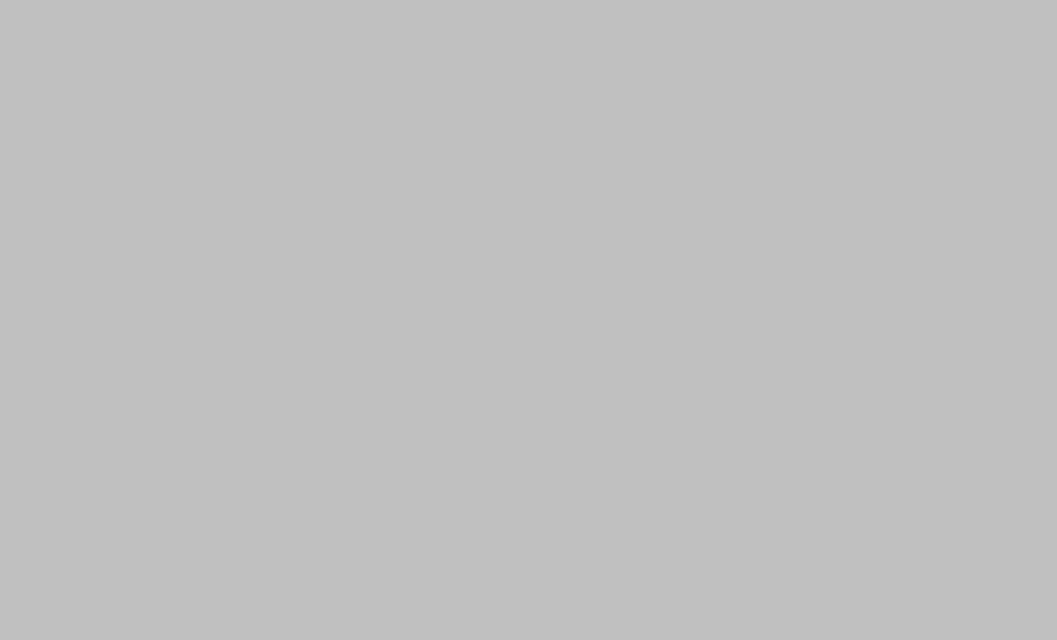
forbes.com
AI-Powered Predictive Analytics: Transforming Business Decisions and Operations
Predictive analytics, enhanced by AI, uses data and algorithms to forecast future outcomes, improving business decisions and operational efficiency across various sectors, as demonstrated by companies like Walmart and UPS, although ethical considerations remain.
- What are the most significant impacts of AI-powered predictive analytics on business decision-making and operational efficiency?
- Predictive analytics uses data and algorithms to forecast future outcomes, going beyond basic forecasting by using statistical modeling and machine learning. This allows for applications in various fields, such as predicting allergic reactions (as seen with AbbieSense) and optimizing delivery routes (as done by UPS).
- How do companies like Walmart and UPS leverage predictive analytics to optimize their operations and gain a competitive advantage?
- The core value of predictive analytics lies in its ability to identify patterns and make data-driven predictions, improving decision-making across various sectors. Businesses use it for proactive improvements in efficiency, customer satisfaction, and cost reduction, as exemplified by Walmart's stock allocation optimization. Early problem detection and risk mitigation are additional benefits, as highlighted by the application in software quality assurance.
- What are the potential ethical considerations and challenges associated with the increasing use of predictive analytics, particularly concerning data privacy and algorithmic bias?
- AI has significantly enhanced predictive analytics by automating processes like feature selection and model training, enabling real-time learning and continuous improvement. This is evident in Amazon's recommendation engine, which contributes significantly to its revenue, and in UPS's route optimization system, leading to substantial cost savings. The future will see even broader integration of predictive analytics across industries.
Cognitive Concepts
Framing Bias
The article frames predictive analytics overwhelmingly positively, highlighting success stories and emphasizing its transformative potential. The headline and introduction set a strongly optimistic tone, potentially influencing reader perception towards unquestioning acceptance of the technology. Negative aspects are largely absent from the prominent sections.
Language Bias
The language used is largely positive and enthusiastic, employing terms like "powerful game-changer," "exponentially amplified," and "unprecedented opportunities." While this generates excitement, it lacks neutrality. More neutral alternatives could be used to maintain objectivity.
Bias by Omission
The article focuses heavily on the positive aspects of predictive analytics and its applications, potentially omitting potential downsides or limitations. For instance, ethical concerns surrounding data privacy and algorithmic bias are not discussed, nor are the potential job displacement effects of automation. While brevity may be a factor, these omissions limit the reader's ability to form a fully informed opinion.
False Dichotomy
The article presents a somewhat simplistic view of predictive analytics as a universally beneficial tool, neglecting potential drawbacks or situations where it might be less effective. It doesn't acknowledge situations where predictive models might fail or lead to inaccurate predictions.
Sustainable Development Goals
Predictive analytics can help reduce inequalities by enabling proactive interventions and improving outcomes in areas like healthcare and education. For example, identifying high-risk patients allows for early intervention, improving healthcare access and outcomes for disadvantaged populations. Similarly, optimizing resource allocation in education can help ensure equitable access to quality education.