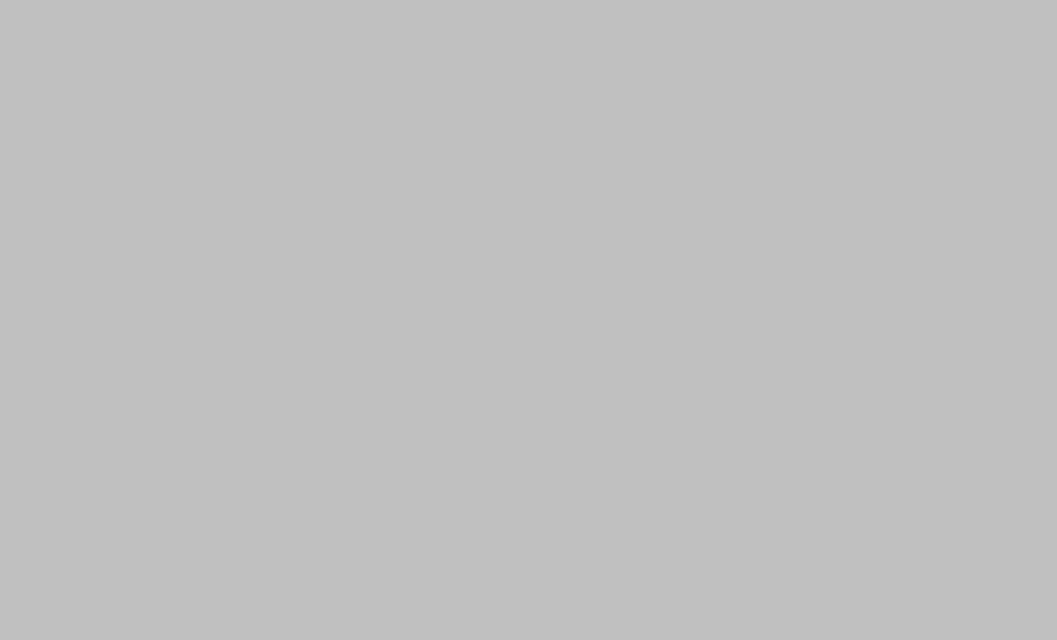
europe.chinadaily.com.cn
AI Developers Acknowledge Lack of Understanding of Generative AI Functionality
Leading AI developers acknowledge a significant gap in understanding how generative AI functions, unlike traditional software; the field of mechanistic interpretability is rapidly developing to address this, with potential breakthroughs expected within two years to mitigate risks in high-stakes applications.
- What are the primary concerns regarding the lack of understanding of how generative AI models function?
- Even the creators of generative AI admit a lack of understanding regarding its internal processes, a situation unprecedented in technological history. Unlike traditional software, generative AI models independently find solutions after initial prompting, making their decision-making opaque. This lack of comprehension poses significant risks.
- How is mechanistic interpretability contributing to our understanding of generative AI, and what are its potential benefits?
- The field of mechanistic interpretability, aiming to reverse-engineer AI models, is gaining traction due to the potential for enhanced AI capabilities and inherent intellectual intrigue. Researchers examine not only outputs but also the computational steps taken during query processing to uncover the reasoning behind AI's responses. This is crucial given the potential for errors to have significant impacts.
- What are the potential consequences of deploying highly intelligent AI models without fully understanding their inner workings, and what steps are being taken to address this?
- Within two years, significant progress is anticipated in deciphering AI's inner workings, driven by advancements in mechanistic interpretability. Full comprehension of AI is crucial for safe and responsible deployment in high-stakes applications like national security, where even minor errors can have significant consequences. The ability to understand and control AI is vital for shaping its impact on humanity.
Cognitive Concepts
Framing Bias
The framing emphasizes the mystery and potential danger of AI, using phrases like "digital minds" and "race against time." The headline itself focuses on the lack of comprehension. This framing could alarm readers and underplay potential benefits or less alarming aspects of AI development. The selection and ordering of quotes also support this negative framing, starting with expressions of concern and uncertainty.
Language Bias
The language used is generally neutral but leans towards dramatic descriptions, such as "unprecedented in the history of technology" and "race against time." These phrases create a sense of urgency and potential danger, which while perhaps factually accurate, aren't strictly neutral. More neutral alternatives might be "novel in technological history" and "significant challenge.
Bias by Omission
The article focuses heavily on the lack of understanding of AI's inner workings, but omits discussion of potential societal benefits beyond national security. It also doesn't explore dissenting viewpoints on the timeline for achieving AI comprehension or the urgency of the situation. While brevity is a factor, the omission of these perspectives limits the reader's ability to form a fully informed opinion.
False Dichotomy
The article doesn't explicitly present a false dichotomy, but the emphasis on the urgency of understanding AI before its widespread implementation could implicitly create a sense of urgency that overshadows potential benefits or alternative approaches to AI development.
Gender Bias
The article features several male experts in the field of AI. While this likely reflects the current gender demographics in the field, the lack of female voices contributes to an overall implicit bias. The analysis does not offer any explicit gendered language or stereotypes.
Sustainable Development Goals
The article highlights advancements in mechanistic interpretability, a field focused on understanding how AI models work. This research directly contributes to innovation in AI, a key aspect of SDG 9. Improved understanding will lead to more robust and reliable AI systems, fostering innovation across various sectors.