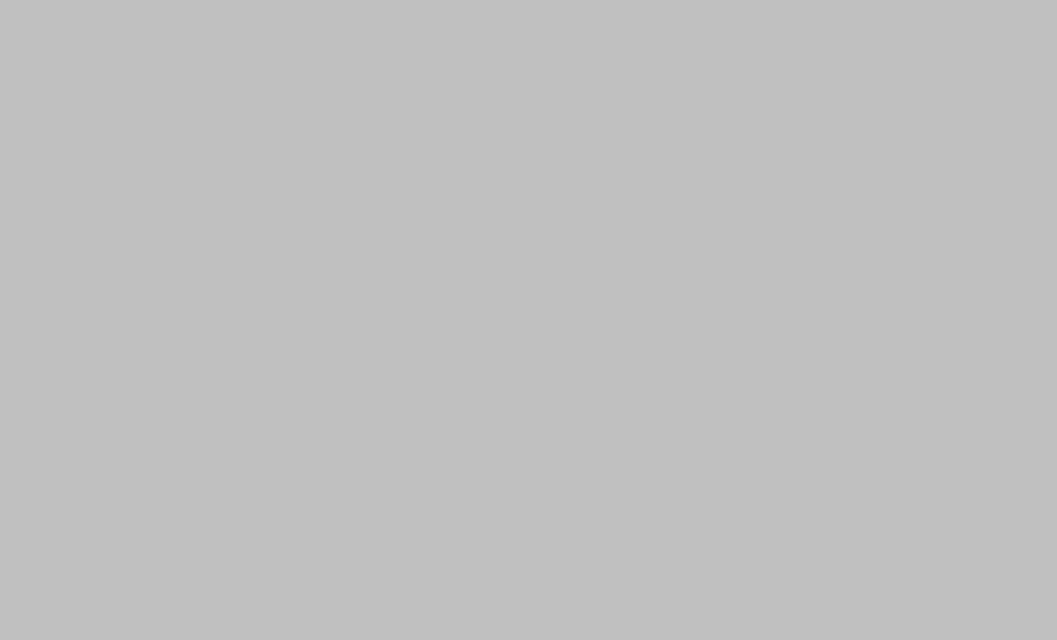
forbes.com
AI Success: Structure Trumps Scale
Goldman Sachs forecasts over \$1 trillion in AI investment, but smaller firms like smallest.ai demonstrate that efficient design and structured systems, rather than scale, are key to AI success.
- How do the recent successes of smaller AI companies challenge the conventional wisdom that larger models yield better AI outcomes?
- Smaller firms like smallest.ai surpass larger competitors in text-to-speech metrics using fewer resources, highlighting efficiency over scale in AI.
- What are the long-term systemic impacts of democratizing AI access and development, and what infrastructure is needed to ensure meaningful progress?
- Future AI leadership requires a balance of experimentation and structured operational models, enabling impactful AI integration into workflows and strategic decision-making.
- What is the primary factor determining success in the rapidly expanding AI market, and what are its immediate implications for businesses of all sizes?
- Goldman Sachs projects over \$1 trillion in AI spending in the coming years, yet success hinges not on scale but on structured systems and integrated platforms.
Cognitive Concepts
Framing Bias
The article frames the narrative to emphasize the success of smallest.ai as a disruptive example challenging the prevailing belief that bigger AI models are better. The positive portrayal and inclusion of direct quotes from the company's founders contribute to this framing. The headline and subheadings reinforce this focus, potentially overshadowing other important aspects of AI development.
Language Bias
The article uses positive language to describe smallest.ai's achievements ("Impressive to say the least"), while terms like "reckless scale" are used to negatively characterize large-scale AI initiatives. More neutral language, such as replacing "reckless scale" with "large-scale resource allocation" could enhance objectivity.
Bias by Omission
The article focuses heavily on the success of smallest.ai, potentially omitting other companies or approaches to AI development that emphasize efficiency over scale. While acknowledging the limitations of space, a broader discussion of diverse AI development strategies would provide more comprehensive context.
False Dichotomy
The article presents a false dichotomy between 'bigger is better' and 'smaller is more efficient'. While acknowledging the importance of scale in some contexts, it oversimplifies the complexity of AI development by framing the choice as an eitheor proposition, neglecting approaches that find balance between scale and efficiency.
Gender Bias
The article doesn't exhibit overt gender bias. The named individuals mentioned are male, but this is not presented in a way that suggests gender is a factor in their success or the broader AI field. More diverse representation in examples would strengthen the analysis.
Sustainable Development Goals
The article highlights how advancements in AI are becoming more accessible to smaller companies and individuals, thus reducing the historical dominance of large corporations and research institutions in the field. This democratization of AI technology can lead to a more equitable distribution of opportunities and resources, fostering innovation and economic growth across various sectors and demographics.