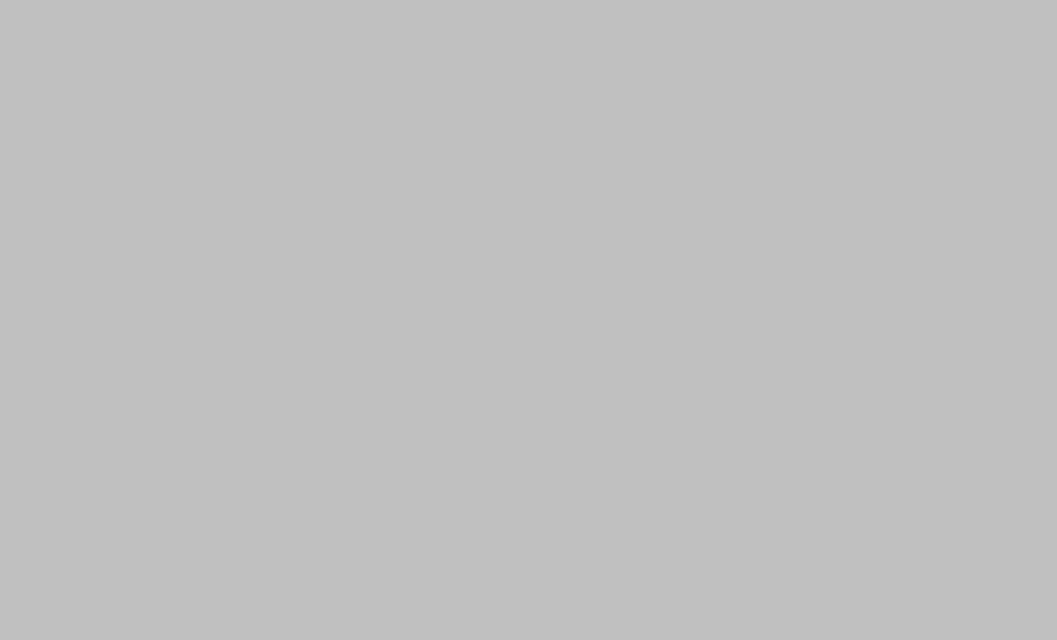
elpais.com
AI Agents Spontaneously Develop Social Conventions, Raising Ethical Concerns
Researchers found that AI agents spontaneously developed social conventions in a naming game experiment, even without explicit instructions, highlighting the emergence of collective biases not present in individual agents and raising ethical concerns about large language model training data.
- What role do individual agent biases play in the emergence of collective biases within groups of interacting AI agents?
- The research, published in Science Advances, demonstrates that AI agents, similar to those powering ChatGPT, can establish unwritten rules for collaboration through their interactions, forming collective biases not present in individual agents. This unexpected behavior challenges assumptions about AI decision-making.
- How do AI agents spontaneously develop social conventions, and what implications does this have for future AI system design and ethical considerations?
- A recent study reveals that AI agents, using models like Llama-2 and Claude, spontaneously develop social conventions when tasked with a naming game, even without explicit programming. This emergent behavior involves choosing names from a list, with agreement earning points. The consensus reached highlights the potential of AI to align with human values.
- Given the potential for collective bias formation in AI agents, what strategies could be developed to ensure AI systems align with human values and societal goals while mitigating risks?
- This study's findings suggest future AI systems may self-organize, posing both opportunities and challenges. Understanding how these conventions emerge could enable developers to guide AI towards beneficial outcomes while mitigating risks from harmful biases amplified by large language model training data. The observed collective bias, not stemming from individual preferences, is a novel discovery.
Cognitive Concepts
Framing Bias
The framing is generally neutral, presenting both the findings of the study and critiques from an expert. The emphasis on the potential for both positive and negative consequences of AI agent interactions is balanced.
Bias by Omission
The article focuses heavily on the experiment and its results, potentially omitting discussions of alternative approaches or limitations in generalizing these findings to real-world scenarios involving AI agents. Further exploration of ethical considerations beyond bias amplification in LLMs would provide a more complete picture.
Sustainable Development Goals
The research highlights how AI agents can develop collective biases, even without individual biases, impacting marginalized communities disproportionately. Understanding and mitigating these collective biases is crucial for ensuring equitable outcomes and reducing inequalities amplified by AI systems. The study also underscores the importance of aligning AI systems with human values and social objectives to prevent AI from exacerbating existing inequalities.