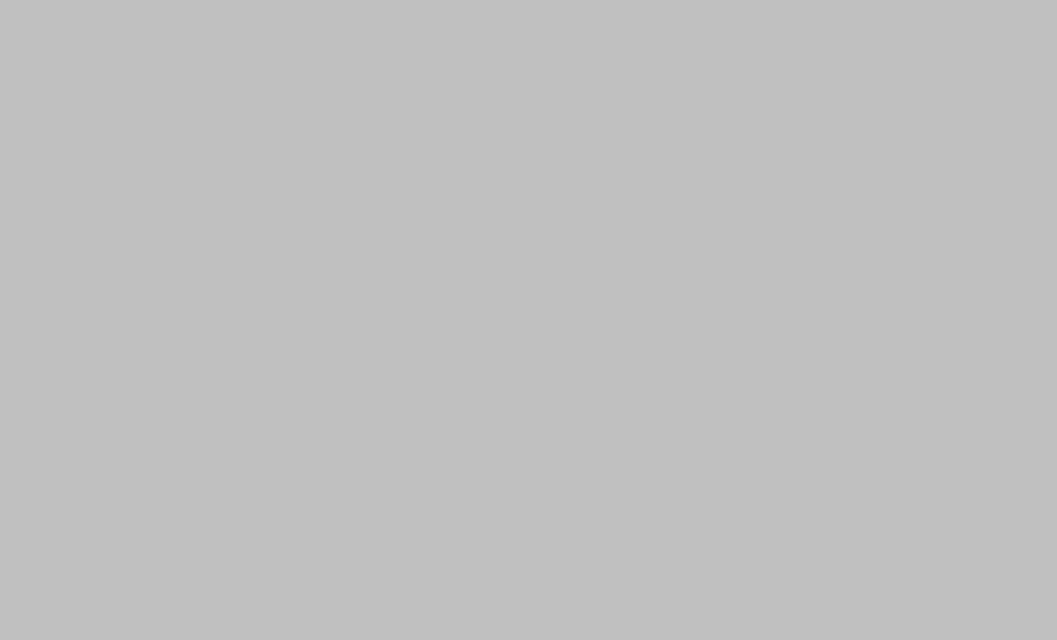
bbc.com
AI Heart Models Improve Medical Device Safety
AI-generated digital twins are improving medical device safety by enabling more inclusive and thorough testing across diverse populations, potentially reducing the thousands of deaths and millions of injuries caused by medical devices annually, as shown by a 2018 investigation.
- How do AI-generated digital twins improve the safety and inclusivity of medical device testing compared to traditional methods?
- AI-generated synthetic hearts, or digital twins, are being used to test implantable cardiovascular devices, offering more thorough testing across diverse populations than traditional methods allow, potentially reducing medical device-related deaths and injuries. This approach allows for testing under various conditions (e.g., different blood pressures, disease progressions) to evaluate device efficacy and safety more comprehensively.
- What are the key advantages of using AI digital twins for medical device manufacturers, considering both safety and economic factors?
- The use of AI in creating digital twins addresses limitations in clinical trial data, particularly the underrepresentation of diverse populations. By simulating various patient anatomies and physiological responses, AI enhances device testing, leading to more inclusive and safer medical devices. This helps mitigate risks associated with insufficient testing, as highlighted by past reports of significant deaths and injuries from medical devices.
- What are the potential limitations or biases in using AI-powered digital twins, and how can these be addressed to ensure fair and accurate representation of diverse patient populations?
- Future implications include wider adoption of AI-powered digital twins in medical device development, leading to improved safety and efficacy. This technology's ability to reduce testing time and costs could accelerate the development of new medical devices, benefiting patients globally. However, challenges remain in addressing data biases to ensure equitable representation in digital twin models.
Cognitive Concepts
Framing Bias
The article's framing is largely positive towards AI-powered digital twins, emphasizing their potential benefits in terms of safety, efficiency, and inclusivity. The headline and introduction highlight the transformative potential of this technology, setting a positive tone that persists throughout the article. While acknowledging some limitations, the overall framing strongly favors the advantages of AI.
Language Bias
The language used is generally positive and enthusiastic about the potential of AI digital twins. Words and phrases such as "transformative," "revolutionary," and "cutting-edge" are frequently used, creating a sense of excitement and optimism that might not be entirely warranted given the technology's relative novelty and potential limitations. More neutral language could be used to present a balanced perspective.
Bias by Omission
The article focuses heavily on the positive aspects of AI in medical device testing, mentioning the potential for reducing deaths and injuries caused by medical devices. However, it downplays or omits potential downsides or limitations of AI-generated digital twins, such as the risk of bias in algorithms trained on incomplete or biased data. This is briefly mentioned in a quote by Charlie Paterson but isn't explored in depth.
False Dichotomy
The article presents a somewhat simplistic eitheor framing by contrasting AI-powered digital twins with traditional human clinical trials, implying that one is a clear replacement for the other. The complexities and nuances of integrating both approaches, and the limitations of relying solely on either method, are not fully explored.
Gender Bias
The article features quotes from both male and female experts (Sheena Macpherson and Matt Truppo), but does not explicitly address gender bias in medical device testing or in the data used to train the AI models. The lack of explicit analysis in this regard is a potential omission.
Sustainable Development Goals
The development and use of AI-powered digital twins for testing medical devices has the potential to significantly improve the safety and efficacy of these devices, leading to better health outcomes and reduced mortality. The technology allows for more thorough testing across diverse populations, addressing historical biases in clinical trials. This directly contributes to SDG 3 (Good Health and Well-being) by reducing medical device-related deaths and injuries and improving the development of safer, more inclusive medical technologies.