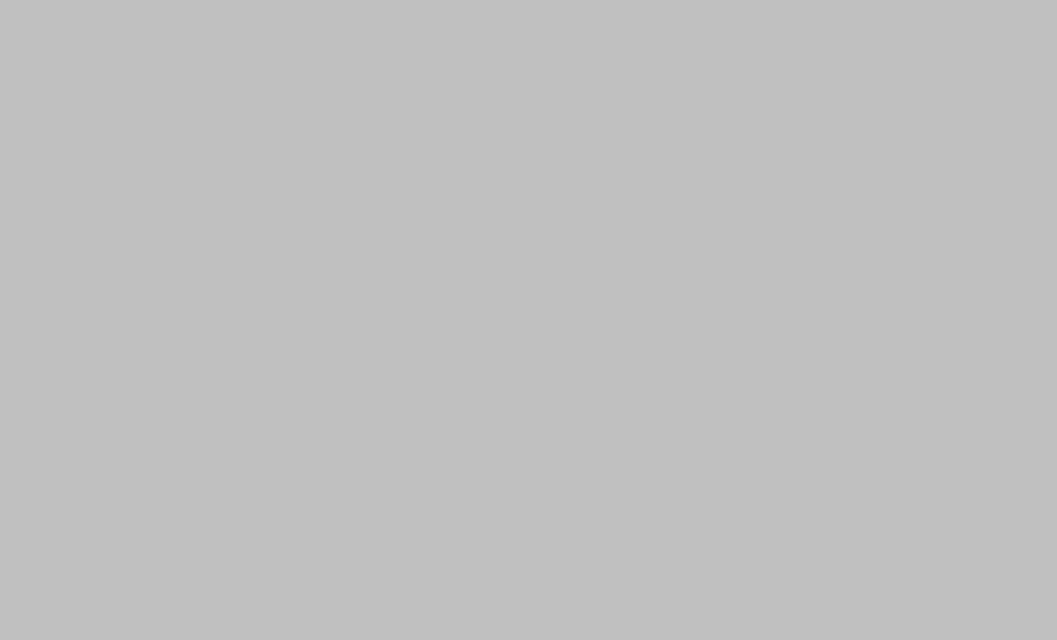
cbsnews.com
AI Algorithm Malfunctions in End-of-Life Care During COVID-19 Pandemic
An AI algorithm designed to help oncologists discuss end-of-life care with patients at the University of Pennsylvania Health System had its accuracy decrease by 7 percentage points during the COVID-19 pandemic, resulting in hundreds of missed opportunities for crucial conversations, according to a 2022 study.
- What are the immediate consequences of AI algorithm decay in the context of end-of-life care discussions for cancer patients?
- An AI algorithm designed to prompt doctors to discuss end-of-life care with cancer patients at the University of Pennsylvania Health System malfunctioned during the COVID-19 pandemic, resulting in hundreds of missed opportunities for crucial conversations. The algorithm's predictive accuracy decreased by 7 percentage points, highlighting the need for consistent monitoring of AI tools in healthcare.
- What systemic factors contributed to the algorithm's decline in accuracy during the COVID-19 pandemic, and how can these issues be addressed in future deployments?
- The algorithm's failure underscores a broader challenge in deploying AI in healthcare: the need for continuous monitoring and maintenance. Changes in underlying data, such as those that occurred during the pandemic, can significantly impact algorithm performance, leading to potentially harmful consequences if not addressed. This necessitates substantial investment in resources and personnel for algorithm validation and oversight.
- What long-term implications does this case have for the implementation and regulation of AI in healthcare, considering the resource demands of ongoing monitoring and validation?
- The incident demonstrates that the integration of AI into healthcare requires a robust infrastructure for ongoing evaluation and improvement. Without comprehensive monitoring and dedicated personnel, the potential benefits of AI may be offset by unforeseen errors and malfunctions, potentially jeopardizing patient care and increasing healthcare costs. Future AI applications in healthcare must prioritize rigorous validation and continuous performance monitoring to ensure safety and efficacy.
Cognitive Concepts
Framing Bias
The article frames AI in healthcare primarily through a negative lens, highlighting failures and challenges. While this is important, the framing might inadvertently discourage adoption of potentially beneficial AI technologies. The emphasis on errors and costs could overshadow the potential benefits for patients and healthcare providers. The headline and opening paragraphs immediately focus on the shortcomings of AI algorithms, setting a negative tone.
Language Bias
The article uses relatively neutral language, although words like "decayed," "failed," and "devastating" carry negative connotations. While these words accurately reflect the concerns discussed, consider using less charged language where possible to maintain objectivity. For example, instead of "failed hundreds of times," consider "in hundreds of instances, the tool did not prompt doctors."
Bias by Omission
The article focuses heavily on the challenges and failures of AI in healthcare, potentially omitting success stories or instances where AI has significantly improved patient care. While acknowledging limitations of space, a more balanced perspective on AI's impact would strengthen the piece. For example, the article could include data on successful AI applications in healthcare to provide a more nuanced picture.
False Dichotomy
The article doesn't present a false dichotomy, but it does emphasize the problems with AI implementation in healthcare without fully exploring the potential solutions or mitigating strategies. The narrative could benefit from including discussions about ongoing research, regulatory efforts, or best practices for AI development and deployment in healthcare.
Sustainable Development Goals
The article highlights the failure of AI algorithms in healthcare, leading to missed opportunities for crucial end-of-life discussions and potentially unnecessary chemotherapy. This negatively impacts the quality of care for cancer patients and undermines efforts to improve health outcomes.