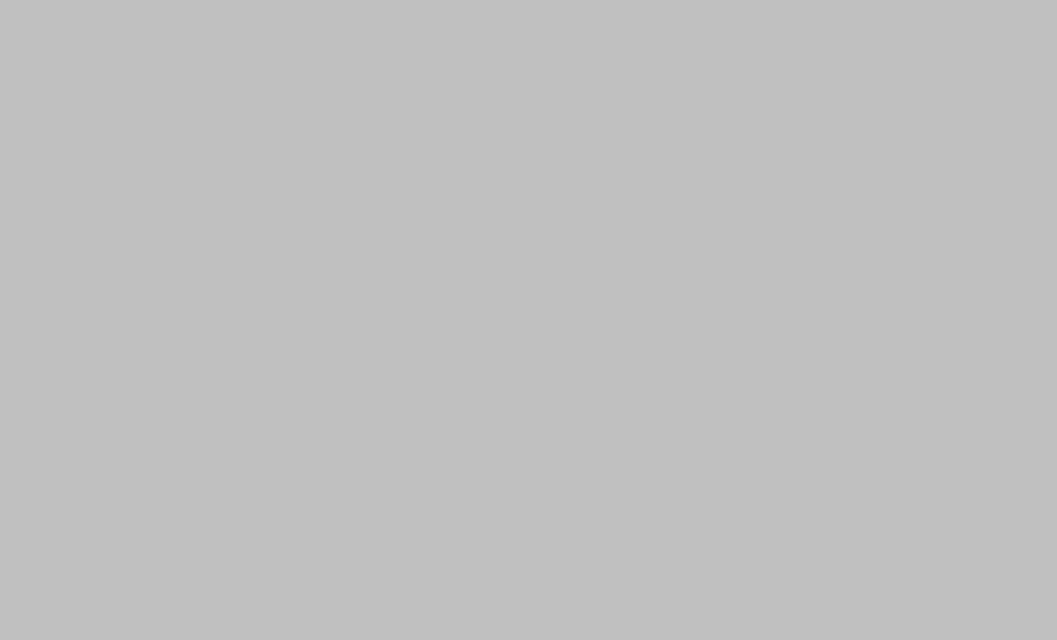
forbes.com
AI Deployment Challenges: Overcoming Unstructured Data Hurdles
AI's transformative potential is hampered by difficulties in managing the vast quantities of unstructured data within enterprise IT systems, requiring robust data engineering and management frameworks for successful implementation and measurable returns.
- What are the main obstacles preventing widespread successful AI deployment in enterprises?
- AI-driven advancements are transforming various sectors, from healthcare to construction, by enhancing product quality and reducing costs through real-time flaw detection in manufacturing. However, successful AI deployment faces significant challenges related to unstructured data management.
- How can organizations effectively manage and utilize the substantial amount of unstructured data crucial for AI functionality?
- The primary obstacle to effective AI implementation is the vast amount of unstructured data (90% of all data) within enterprise IT stacks. Efficient AI tools require synthesized and curated data, necessitating robust data engineering processes involving exploration, cleansing, normalization, and organization.
- What future strategies and considerations are essential for organizations to fully harness the potential of AI while addressing ethical and regulatory concerns?
- Future success with AI hinges on a holistic data engineering approach, encompassing data provenance, preparation, and proliferation. Organizations must establish strong data management frameworks for seamless data ingestion and accessibility to maximize AI tool effectiveness and achieve measurable returns, focusing on business value and regulatory compliance.
Cognitive Concepts
Framing Bias
The article frames the narrative around the difficulties and complexities of enterprise AI deployment, emphasizing the challenges related to unstructured data and data engineering. This framing, while accurate in highlighting a crucial aspect, might unintentionally downplay the significant potential benefits of AI and create a sense of overwhelming difficulty for potential adopters. The headline and introduction both focus on the obstacles, not the success stories, which could shape the reader's perception negatively.
Language Bias
The article uses relatively neutral language. However, the repeated use of phrases like "magic," "impossible-to-find flaws," and "crystal clear" contributes to a slightly sensationalized tone. While not overtly biased, these expressions could subtly influence reader perception by highlighting the positive aspects of AI more forcefully than the challenges.
Bias by Omission
The article focuses heavily on the challenges of AI deployment within enterprises, particularly concerning unstructured data. While it mentions the benefits of AI in manufacturing and media, it omits discussion of potential negative consequences or ethical concerns related to AI implementation, such as job displacement or algorithmic bias. This omission might limit readers' understanding of the broader implications of AI.
False Dichotomy
The article presents a false dichotomy by framing the choice as either 'AI is magic' or 'AI deployment is a complex data engineering challenge.' This oversimplifies the reality of AI implementation, which involves various factors beyond data engineering, such as model selection, ethical considerations, and regulatory compliance. The article doesn't adequately explore the nuances and intermediate stages between these two extremes.
Sustainable Development Goals
The article discusses the use of AI in manufacturing to improve product quality and reduce costs. This directly contributes to SDG 9 (Industry, Innovation, and Infrastructure) by promoting industrial efficiency and innovation. AI-driven improvements in manufacturing processes lead to better products and increased productivity, supporting economic growth and infrastructure development.