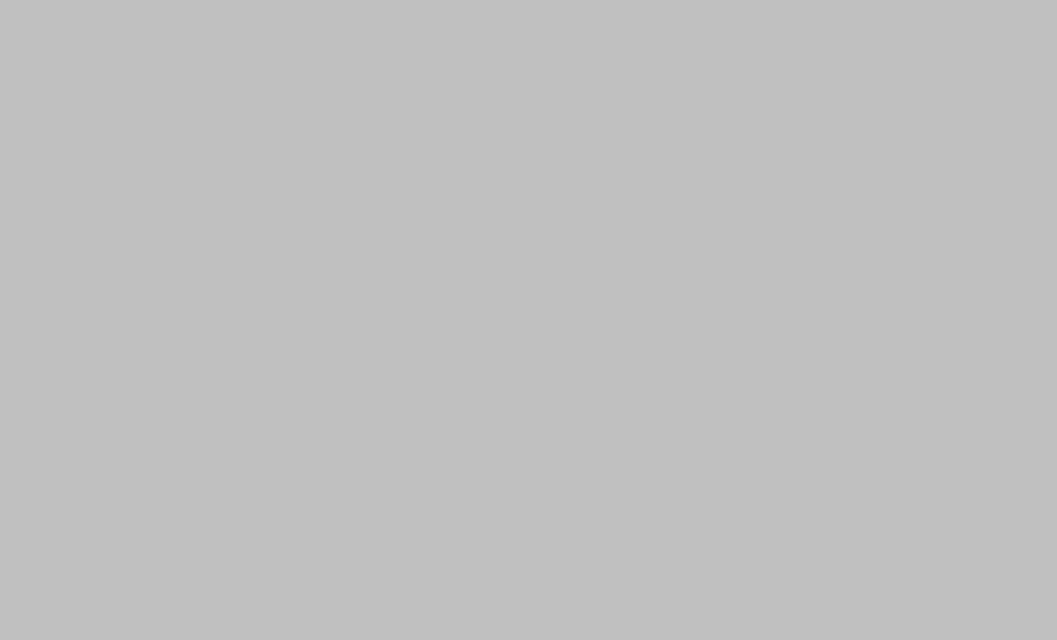
theglobeandmail.com
Canada Investigates AI-Driven Algorithmic Pricing in Rental Market
Canada's Competition Bureau is investigating AI-driven algorithmic pricing in real estate rentals following an American antitrust lawsuit alleging collusion and rent inflation by companies using RealPage's YieldStar platform; the investigation was prompted by the NDP and a motion by B.C. NDP MP Bonita Zarrillo.
- What are the potential long-term implications of this investigation for AI regulation and the Canadian rental market?
- This investigation could significantly impact the Canadian rental market and AI regulation. Potential outcomes include fines, policy changes banning AI-driven pricing practices, and increased scrutiny of algorithmic transparency in other industries. The outcome will influence future discussions about AI's role in price setting and consumer rights.
- What is the immediate impact of the Competition Bureau's investigation into AI-driven algorithmic pricing on Canadian renters?
- The Competition Bureau of Canada is investigating the use of AI-driven algorithmic pricing in the country's real estate rental market, prompted by an American antitrust lawsuit alleging collusion and artificial rent inflation by dozens of companies using RealPage's YieldStar platform. This follows an NDP request and a motion by B.C. NDP MP Bonita Zarrillo.
- How does the American antitrust lawsuit involving RealPage's YieldStar platform inform the Canadian Competition Bureau's investigation?
- The investigation connects to a broader pattern of algorithmic price manipulation in various sectors, raising concerns about market fairness and consumer protection. The U.S. lawsuit, involving six corporate landlords and RealPage's YieldStar platform, provides key evidence for the Canadian inquiry, highlighting the potential for cross-border algorithmic collusion.
Cognitive Concepts
Framing Bias
The framing emphasizes the political response and accusations of conflict of interest, potentially overshadowing the central issue of algorithmic pricing and its impact on renters. The headline and early focus on political maneuvering and accusations may lead readers to prioritize political angles over the broader economic consequences for renters. The inclusion of Zarrillo's comments linking Freeland's policy to Carney's past associations frames the issue in a partisan light.
Language Bias
The article uses charged language such as "scheme," "squeeze renters," and "artificially inflate rents." While these phrases reflect the seriousness of the allegations, they are not strictly neutral and could sway reader opinion. More neutral alternatives would be "alleged scheme," "increase rents," and "influence rent prices.
Bias by Omission
The article focuses heavily on the political responses and potential conflicts of interest, but provides limited details on the actual mechanics of AI-driven algorithmic pricing in real estate. The technical aspects of how these algorithms function and the specific data used are not explored in depth. While the article mentions the YieldStar platform, it does not delve into its functionality. There is also a lack of information regarding the scale and impact of this practice on Canadian renters.
False Dichotomy
The article presents a somewhat simplistic dichotomy between corporate landlords using AI to inflate rents and politicians advocating for renters. The nuanced challenges of regulating AI in the market and potential unintended consequences of a ban are not fully explored.
Sustainable Development Goals
The investigation and potential ban on AI-driven algorithmic pricing in the rental market aim to address issues of affordability and fairness, which directly impact income inequality. By preventing artificial inflation of rents, the measures could lead to more equitable access to housing and reduce the financial burden on renters, particularly low-income households.