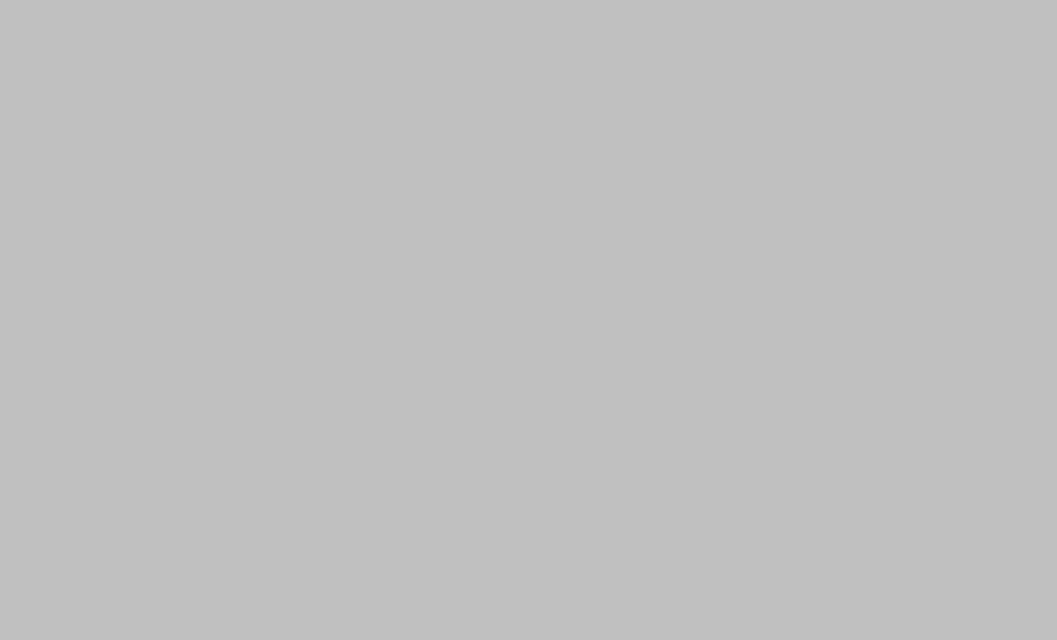
forbes.com
AI Stock Sell-Off: January 2025 Update
The January 27, 2025, sell-off in AI-related stocks, triggered by the release of a cheaper large language model, saw a median 50% drop, but recovery patterns varied depending on market conditions, with some stocks quickly rebounding, others lagging, and the long-term outcome remaining uncertain.
- What were the immediate consequences of the January 2025 AI stock sell-off, and how did it compare to previous market events?
- In January 2025, numerous AI-related stocks experienced significant single-day drops following 52-week highs, triggered by the announcement of a cheaper large language model. Analysis of similar past events revealed varied recovery patterns depending on the overall market conditions.
- How did overall market conditions influence the recovery trajectories of stocks following significant drops in the past four years?
- Comparing stock performance after sharp drops in 2021-2022 and 2023-2024, the study found that market conditions significantly influenced recovery times and ultimate returns. Stocks in 2023-2024 generally outperformed the S&P 500 post-drop, while those in 2021-2022 generally did not.
- What are the key indicators for predicting which AI stocks will recover to their previous highs after the January 2025 sell-off, and what factors will likely shape the long-term outcome?
- The January 2025 AI stock sell-off initially resembled the 2021-2022 period in terms of percentage losses but mirrored 2023-2024 in recovery speed. However, the recovery's strength and the number of stocks returning to highs remain uncertain, highlighting the importance of individual stock analysis and base formation.
Cognitive Concepts
Framing Bias
The article frames the analysis around past stock performance to predict future trends. While using data-driven analysis, the emphasis on historical patterns might lead readers to overestimate the predictability of future stock behavior. The headline 'Update from January Sell-Off in Artificial Intelligence' subtly suggests that the analysis will provide definitive conclusions about future market trends rather than highlighting the inherent uncertainties of the stock market.
Language Bias
The language used is mostly neutral, with some instances of potentially loaded terms. For example, phrases like 'rapid recovery' and 'no recovery' could be seen as subjective and potentially emotional. More neutral terms like 'faster than average recovery', 'slower than average recovery', and 'no return to previous high' would better convey the data without bias.
Bias by Omission
The analysis focuses heavily on specific stock performance data, potentially omitting broader economic factors or alternative interpretations of the AI market trends that contributed to the January sell-off. While acknowledging market conditions, a more comprehensive analysis might include discussion of other contributing factors beyond the DeepSeek announcement, such as regulatory concerns or investor sentiment shifts.
False Dichotomy
The analysis presents a somewhat false dichotomy by categorizing stock performance into only four groups (Rapid Recovery, More Typical Recovery, Below Typical Recovery, No Recovery). The reality is likely more nuanced, with a wider range of recovery patterns.
Sustainable Development Goals
The analysis of stock market trends and their recovery patterns can indirectly contribute to reduced inequality by providing insights for investors, potentially leading to more equitable distribution of wealth if the information helps investors make sound decisions that benefit a broader range of people. The data analysis focuses on market behavior and does not directly address social inequality, but the information can inform investment strategies that may indirectly impact wealth distribution.