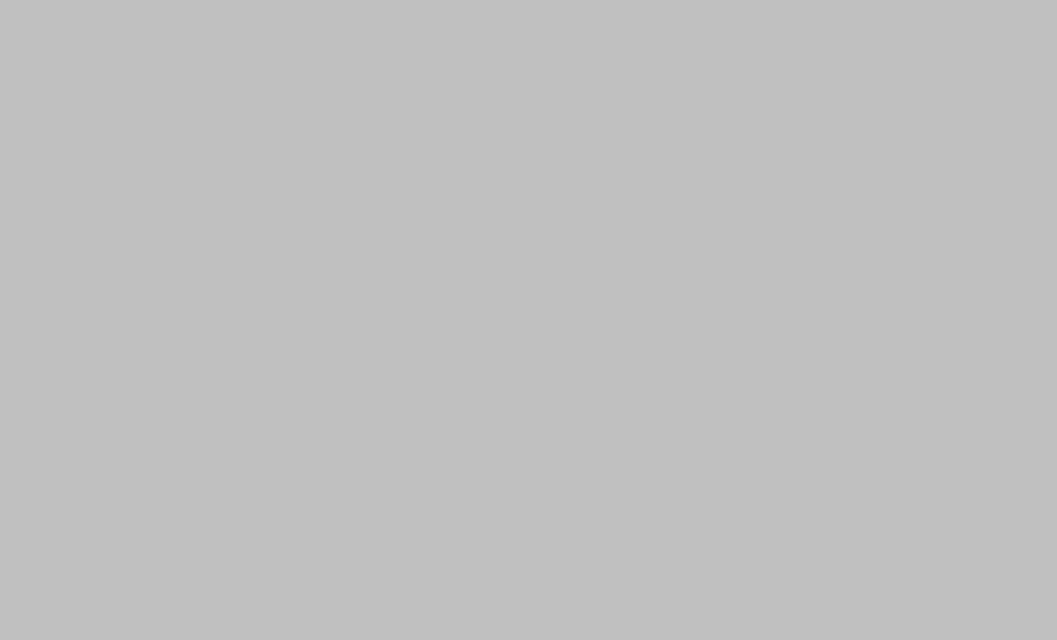
jpost.com
AI System scNET Improves Understanding of Cellular Drug Response
Tel Aviv University researchers developed scNET, an AI system that analyzes single-cell gene activity and interactions to reveal subtle changes in how cells respond to drug treatments, especially in cancer, as published in Nature Methods.
- How does scNET's dual-layered approach enhance the analysis of cellular behavior compared to traditional methods?
- scNET overlays gene expression data with a gene interaction map, identifying hidden activity patterns. This network-based approach allows researchers to observe how immune cells, such as T cells, increase their activity in response to treatment, enhancing the understanding of cellular responses to therapies. The improved resolution enables a more comprehensive analysis of complex biological processes, particularly in cancer treatment.
- What is the primary impact of scNET on cancer research, and how does it improve the understanding of treatment response?
- Tel Aviv University researchers developed scNET, an AI system analyzing single-cell gene activity and gene interactions to understand cellular responses to drug treatments. This reveals subtle changes in cell behavior, especially in complex environments like tumors, improving the detection of rare cell populations' responses to therapies like immunotherapy or chemotherapy. The study, published in Nature Methods, demonstrated scNET's ability to detect previously undetectable increases in T cell cytotoxicity in treated tumor environments.
- What are the potential future implications of scNET for personalized medicine and the development of new therapies beyond cancer treatment?
- scNET's application extends beyond cancer research; it could improve the development of new treatments, deepen our understanding of immune function, and advance personalized medicine. The ability to analyze individual cell responses to therapy may guide clinical decisions, offering tailored treatment approaches based on specific cellular reactions. This innovative tool could significantly impact various disease investigations and clinical practices.
Cognitive Concepts
Framing Bias
The framing is overwhelmingly positive. The headline and introduction highlight the significant potential of scNET. The quotes from researchers emphasize the groundbreaking nature of the technology and its ability to solve previously unsolvable problems. This positive framing, while not inherently biased, could lead readers to overestimate the immediate impact and applicability of the technology.
Language Bias
The language used is largely neutral and objective, using descriptive terms like "innovative," "subtle but critical," and "powerful demonstration." However, phrases like "unprecedented resolution" and "groundbreaking" are somewhat emotive and suggestive of a strongly positive appraisal. The use of terms like "noisy" data to describe a challenge, then later highlighting scNET's ability to identify patterns "previously hidden in the noise", could be construed as slightly loaded.
Bias by Omission
The article focuses primarily on the positive aspects of the new AI-based method, scNET, and its potential applications. While acknowledging its limitations in detecting rare cell populations, it doesn't delve into potential drawbacks or limitations of the method itself, such as computational cost, data dependency, or potential biases in the underlying gene interaction network. Further, there is no mention of alternative methods or approaches to studying cellular responses to drug treatments.
Sustainable Development Goals
The development of scNET, a new AI-based method, significantly enhances our understanding of how cells respond to drug treatments, particularly in complex environments like cancerous tumors. This leads to improved diagnosis, treatment efficacy, and potentially cures, directly contributing to better health and well-being. The method's ability to identify subtle changes in cell behavior in response to immunotherapy or chemotherapy is a major advancement in cancer treatment.