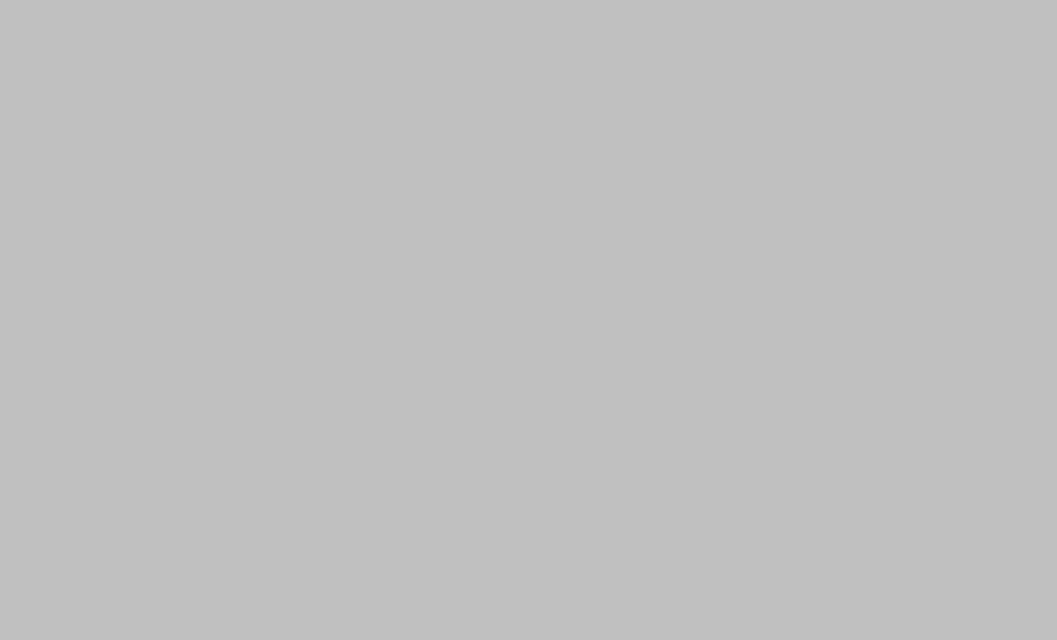
welt.de
AI Improves Weather Forecasting Accuracy
DeepMind's AI model, GenCast, outperforms traditional methods in medium-range weather forecasting by achieving 97% accuracy across 1320 atmospheric variables, generating 15-day global forecasts in eight minutes, but a German Weather Service expert highlights limitations in replicating complex weather patterns.
- How does DeepMind's GenCast improve upon existing medium-range weather forecasting methods, and what are the immediate implications for weather prediction accuracy?
- DeepMind's new AI model, GenCast, outperforms traditional methods in medium-range weather forecasting, achieving 97% accuracy across 1320 atmospheric variables. It generates global 15-day forecasts in eight minutes, surpassing the European Centre for Medium-Range Weather Forecasts (EZMW) in accuracy. However, a German Weather Service (DWD) expert notes limitations in replicating complex weather patterns.
- What are the limitations of AI-based weather forecasting models compared to traditional physics-based models, and how does the DWD plan to integrate both approaches?
- GenCast leverages 40 years of weather data (1979-2018) and employs a novel approach of running 50 calculations per forecast to improve prediction reliability. This surpasses traditional models, especially in predicting extreme weather, tropical cyclone paths, and wind speeds. The DWD, while acknowledging GenCast's potential, emphasizes its role as a supplement rather than a replacement for existing physical models.
- What are the long-term implications of integrating AI into weather forecasting, and what challenges need to be addressed to ensure the reliability and accuracy of these combined systems?
- The integration of AI, like GenCast, into weather forecasting signifies a shift towards faster and potentially more accurate predictions. However, challenges remain in fully replicating the intricate processes of weather systems using AI alone. Future development will likely focus on combining the strengths of both AI and physics-based models for comprehensive and reliable forecasts, potentially leading to enhanced early warning systems for extreme weather events.
Cognitive Concepts
Framing Bias
The headline and initial paragraphs emphasize the positive aspects of the AI model's capabilities, highlighting its speed and accuracy improvements. While the expert's skepticism is included, it's presented later, potentially downplaying the limitations of the AI model in the reader's initial understanding.
Language Bias
The article uses language that leans slightly towards promoting the AI model. Words like "Genauere" (more precise), "schneller" (faster), and "verlässlichere" (more reliable) are used to describe the AI model, while the expert's skepticism is presented more cautiously. Neutral alternatives could include phrasing emphasizing both strengths and limitations more equally.
Bias by Omission
The article focuses heavily on the DeepMind AI model's capabilities and its comparison to traditional models, but omits discussion of the computational resources required to train and run GenCast. This omission could lead readers to underestimate the infrastructure demands and associated costs of implementing such technology on a large scale.
False Dichotomy
The article presents a false dichotomy by framing the AI model and traditional models as competing alternatives, rather than exploring their potential for collaborative use. The expert's quote mentioning AI as a complement, not a replacement, counters this but the main narrative leans towards a competition.
Sustainable Development Goals
The development of more accurate and faster weather forecasting models, such as DeepMind's GenCast, can significantly contribute to climate action. Improved predictions of extreme weather events, including tropical storms and wind speeds, enable better preparation and mitigation strategies, reducing the impact of climate-related disasters on communities and infrastructure. More efficient forecasting also allows for better resource allocation and preparedness.