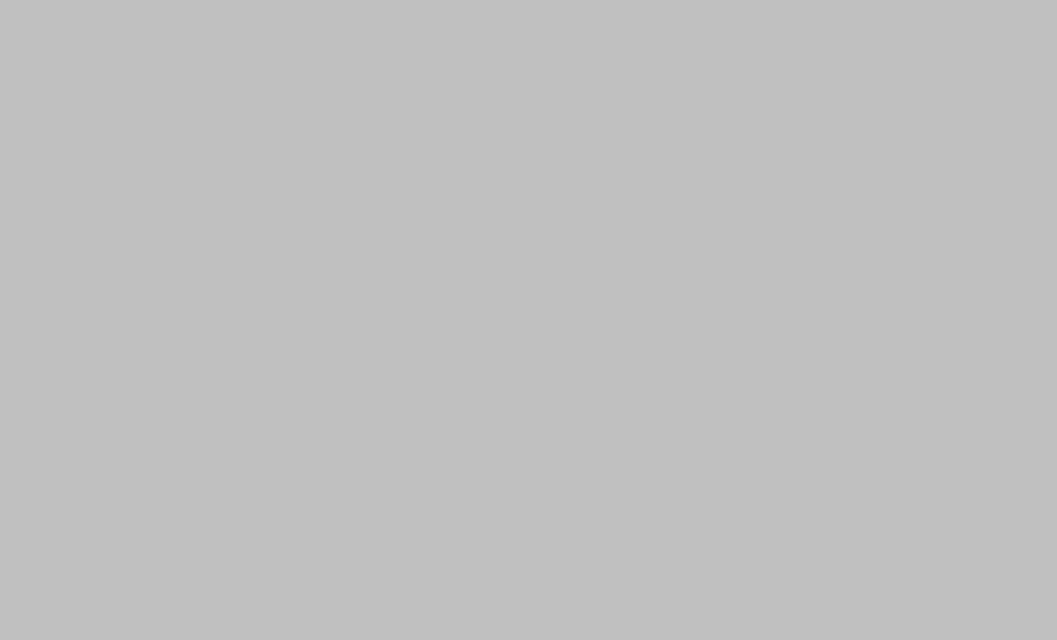
forbes.com
AI and Machine Learning Reduce Power Plant Losses
Global electricity transmission and distribution losses average 8–9%, but some countries experience losses exceeding 20%, impacting sustainable energy development. AI and machine learning offer solutions by optimizing efficiency and reducing losses across the power supply chain, with the global AI-driven energy management market projected to grow at a 21.2% CAGR from 2022–2030.
- How do AI and machine learning contribute to reducing specific types of losses in power plants, providing concrete examples?
- Technical losses stem from factors like heat dissipation in power plants (33-60% for natural gas, 30% for oil) and grid inefficiencies. Non-technical losses, like electricity theft, cause billions in lost revenue annually, especially in developing nations. AI addresses both, improving predictive maintenance and enabling accurate theft detection.
- What are the future implications of AI-driven energy management for sustainable energy development, particularly in developing countries?
- AI-driven predictive maintenance, using sensor data and machine learning, reduces unplanned downtime (e.g., Pacific Gas & Electric reduced downtime by 30%). AI-powered theft detection, analyzing smart meter data, provides accurate identification and prioritization for revenue recovery, particularly impactful in developing countries. The global AI-driven energy management market is projected to grow at a 21.2% CAGR from 2022-2030.
- What are the primary impacts of technical and non-technical losses in global power grids, and how do AI and machine learning offer solutions?
- Global electricity transmission and distribution losses average 8-9%, but some countries exceed 20%. These losses, categorized as technical (inefficiencies in systems) and non-technical (theft, inaccurate metering), hinder sustainable energy development. AI and machine learning offer solutions by optimizing efficiency and reducing losses across the power supply chain.
Cognitive Concepts
Framing Bias
The framing strongly emphasizes the positive impact of AI and machine learning in solving energy loss issues. While the challenges are acknowledged, the narrative leans heavily towards presenting AI as a primary solution. The introduction sets this tone by highlighting AI as a powerful tool to mitigate inefficiencies. This positive framing might overshadow the complexity and limitations of implementing AI solutions in diverse contexts.
Language Bias
The language used is generally neutral and objective, using descriptive terms for different types of losses and solutions. However, phrases like "powerful tools" and "rapid growth" might subtly convey a more positive tone than strictly neutral reporting would allow. Phrases such as "mounting financial losses" or "hinder efforts to transition" create a sense of urgency that could be replaced with more neutral language.
Bias by Omission
The article focuses heavily on technical and non-technical losses and the application of AI to solve them. However, it omits discussion of the social and political factors that contribute to these losses, such as energy poverty or regulatory challenges. The article also doesn't explore potential downsides or limitations of AI solutions, such as data bias or the cost of implementation. While the scope is broad, these omissions limit a complete understanding of the problem and its solutions.
False Dichotomy
The article presents a somewhat simplistic view of the problem by primarily focusing on the technical and non-technical dichotomy. It doesn't fully explore the interplay between these categories or acknowledge that some losses might have aspects of both. For example, aging infrastructure (technical) might be exacerbated by lack of maintenance due to financial constraints (non-technical).
Sustainable Development Goals
The article discusses using AI and machine learning to reduce power plant losses, which directly contributes to more efficient and sustainable energy production and distribution. Reducing losses decreases the overall energy needed to meet demand, thus lessening the environmental impact and improving energy affordability.