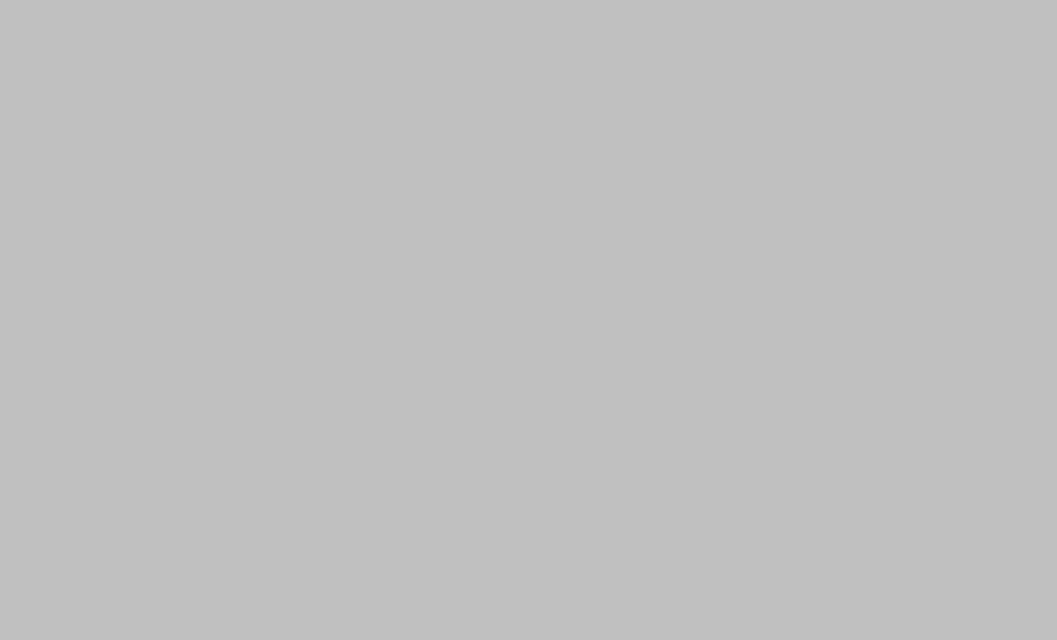
forbes.com
AI's Shift to "xLM" Models Demands Real-Time Data Engineering
The AI industry is transitioning from large language models (LLMs) to diverse "xLM" models, requiring a shift from batch data processing to real-time "live AI" data pipelines to meet demands for speed and accuracy, impacting data engineering roles and processes.
- What is the evolving role of data engineers in the context of live AI and the rapid development of new AI models?
- The future of data engineering in AI involves a shift from data protection to data stewardship, where data engineers play a crucial role in strategic decision-making and pipeline innovation. By adopting automated and intelligent data management frameworks, teams can reduce manual tasks, enabling faster experimentation and more efficient AI-driven processes. This proactive approach ensures that data pipelines can adapt to the rapid pace of change in the evolving AI model landscape. This will require a focus on robust real-time data infrastructure and the adoption of modern data engineering practices.
- How does the increasing demand for real-time AI applications impact data engineering practices and resource requirements?
- The transition to xLM models creates challenges for data engineering teams relying on static batch data uploads. Frequent batch uploads, necessary for maintaining data accuracy in these dynamic systems, demand specialized expertise, leading to cost and resource constraints. This is compounded by the growing demand for applications requiring real-time accuracy. The solution is transitioning to "live AI", using continuous stream processing to enhance model accuracy and reduce the load on data engineering teams.
- What are the key challenges and opportunities presented by the shift from LLMs to the more diverse "xLM" models in the AI industry?
- The AI landscape is rapidly shifting from large language models (LLMs) to a more diverse range of "xLM" models, encompassing various sizes, domains, and specializations. This evolution necessitates a significant change in data infrastructure, demanding architectures that handle diverse data types, streaming data, and real-time updates, all while adhering to strict governance and security protocols. The increasing prevalence of real-time applications further intensifies the need for agile, adaptable data pipelines.
Cognitive Concepts
Framing Bias
The article frames the evolution of AI models and data infrastructure as primarily a positive development, emphasizing the opportunities presented by 'live AI' and faster data processing. While acknowledging challenges, the narrative leans towards a predominantly optimistic outlook, potentially downplaying potential risks or negative consequences.
Language Bias
The language used is generally neutral and informative, using technical terms precisely. However, terms like "explosion" in relation to the volume of models, and "radical shift" in relation to data infrastructure, might inject a slightly sensationalist tone. Using more measured language would enhance neutrality.
Bias by Omission
The article focuses heavily on the opinions and predictions of Zuzanna Stamirowska, CEO of Pathway, and doesn't include diverse perspectives from other experts in the field of AI data infrastructure. This omission might limit the reader's understanding of the challenges and opportunities in the evolving AI model landscape. While acknowledging space constraints, including a counterpoint or alternative view would strengthen the analysis.
False Dichotomy
The article presents a somewhat simplistic dichotomy between batch processing and real-time data processing, suggesting that the latter is a clear and inevitable superior solution. It doesn't fully explore the potential benefits or use cases where batch processing might remain efficient and appropriate, potentially misleading the reader into believing it's universally obsolete.
Gender Bias
The article centers around the perspective and expertise of Zuzanna Stamirowska. While her insights are valuable, a lack of other voices might unintentionally perpetuate a gender imbalance in portraying leadership within the AI industry. Including additional expert opinions from a diverse range of genders would improve the representation.
Sustainable Development Goals
The article discusses the rapid evolution of AI models, highlighting innovations in data infrastructure and real-time data engineering. This directly contributes to advancements in technology and infrastructure, key aspects of SDG 9. The development of "live AI" and efficient data pipelines improves the speed and efficiency of AI development and deployment, fostering innovation and infrastructure improvements.