenges Remain
A panel discussion highlights AI's growing role in healthcare, with over 800 radiology applications improving diagnosis and reporting, but acknowledges that AI's capabilities are still developing and that challenges in data integration and access remain.
- What are the immediate impacts of AI on clinical diagnosis and patient care, based on the discussed applications and limitations?
- AI is significantly advancing healthcare, particularly in radiology, with over 800 applications aiding diagnosis and reporting, leading to faster and more effective patient outcomes. However, AI's capabilities are still developing and do not yet match human expertise.
- How does the integration of diverse data sets, such as genetic information and brain imaging, improve the understanding and treatment of neurological diseases?
- The integration of AI in healthcare research connects various data points—genetics, epigenetics, protein structures, brain activity—to create comprehensive models for improved diagnosis and treatment of neurological diseases. This integrated approach addresses the high failure rate of CNS drugs due to insufficient fundamental knowledge.
- What are the long-term implications of AI in healthcare, considering both the potential for improved prediction and prevention, and the challenges in accessibility and data integration?
- Future healthcare will likely transition from precision medicine to predictive and preventive approaches, leveraging AI's ability to analyze diverse data and predict potential health issues. This transition requires overcoming challenges in data integration and ensuring equitable access to AI-powered healthcare solutions.
Cognitive Concepts
Framing Bias
The framing is largely positive and emphasizes the potential benefits of AI in healthcare. The selection of quotes and the overall tone highlight the progress and exciting possibilities. While this is not inherently biased, it could create an overly optimistic view and downplay challenges or potential downsides. The headline itself, if there was one, would likely reflect this positive framing.
Language Bias
The language used is generally neutral and informative. However, phrases like "crack open this jewel" and "decipher our own signals at a neurological level" employ slightly sensationalistic language, suggesting a more exciting and dramatic narrative than might be strictly accurate or appropriate for a scientific topic. The overall tone is positive and enthusiastic, which, while not inherently negative, could be perceived as promoting the AI narrative without sufficient counterpoint.
Bias by Omission
The article focuses heavily on the advancements and potential of AI in healthcare, particularly in brain mapping and diagnosis. However, it omits discussion of potential drawbacks, limitations, or ethical concerns associated with AI in healthcare. There is no mention of the potential for bias in AI algorithms, data privacy issues, or the displacement of human healthcare workers. While brevity may necessitate some omissions, the lack of counterpoints weakens the overall analysis.
False Dichotomy
The article doesn't present a false dichotomy, but it does present a somewhat overly optimistic view of the progress and future potential of AI in healthcare without fully acknowledging the challenges and complexities involved. The narrative implicitly suggests a straightforward path towards seamless AI integration in healthcare, which may oversimplify the reality of implementation.
Sustainable Development Goals
The article discusses the application of AI in healthcare, specifically in improving diagnosis, reporting, and patient outcomes in radiology and neurology. AI-powered tools are aiding faster and more effective diagnoses, leading to better treatment and potentially improved patient health. The development of AI models for understanding brain diseases like Alzheimer's and Parkinson's also holds significant promise for improving treatment and quality of life.