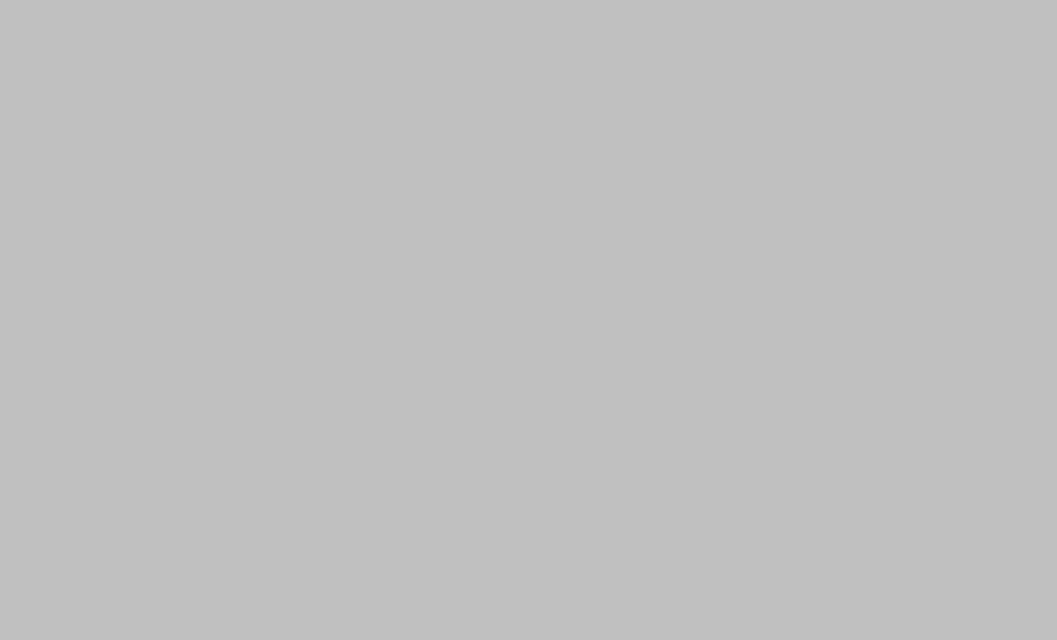
forbes.com
AI Bias: A Critical Business Challenge
Amazon's discriminatory AI recruiting tool highlighted the urgent need to address bias in AI; biased AI systems perpetuate societal inequalities, impacting recruitment, decision-making, and a company's bottom line; addressing bias requires diverse teams, robust testing, quality data, and human oversight.
- What is the most significant impact of biased AI systems on businesses?
- Amazon's biased AI recruiting tool, trained on historical data, amplified existing gender bias, forcing its abandonment. This incident exposed the urgent need to mitigate AI bias before it becomes deeply entrenched in business systems. The incident cost Amazon time and resources.
- How does the lack of diversity in AI development contribute to biased outcomes?
- AI systems often mirror societal biases, with studies showing 61% of performance feedback reflecting evaluator bias rather than employee performance. This biased data, used for AI training, creates a compounding effect leading to systematic bias in automated decisions impacting recruitment and other processes.
- What are the long-term consequences of failing to address AI bias in business applications?
- Failure to address AI bias will result in missed opportunities, legal issues, reputational damage, and restricted market reach due to a failure to connect with diverse customer segments. Proactive bias mitigation is crucial for long-term business success and ethical AI development.
Cognitive Concepts
Framing Bias
The article frames the issue of AI bias predominantly through a negative lens, focusing on the problems and risks associated with biased AI systems. While this is important, it could be balanced with more positive examples of companies actively addressing the issue and achieving successful outcomes. The focus on the negative might inadvertently discourage proactive efforts from businesses.
Language Bias
The language used is generally neutral and objective, although terms like "cautionary tale" and "troubling reality" introduce a slightly negative connotation. These phrases could be replaced with more neutral terms such as "illustrative example" and "recent findings." The use of the phrase "garbage in, garbage out" is an informal expression which might not be appropriate in a formal analysis. A more formal and neutral explanation of the importance of data quality could be provided.
Bias by Omission
The article does not discuss the specific algorithms used in the biased AI recruiting tool, which could provide further insight into the nature of the bias. There is also no mention of the legal ramifications faced by Amazon or other companies due to biased AI, which would add valuable context.
False Dichotomy
The article presents a clear dichotomy between ethical concerns and business implications of AI bias, implying these are separate issues. However, ethical considerations often directly impact a company's bottom line through reputational damage, legal risks, and limited market reach. The article could benefit from a more nuanced discussion that integrates these aspects.
Gender Bias
The article highlights the underrepresentation of women in AI development, citing the statistic that only 22% of AI professionals are women. This is presented as a key factor contributing to AI bias. However, the article does not delve into the specific ways gender bias manifests in AI systems beyond recruitment. A deeper analysis of how gender stereotypes might influence other AI applications would strengthen the analysis.
Sustainable Development Goals
The article highlights the issue of gender bias in AI recruitment tools, advocating for the diversification of AI development teams and the implementation of bias testing protocols to mitigate gender inequality in the tech industry. The emphasis on including diverse perspectives in data collection and AI system audits directly addresses SDG 5, aiming to achieve gender equality and empower all women and girls. Solutions such as using synthetic data to balance underrepresented groups directly contribute to fairer outcomes.