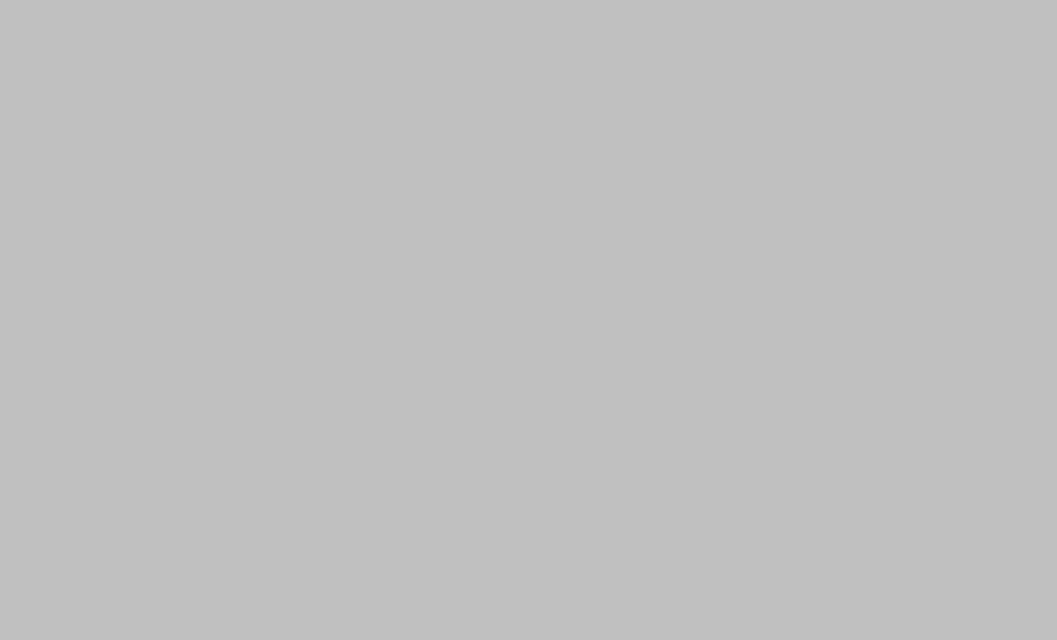
bbc.com
AI Shows Promise in Preventing Diabetes-Related Sight Loss
AI-powered tools are being developed to detect diabetic retinopathy earlier and more efficiently by analyzing fundus images, potentially preventing vision loss, though cost-effectiveness varies and equitable access is a key challenge.
- What are the economic and logistical barriers to implementing AI-powered diabetic retinopathy screening globally, and how can these be overcome?
- The cost-effectiveness of AI in diabetic retinopathy screening varies depending on existing infrastructure and healthcare systems. While studies show promising results in high-income countries, broader implementation requires addressing challenges like data quality and ensuring equitable access in low- and middle-income countries. Hybrid models, combining AI with human expertise, offer a balance between cost and accuracy.
- How can AI improve the efficiency and accessibility of diabetic retinopathy screening, and what are the potential impacts on vision loss prevention?
- AI-powered diagnostic tools are being developed to improve the detection of diabetic retinopathy, a diabetes-related eye disease that can lead to vision loss. These tools analyze fundus images, speeding up the process and potentially reducing costs compared to manual interpretation. Early detection is crucial, as it allows for timely intervention and prevents vision deterioration.
- What are the long-term implications of AI-driven diabetic retinopathy screening on healthcare systems, particularly regarding cost-effectiveness, equity, and the potential for broader applications in ophthalmology?
- Future applications of AI in diabetic retinopathy screening could lead to timely interventions, reducing cases of vision loss and improving the quality of life for patients with diabetes. However, ensuring widespread access and addressing health equity gaps, particularly in low-resource settings, is crucial for maximizing the technology's potential benefits. Further research is needed to improve AI's accuracy in detecting other eye diseases.
Cognitive Concepts
Framing Bias
The narrative frames AI as a largely positive and promising solution, highlighting success stories and minimizing potential drawbacks. The headline itself implies a positive outcome. The use of strong quotes from satisfied patients and experts contributes to this positive framing. While challenges are mentioned, the overall tone emphasizes the potential benefits, potentially creating an overly optimistic view of the technology's immediate impact.
Language Bias
The language used is generally neutral, though some words like "grabbed it with both hands" in the final quote inject a slightly emotional tone. However, this is mostly within the context of a personal anecdote and does not significantly skew the overall presentation. The use of specific statistics and expert quotes also aids in maintaining objectivity.
Bias by Omission
The article focuses heavily on the experiences of Terry Quinn and the successes of AI in developed countries, potentially omitting challenges and limitations in low-resource settings. While the concerns of cost and accessibility are mentioned, a more in-depth exploration of these challenges in various global contexts would provide a more balanced perspective. The article also doesn't discuss potential negative societal impacts of increased automation in healthcare, such as job displacement for human graders.
False Dichotomy
The article presents a somewhat simplified view of the AI solution, contrasting it with the current manual process without fully exploring alternative solutions or intermediate approaches. The focus on AI as either a complete replacement or a simple support tool overlooks the potential for other innovations or hybrid models.
Sustainable Development Goals
The article discusses the use of AI to improve the detection and prevention of diabetic retinopathy, a leading cause of vision loss. Early detection through AI-powered screening can enable timely intervention, preventing vision impairment and improving the quality of life for individuals with diabetes. This directly contributes to SDG 3, ensuring healthy lives and promoting well-being for all at all ages.