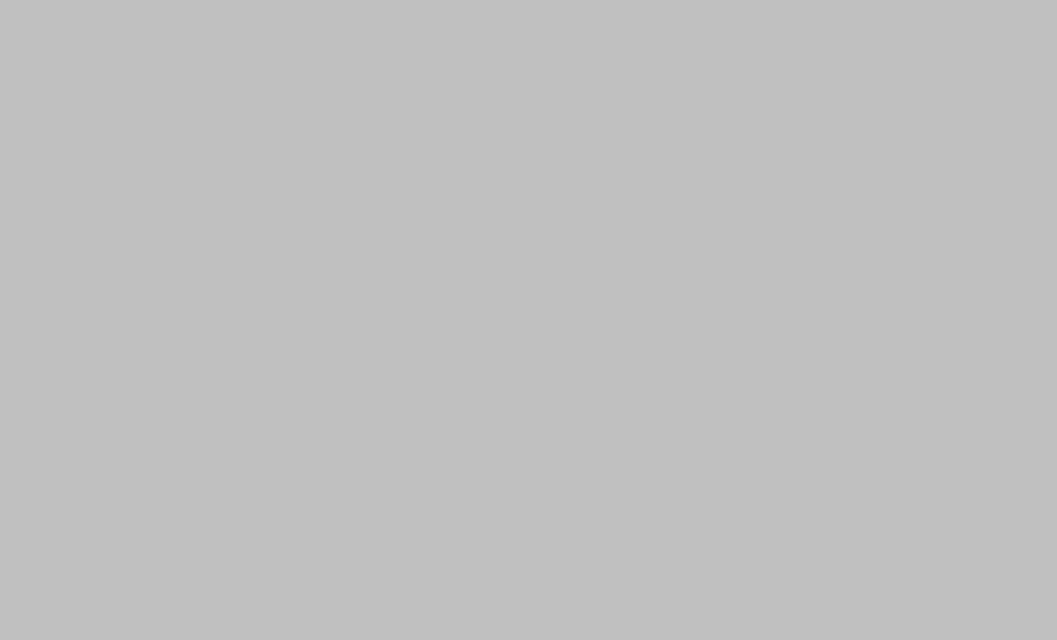
nrc.nl
AI-Powered Model Precisely Maps Tropical Deforestation
A new self-learning computer model uses satellite imagery to precisely measure various types of deforestation in tropical forests, differentiating between natural and human-caused losses for improved monitoring and intervention.
- What is the primary impact of this new computer model on monitoring and mitigating deforestation in tropical regions?
- A new self-learning computer model developed at Wageningen University allows for the precise measurement of deforestation in tropical forests using various satellite images, including radar and infrared, overcoming cloud cover limitations during the rainy season. This model distinguishes between different types of deforestation, such as large-scale logging, agricultural conversion, and mining, by analyzing reflection differences and contextual factors.
- How does the model distinguish between different causes of deforestation, and what are the implications for environmental impact assessment?
- The model's ability to differentiate deforestation types is crucial for accurately assessing the environmental consequences, such as CO2 emissions, and for targeted interventions. The increasing resolution of satellite imagery, now providing weekly updates with 10x10 meter pixels, significantly enhances the model's accuracy and timeliness. This contrasts with previous models that often lagged behind actual events.
- What are the potential future applications of this technology beyond environmental monitoring, and what challenges remain in ensuring its accuracy and effectiveness?
- This near real-time monitoring system, trained using artificial intelligence, enables timely intervention against illegal or unsustainable activities. Future applications include monitoring companies for compliance with regulations, assisting in the implementation of the European deforestation law, and providing data for sustainable forest management practices. The model's accuracy is continuously assessed and refined, with field work planned to validate its findings.
Cognitive Concepts
Framing Bias
The article frames the issue through the lens of technological innovation, emphasizing the accuracy and efficiency of the new model. This framing could inadvertently minimize the urgency and severity of the environmental crisis and shift the focus away from policy solutions and direct action to combat deforestation. The headline, if there was one, would likely highlight the technological achievement.
Language Bias
The language used is largely neutral and objective, employing scientific terminology and focusing on data and methods. However, phrases such as "live naar de bosvernietiging aan het kijken" (live watching the forest destruction) might evoke strong emotional responses, though this is likely unintentional given the overall scientific focus.
Bias by Omission
The article focuses primarily on the technological advancements in deforestation monitoring, potentially omitting discussions on socio-economic factors driving deforestation, such as poverty, population growth, or land tenure issues. While acknowledging limitations in scope, a broader contextualization of the drivers of deforestation would enhance the article's understanding.
False Dichotomy
The article doesn't explicitly present a false dichotomy, but it might implicitly frame the issue as a technological solution to a complex problem. The focus on advanced monitoring suggests technological solutions are sufficient to address deforestation, while ignoring underlying social and political issues that may need separate solutions.
Sustainable Development Goals
The research develops a self-learning computer model to monitor deforestation in tropical forests, enabling more accurate measurement of forest loss and timely intervention against illegal or unsustainable activities. This directly contributes to SDG 15, Life on Land, by improving forest management and reducing deforestation rates.