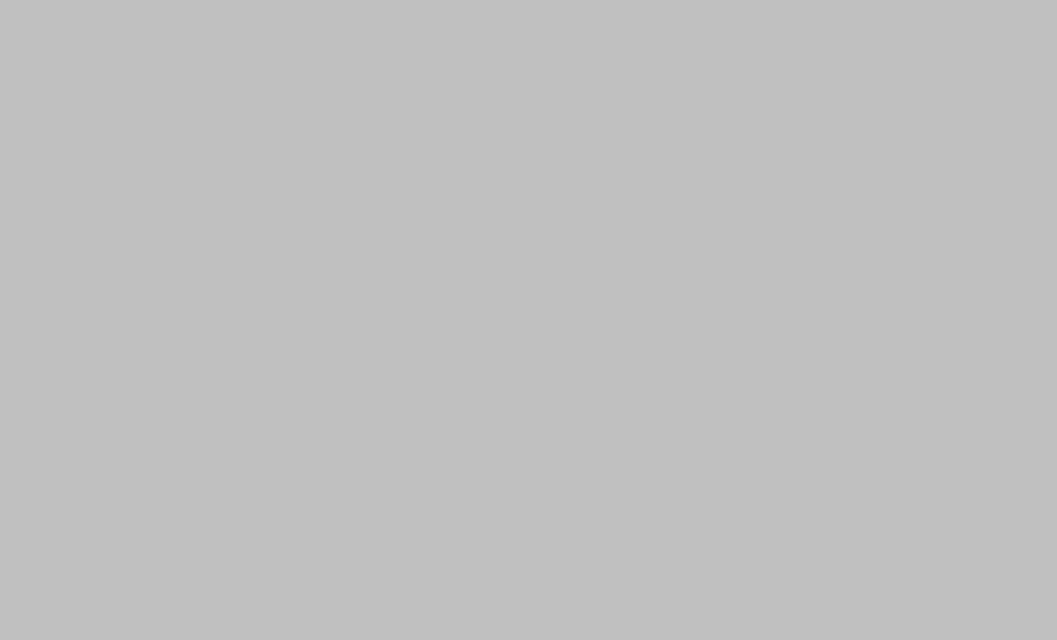
faz.net
AI Models Show Left-Leaning Bias in German Election Test
Studies reveal AI language models, including ChatGPT and Elon Musk's Grok, exhibit a left-leaning bias when tested with Germany's Wahl-O-Mat, although the methodology and interpretation remain controversial; newer models show a potential rightward shift.
- What are the long-term implications of AI's evolving political leanings, and how might this impact future elections and political decision-making?
- Future AI models may shift politically depending on user feedback and societal changes. A recent study from Peking University detected a significant rightward shift in newer ChatGPT versions, suggesting AI's political leaning is dynamic and reflects evolving public opinion.
- What specific evidence demonstrates a political bias in current AI language models, and what are the immediate implications for political discourse?
- Studies using Germany's Wahl-O-Mat questionnaire found that AI models, including Elon Musk's Grok, showed a preference for Green or left-leaning positions. This aligns with other research indicating a leftward bias in AI models like ChatGPT.
- How do the methodologies used to assess political bias in AI models impact the results, and what alternative approaches could provide more objective insights?
- The bias is attributed to either overrepresentation of left-leaning viewpoints in training data or distortions during the fine-tuning process. However, the methodology is questionable, as the 'objective' comparison values used (like the Political Compass test) are themselves biased and politically charged.
Cognitive Concepts
Framing Bias
The article frames the debate around the alleged left-leaning bias of AI models, giving prominence to studies supporting this claim. While acknowledging opposing views, the overall narrative emphasizes the prevalence and implications of this perceived bias. The headline and introduction both emphasize the left-leaning tendencies, influencing the reader's initial perception of the article's findings and potentially shaping their interpretation of the subsequent information.
Language Bias
While largely neutral in tone, the article employs language that can subtly influence the reader. For example, phrases like "links is, who does not beat children" (translated from German) present a simplified and potentially loaded view of political positions. The use of the term "links" (left) repeatedly, while technically correct, could imply a negative connotation for some readers. Additionally, describing AI as "ticking left" (translated from German) employs anthropomorphism and subjective language.
Bias by Omission
The analysis omits discussion of the methodologies used in various studies assessing AI political bias, limiting a comprehensive understanding of the reliability and validity of the findings. It also omits counterarguments or alternative interpretations of the observed biases, which could stem from factors beyond inherent political leanings. For example, the overrepresentation of certain viewpoints in training data is mentioned, but not fully explored. The limitations of using the 'Political Compass' test are noted, but a deeper analysis of alternative comparative metrics is absent.
False Dichotomy
The article presents a false dichotomy by framing the debate as solely between a 'left-leaning' bias and a 'right-leaning' bias in AI models, neglecting the possibility of other factors influencing the observed outcomes, such as biases in data collection, algorithmic design, or user interaction. The nuanced reasons for observed biases are oversimplified and presented as either inherent political leaning or the influence of training data.
Sustainable Development Goals
The article discusses biases in AI language models, showing a tendency to lean towards left-leaning political positions. This could exacerbate existing inequalities by potentially marginalizing or misrepresenting certain viewpoints in public discourse. The lack of diversity in training data and potential biases in evaluation metrics contribute to this issue, hindering equal representation of diverse perspectives.