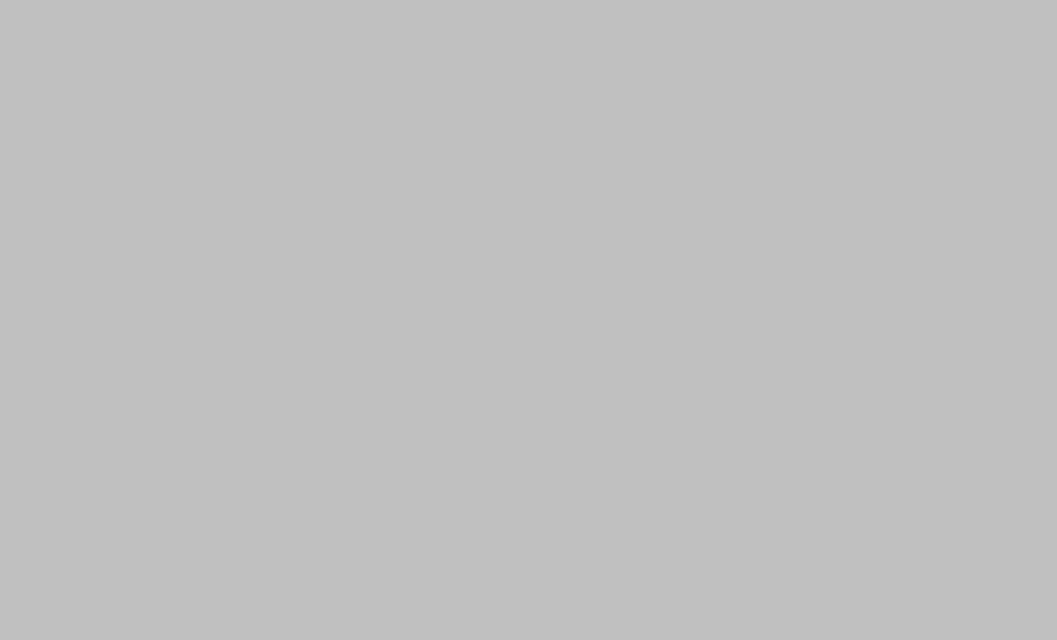
lexpansion.lexpress.fr
"AI Development Slowdown: Data Scarcity and Rising Costs Challenge the Scale Rule"
"OpenAI's latest AI model, Orion, failed to meet expectations, along with Google's Gemini and Anthropic's Claude 3.5 Opus, challenging the 'scale' rule in AI development; the limitations stem from the scarcity of high-quality data and increasing costs, leading to a shift towards strategic partnerships and innovative model designs."
- "How are leading AI companies addressing the scarcity of high-quality training data, and what are the long-term implications of these strategies?"
- "The slowing progress of leading AI companies like OpenAI, Google, and Anthropic challenges the long-held belief that increased computing power and data automatically lead to proportionally larger improvements in AI capabilities. This slowdown has significant implications for investment decisions, as the costs of training increasingly large models are escalating rapidly. The need to find and utilize high-quality data is paramount to future AI advancements.", "The challenge of finding high-quality data is critical because repetitive or biased content significantly reduces the performance of AI models. This scarcity is driving a shift in strategy, with companies like OpenAI investing in partnerships with content publishers to access exclusive, high-quality data sources. This approach is more expensive and time-consuming than simply scraping data from the web, but promises better results.", "While the cost and limitations of data acquisition are significant challenges, several innovative approaches are emerging. The "mixture-of-experts" method, which combines specialized sub-networks, appears to be a promising path. This approach allows for more efficient use of data and computational resources, and may overcome some limitations associated with simply scaling up model size."
- "What are the primary challenges hindering the development of more powerful AI models, and what are the immediate consequences for leading AI companies?"
- "OpenAI's latest model, Orion, has fallen short of expectations, struggling with programming and reasoning tasks. This, coupled with similar setbacks for Google's Gemini and Anthropic's Claude 3.5 Opus, challenges the 'scale' rule in AI development. The slowing progress highlights the increasing costs and data limitations faced by leading AI companies.", "The limitations stem from the dwindling supply of high-quality, unused data for training. While synthetic data generation is possible, it lacks diversity. Estimates suggest that all publicly available text could be exhausted within the next decade. OpenAI's strategic partnerships with content publishers exemplify the shift towards slower, more expensive, high-quality data acquisition.", "The future of AI development may lie in refining existing models through "post-training" and "mixture-of-experts" approaches. Post-training leverages human feedback and expert data labeling to enhance model performance. The mixture-of-experts technique involves combining specialized sub-networks for improved task execution. This evolving landscape indicates that brute-force scaling may not be the only path to progress. Increased competition from Chinese AI companies adds further pressure."
- "What are the potential future impacts of the current limitations on AI development, and how might these limitations reshape the competitive landscape and the direction of AI research?"
- "The slowdown in AI advancements could lead to a consolidation of the market as only the companies with access to large sums of capital can continue to invest in the development of increasingly complex models. This may result in a few dominant players controlling the future direction of AI. The increasing competition from Chinese AI companies, who are demonstrating efficiency in model development, adds another layer of complexity.", "The shift from simple data scraping to strategic partnerships with content providers will have significant implications for the publishing industry and intellectual property rights. These partnerships may lead to new business models and revenue streams for content creators. However, the need to maintain data quality while ensuring diversity of sources is crucial to avoid biases or inaccuracies in future models. ", "The limitations currently faced by leading AI companies in the data and computational power domains may also lead to more focus on refining existing models, exploring innovative architectural designs, and leveraging novel training methods. This shift in strategy could yield significant benefits in terms of cost-effectiveness, accuracy, and efficiency."
Cognitive Concepts
Framing Bias
The headline (if any) and introduction likely set a negative tone by focusing on the difficulties faced by major AI companies. The article's structure emphasizes the challenges and slowdowns more than any potential breakthroughs, shaping the reader's understanding towards pessimism about the future of AI development. The inclusion of financial figures for AI model training costs further reinforces this negative framing.
Language Bias
The article uses phrases such as "less réjouissantes" (less cheerful) and "rendements décroissants" (decreasing returns) to describe the progress in AI, subtly conveying a sense of disappointment and slowdown. More neutral language, such as "progress has been slower than anticipated" or "efficiency gains have plateaued", would be less subjective.
Bias by Omission
The article focuses heavily on the challenges faced by leading AI companies like OpenAI, Google, and Anthropic, but omits discussion of other significant players in the AI field and their progress. This omission might lead readers to believe that the entire industry is facing a slowdown, when this may not be entirely true. The lack of data on smaller companies' achievements could be a limitation of scope, but it still contributes to a potentially biased perspective.
False Dichotomy
The article presents a somewhat false dichotomy by implying that the only way to advance AI is through ever-increasing scale and data. While the challenges with scaling are highlighted, alternative approaches, such as focusing on more efficient algorithms or improving data quality instead of quantity, are not thoroughly explored.
Gender Bias
The article primarily focuses on the actions and statements of male leaders in the AI industry (Sam Altman, Dario Amodei, Kai-Fu Lee). While this might reflect the current leadership demographics, it could inadvertently reinforce gender bias by overlooking the contributions of women in the field. More balanced representation of genders in the discussion would improve the analysis.
Sustainable Development Goals
The article discusses advancements and challenges in AI model development, highlighting innovation in model architecture (e.g., "mélange d'experts") and the ongoing pursuit of more efficient training methods. This directly relates to SDG 9, which promotes building resilient infrastructure, promoting inclusive and sustainable industrialization and fostering innovation.