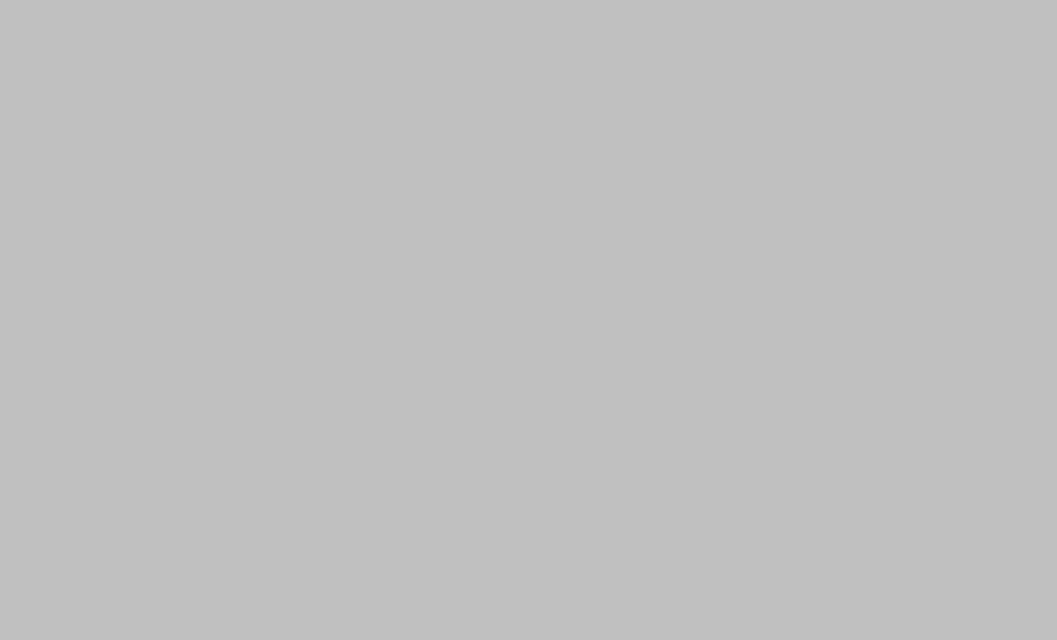
english.elpais.com
AI Revolutionizes Protein Structure Prediction, Opening New Scientific Horizons
Google DeepMind's AI tool, AlphaFold2, has determined the 3D structure of 200 million proteins, revolutionizing fields like drug discovery and biological research, with future applications in genomics, new materials, and nuclear fusion.
- What are the limitations of AI in scientific research, and how can these be addressed?
- AI's application extends beyond protein structure prediction; it's transforming genomics by analyzing DNA semantics and impacting materials science and nuclear fusion research. The ability to predict protein structures facilitates advancements in medicine and biotechnology.
- What are the long-term implications of using AI-generated synthetic data in scientific modeling?
- Future applications of AI in genomics could enable precise gene editing for disease treatment and prevention. Furthermore, AI's role in developing new materials and optimizing nuclear fusion processes will likely accelerate technological advancements in energy and other industries. The limitations of current models are crucial to understand, especially in areas involving human subjectivity.
- How is artificial intelligence revolutionizing scientific research and what are its immediate implications?
- Google DeepMind's AlphaFold2, using AI, has mapped the 3D structure of 200 million proteins, a breakthrough impacting various scientific fields. This has significant implications for drug discovery and understanding biological processes.
Cognitive Concepts
Framing Bias
The overwhelmingly positive framing of AI's impact on science, particularly highlighting Google DeepMind's achievements, creates a potential bias. The use of quotes from Google DeepMind researchers and the focus on their projects shapes the narrative towards a highly optimistic viewpoint. While their contributions are significant, a more balanced perspective is needed.
Language Bias
The language used is largely neutral and objective, with the exception of phrases like "amazing" and "opened up new horizons," which reveal a positive bias towards AI. While these terms are common in promotional materials, more neutral vocabulary would enhance the article's objectivity. Suggesting neutral alternatives would increase the objectivity of the article.
Bias by Omission
The article focuses heavily on Google DeepMind's successes with AI in science, potentially omitting other significant contributions from other institutions or researchers. While acknowledging limitations of scope is important, a broader perspective on the field's advancements would enrich the piece. The article also doesn't discuss potential downsides or ethical concerns of using AI in scientific research.
False Dichotomy
The article presents a somewhat simplistic view of AI's applicability to science, suggesting that any problem formulated as a reasoning or pattern recognition problem can benefit. This ignores complexities and potential limitations of AI in specific scientific fields, particularly those involving subjective human elements or insufficient data.
Gender Bias
The article does not exhibit overt gender bias. The prominent mention of Pushmeet Kohli does not overshadow any other scientist's contributions. However, it would be beneficial to include more diverse voices and perspectives within the piece for better representation.
Sustainable Development Goals
The development of AlphaFold2, an AI tool that predicts protein structures, is revolutionizing biological research. Understanding protein structures is fundamental to understanding diseases and developing new treatments. The ability to predict the 3D shape of 200 million proteins vastly accelerates drug discovery and disease research, leading to improved health outcomes. Further work on genomics aims to understand the semantics of DNA, predicting the effects of mutations and paving the way for more effective gene therapies.