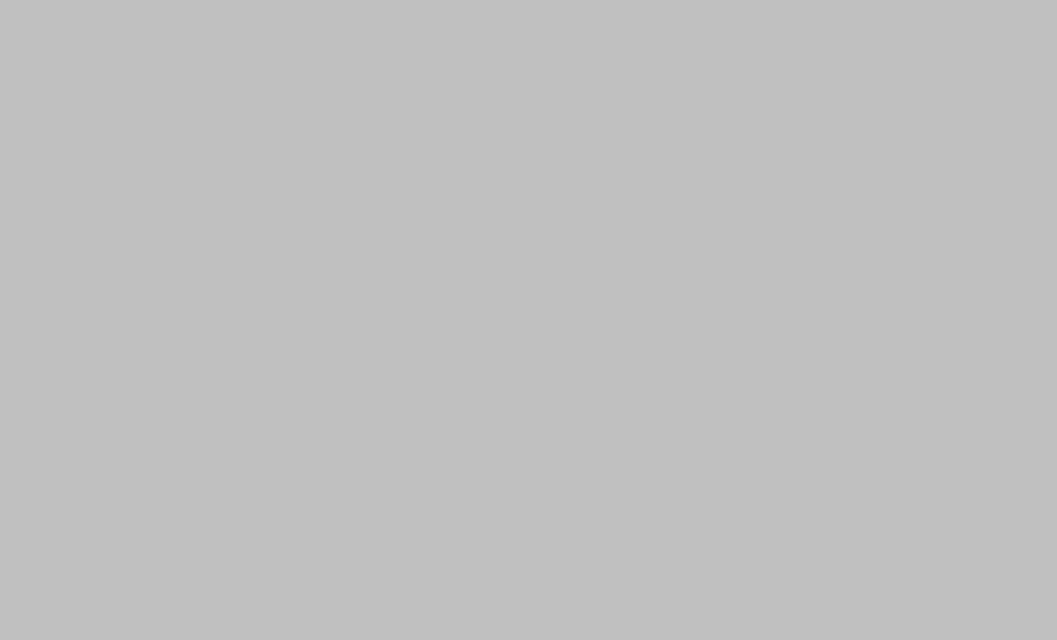
us.cnn.com
AI Weather Model GenCast Outperforms Traditional Forecasts
Google's DeepMind's AI model, GenCast, outperforms traditional models in 15-day weather forecasting accuracy by analyzing historical data, achieving 97% accuracy across 1,300 weather variable combinations in 2019, showing a 10-30% improvement in the three-to-five-day range compared to the European Centre for Medium-Range Weather Forecasts's model.
- How does GenCast's accuracy compare to existing models, and what are the key improvements leading to this enhanced performance?
- Google's DeepMind developed GenCast, an AI weather forecasting model significantly outperforming traditional models in accuracy for up to 15 days. This surpasses previous AI models by providing a range of possible future weather scenarios and their likelihoods, leading to more reliable long-range forecasts. The model achieved 97% accuracy across 1,300 weather variable combinations.
- What are the limitations of GenCast, and what challenges remain in using AI for weather forecasting, particularly concerning extreme weather events?
- GenCast's improved accuracy stems from analyzing historical weather data to predict future atmospheric behavior, unlike traditional models relying on real-time observations and complex physics equations. This approach reduces errors from real-time data, enabling faster simulations and improved forecasts with less computational power. The model's ability to run numerous simulations simultaneously enhances confidence in longer-range predictions.
- What are the future implications of this technology for weather forecasting and public safety, considering both advancements and remaining limitations?
- GenCast's success marks an inflection point in AI weather forecasting. While challenges remain, especially in predicting extreme weather events in a changing climate and tropical cyclone intensity, ongoing improvements and hybrid models combining AI with physics promise further advancements. The integration of GenCast into operational forecasting could significantly enhance accuracy and lead to better public safety measures.
Cognitive Concepts
Framing Bias
The framing is generally positive towards AI weather forecasting, highlighting its advancements and potential. The headline and introduction immediately establish this positive tone, focusing on the accuracy improvements. While limitations are mentioned, they are presented as challenges to be overcome rather than significant drawbacks.
Language Bias
The language used is largely neutral, although phrases like "impressive result," "big step," and "game changer" reveal a positive bias towards GenCast. However, these are largely within the context of reporting expert opinions.
Bias by Omission
The article focuses heavily on the successes of the GenCast model and mentions limitations, but doesn't deeply explore potential societal impacts of more accurate long-range forecasts (e.g., economic effects on agriculture or tourism). It also doesn't discuss the ethical implications of relying on AI for life-critical predictions.
False Dichotomy
The article presents a somewhat simplistic 'AI vs. traditional models' dichotomy, overlooking the potential for hybrid approaches combining both methods. While acknowledging limitations, it focuses primarily on AI's advantages without fully exploring the benefits of traditional modelling.
Sustainable Development Goals
The development of AI-powered weather forecasting models, such as Google's GenCast, has the potential to significantly improve the accuracy and timeliness of weather predictions. More accurate forecasts can lead to better preparedness for extreme weather events, reducing their impact and improving climate resilience. The model's ability to predict extreme weather events, even those outside its training data, suggests a positive impact on climate change adaptation.