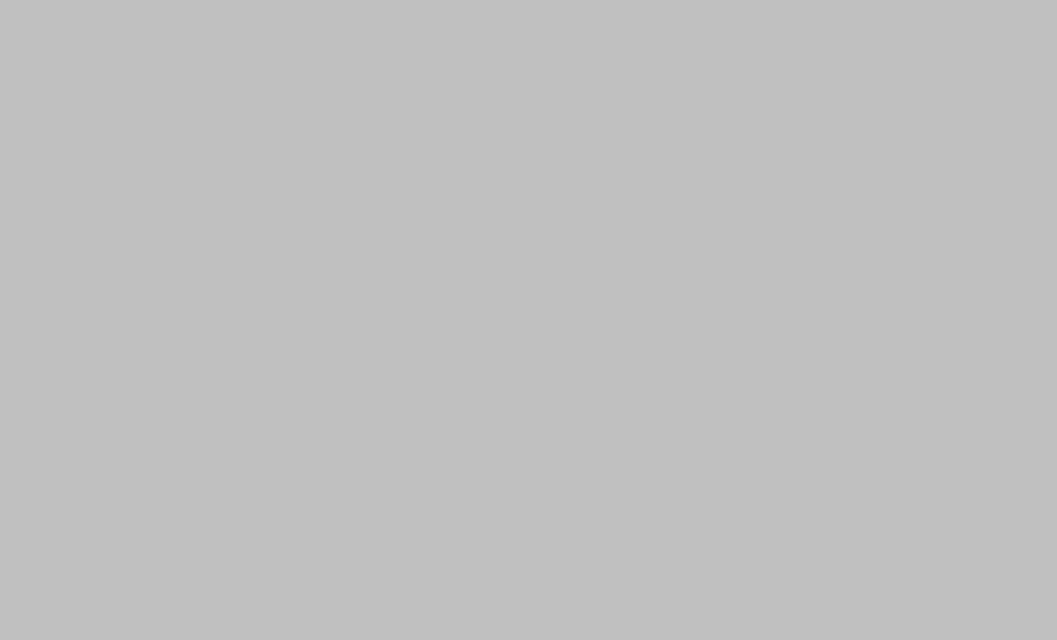
elpais.com
Algorithms in Law Enforcement: Balancing Efficiency and Equity
Algorithms are increasingly used in law enforcement and the justice system to predict crime and recidivism, raising concerns about bias, transparency, and accountability, despite potential benefits in consistency and efficiency.
- What are the specific ways in which algorithms used for risk assessment in the justice system can perpetuate or amplify existing inequalities?
- Algorithms, often using supervised learning, predict recidivism or crime likelihood based on historical data. While potentially correcting human biases in pre-trial decisions, they risk encoding existing inequalities if training data reflects historical discrimination.
- What measures are necessary to ensure that the use of algorithms in public safety aligns with democratic values and principles of justice and fairness?
- The use of algorithms in law enforcement necessitates a democratic conversation about transparency, oversight, and ethical considerations. Private companies developing these tools lack the same accountability as the state, raising concerns about fairness and justice.
- How do algorithms used in law enforcement and the justice system impact fairness and accountability, considering their potential for bias and lack of transparency?
- Algorithms are increasingly used in law enforcement and justice, impacting decisions on arrests, police deployment, and suspect identification. These tools promise greater consistency but raise concerns about fairness and accountability.
Cognitive Concepts
Framing Bias
The article presents a balanced perspective, acknowledging both the potential for algorithms to improve the justice system and the significant risks of bias and lack of accountability. While it highlights the concerns, it also presents arguments in favor of algorithmic tools if used responsibly and with sufficient oversight. The framing is generally neutral and avoids overly sensationalizing either side.
Bias by Omission
The article adequately discusses the potential biases in algorithms used in law enforcement, but it could benefit from mentioning specific examples of algorithms currently in use and the data sets they rely on. While the risks of biased data are highlighted, concrete examples of how this bias manifests in real-world applications would strengthen this section. Also, the discussion of oversight is strong but could mention specific examples of successful or unsuccessful oversight mechanisms in place.
Gender Bias
The analysis doesn't explicitly discuss gender bias within the algorithms or data sets used. While race and ethnicity are highlighted as sources of bias, the potential for gender bias is overlooked. This omission limits the comprehensiveness of the analysis.
Sustainable Development Goals
The article highlights how algorithms used in criminal justice can perpetuate existing inequalities and biases, leading to unfair outcomes and undermining the principles of justice and equal treatment under the law. The lack of transparency and accountability in the development and deployment of these algorithms further exacerbates this negative impact. The potential for biased algorithms to disproportionately affect marginalized communities raises serious concerns about the fairness and legitimacy of the justice system.