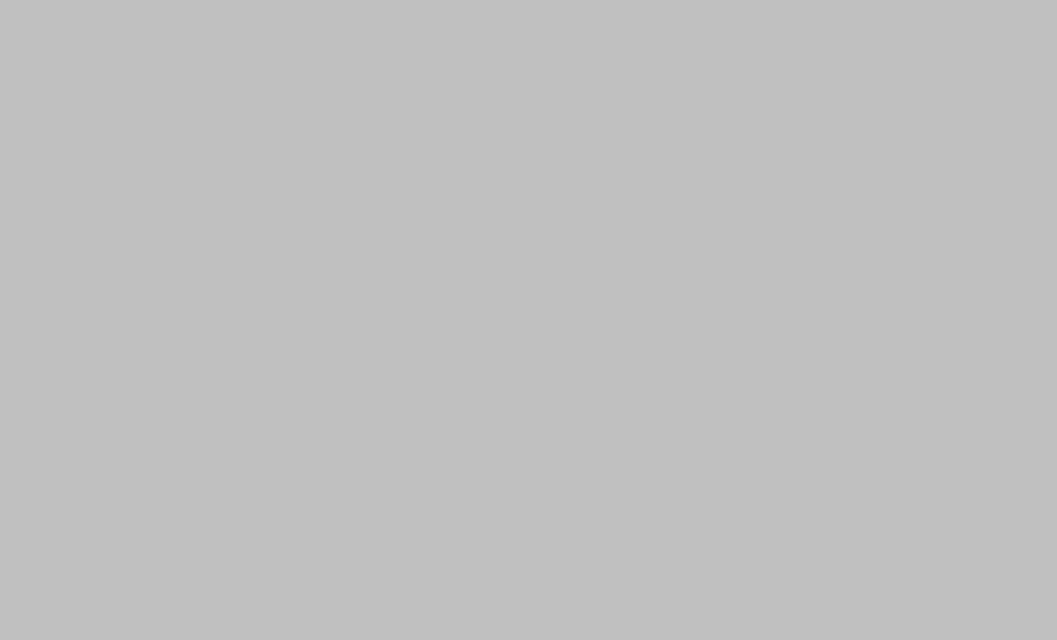
forbes.com
AI Data Center Spending to Hit $600 Billion by 2027
UBS predicts $600 billion in AI data center spending by 2027, driven by increased usage, scaling laws, and complex inferencing, with synthetic data addressing data scarcity and IP issues; this will lead to hardware innovation and potentially reinvent business models.
- What are the key factors driving the massive projected increase in AI data center spending by 2027?
- UBS CIO Ulrike Hoffmann-Burchardi predicts $600 billion in AI data center spending by 2027, surpassing general-purpose data center spending due to increased usage, scaling laws, and complex inferencing requiring more compute. Synthetic data will address data scarcity and IP issues in training AI models.
- What are the potential long-term impacts of AI adoption on business models, workforce dynamics, and overall economic growth?
- The integration of AI will fundamentally reshape industries. Companies with an "AI-first" mindset and proprietary data will lead, potentially reinventing business models and creating new revenue streams. However, the rapid pace of change necessitates proactive adaptation and strategic planning.
- How will the use of synthetic data mitigate challenges related to data acquisition and intellectual property in AI development?
- Hoffmann-Burchardi's predictions highlight the escalating demand for AI infrastructure, driven by the Jevons Paradox and advancements in model training and inferencing. This increased spending will fuel hardware innovation, particularly in GPUs, while simultaneously lowering costs and improving efficiency for businesses adopting AI.
Cognitive Concepts
Framing Bias
The article frames AI's impact primarily through the lens of financial investment and market trends. The focus on data center spending, hardware advancements, and stock market predictions prioritizes a business-centric perspective, potentially downplaying the social and ethical consequences of widespread AI adoption. The headline itself, while not explicitly biased, sets a tone of anticipating financial gains.
Language Bias
The language used is generally neutral, but phrases like "AI race" and "boom" carry a slightly positive and optimistic tone, suggesting inevitable success rather than balanced analysis of potential challenges. Words like "rescue" (in relation to synthetic data) introduce an element of subjective interpretation.
Bias by Omission
The article focuses heavily on the predictions of Ulrike Hoffmann-Burchardi and the financial implications of AI integration, potentially omitting other perspectives on the societal or ethical impacts of AI development. There is no mention of potential job displacement beyond a brief discussion of cost reduction strategies. The lack of diverse viewpoints might limit the reader's understanding of the broader implications of AI.
False Dichotomy
The article presents a somewhat simplistic dichotomy between cost reduction (through job losses) and revenue improvement as the only two paths for companies responding to AI. It overlooks the possibility of other strategies or a more nuanced approach that balances cost optimization with employee retention and ethical considerations.
Gender Bias
The article features only one prominent female expert, Ulrike Hoffmann-Burchardi. While this doesn't inherently represent bias, it raises the possibility of underrepresentation of female voices in the AI field. The lack of gender diversity in the cited sources might affect the breadth of perspective presented.
Sustainable Development Goals
The article discusses the significant increase in spending on AI data centers, advancements in AI hardware (like Groq 3 surpassing existing models), and the use of AI in software development (Google's 25% AI-written codebase). These advancements directly contribute to technological innovation and improved infrastructure for AI development and deployment, aligning with SDG 9.