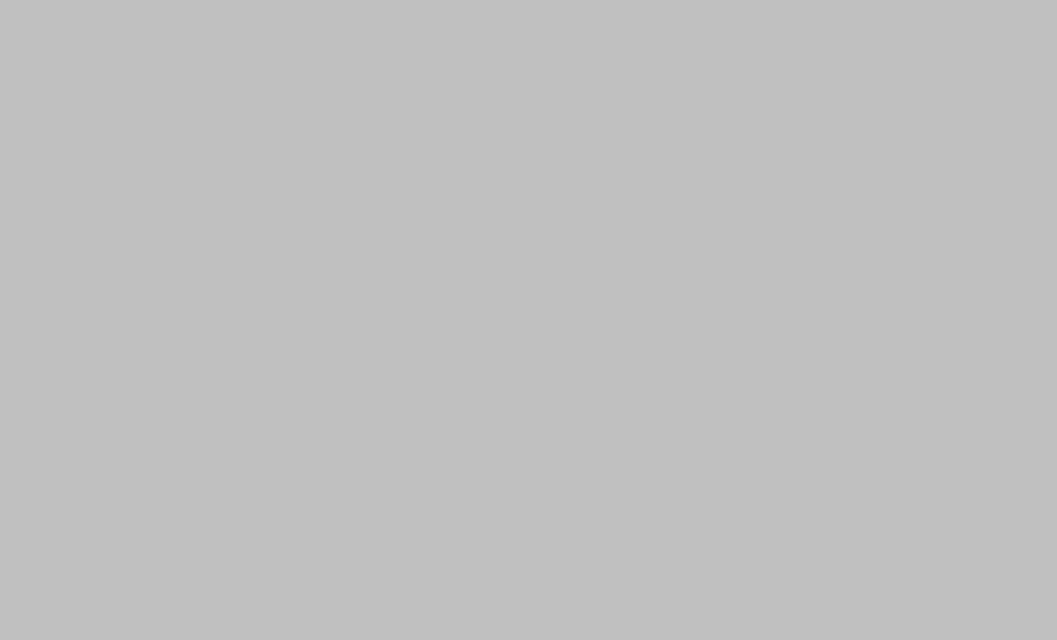
immobilier.lefigaro.fr
AI Bias in Housing: $2 Million Settlement for Erroneous Rental Rejection
An AI-powered tenant screening system, SafeRent, unfairly rejected Mary's rental application due to a low score, prompting a $2 million settlement and a five-year ban on using the system for voucher holders; highlighting AI bias in housing.
- How did SafeRent's AI algorithm fail to consider relevant financial information, and what systemic issues does this expose regarding the use of AI in housing decisions?
- SafeRent's AI algorithm considered Mary's debt but apparently disregarded her 17-year history of on-time rent payments, a landlord attestation, and a low-income housing voucher. This oversight led to her losing the apartment and highlights potential biases in AI-driven rental screening.
- What immediate impact did the AI-powered tenant screening system have on Mary's housing application and what broader implications does this have for fair housing practices?
- In May 2021, Mary, a security guard, was denied a rental application by a software program called SafeRent, which uses an AI-powered tenant selection tool. SafeRent assigned Mary a score of 384, below the required 443, resulting in her application's rejection. The scoring rationale remains undisclosed.
- What are the long-term implications of this case for the regulation and ethical use of AI in tenant screening, considering the potential for bias and discrimination against minority groups?
- This case reveals the discriminatory impact of opaque AI algorithms in housing. The $2 million settlement and SafeRent's agreement to cease using its scoring system for voucher holders for five years demonstrate the legal and financial consequences of biased AI and suggests a broader need for algorithmic transparency and accountability in housing.
Cognitive Concepts
Framing Bias
The headline and introduction immediately frame the AI as the antagonist, setting a negative tone. The emphasis on Mary's case and the large settlement amount may disproportionately highlight the negative impacts of AI while minimizing potential benefits. The article also focuses on the flaws of AI, neglecting possible merits of using AI for efficient tenant screening, if done correctly.
Language Bias
The article uses loaded language such as "capoter une location" (which translates roughly to "sabotage a rental"), "sanctionné" (sanctioned), and "discriminé" (discriminated). These words carry strong negative connotations. More neutral terms like "rejected," "flawed," or "controversial" could be used to maintain objectivity. The description of the algorithm's score as "absurdities" is also a subjective judgment.
Bias by Omission
The article omits details about how SafeRent's algorithm was trained and the specific data points used in scoring, limiting the ability to fully assess the algorithm's fairness and accuracy. The lack of transparency makes it difficult to determine if other factors beyond debt were considered or if biases were unintentionally introduced during the development process. The article also doesn't explore the broader societal implications of using AI in tenant screening.
False Dichotomy
The article presents a false dichotomy by framing the issue as AI versus human judgment. While AI may have flaws, human decision-making also has biases. The focus should be on improving the AI and ensuring transparency, not discarding AI entirely.
Sustainable Development Goals
The lawsuit and subsequent settlement demonstrate a step towards addressing algorithmic bias in housing, which disproportionately affects marginalized communities. The $2 million settlement and SafeRent's agreement to change its practices represent a positive impact on reducing inequality in access to housing.