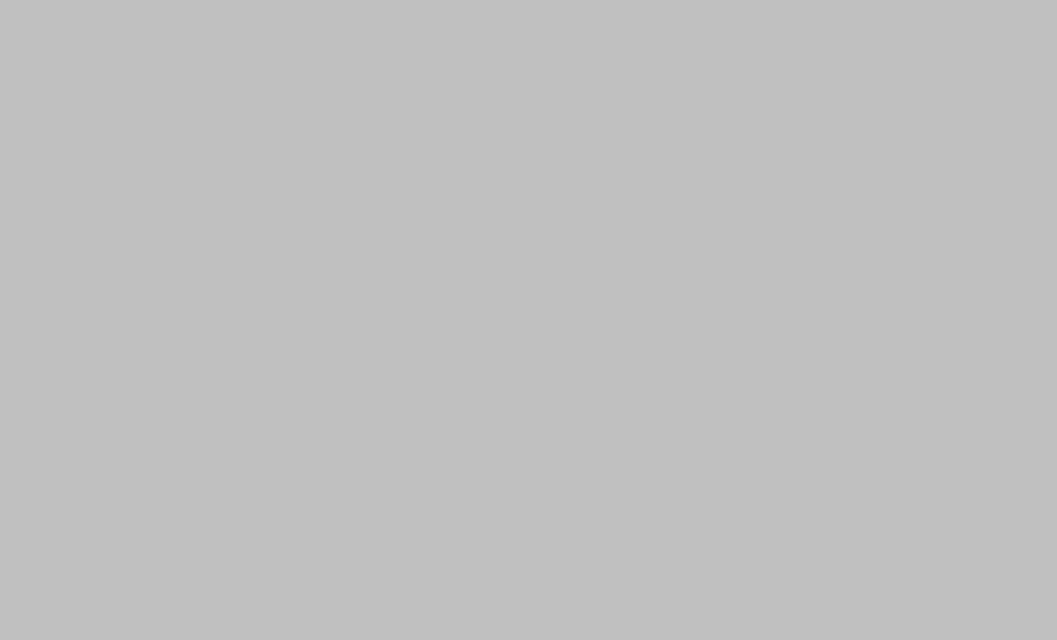
theglobeandmail.com
Cohere Shifts AI Strategy to Customized Enterprise Models
Canadian AI startup Cohere, valued at $5.5 billion, is shifting its focus from building large language models to creating customized AI models for enterprise clients, reflecting diminishing returns from scaling up models and a greater customer demand for practical, task-specific solutions.
- What are the potential long-term implications of Cohere's strategy for the AI industry and its competitive landscape?
- Cohere's focus on enterprise-specific AI models positions it uniquely within the competitive landscape. By prioritizing practical application over theoretical advancements like AGI, the company aims for faster deployment and demonstrable value for its clients. This approach could lead to more rapid adoption and a stronger market position.
- What factors contributed to Cohere's decision to prioritize customized model development over larger foundation models?
- Cohere's strategic pivot highlights a broader industry trend: diminishing returns from simply scaling up AI models. Competitors like OpenAI are facing delays and plateauing results from this approach. Cohere's focus on customization offers a potentially more capital-efficient path to success, reducing reliance on massive computational resources.
- What is Cohere's new strategic focus, and how does it differ from the current industry trend of developing larger AI models?
- Cohere, a $5.5 billion Canadian AI startup, shifts its strategy from developing large foundation models to creating customized AI models for enterprise clients. This change reflects customer demand for practical, task-specific solutions rather than general-purpose models. The company will continue developing foundation models but prioritize improving existing models instead of solely increasing their size.
Cognitive Concepts
Framing Bias
The article frames Cohere's strategy shift positively, emphasizing its capital efficiency and focus on customer needs. The headline and opening paragraphs highlight the company's pivot to customized models as a smart and forward-thinking move. While it mentions challenges in scaling large language models, this is presented more as a justification for Cohere's strategy than a balanced assessment of industry-wide trends. The positive framing might influence readers to view Cohere's approach as superior without presenting a full comparative analysis.
Language Bias
The language used is largely neutral, but there are instances of framing that might subtly favor Cohere. Phrases like "smart and forward-thinking move" and "capital-efficient" carry positive connotations. More neutral alternatives could be "strategic shift" and "cost-effective". The repeated mention of Cohere's success in attracting major investors further paints a positive picture.
Bias by Omission
The article focuses heavily on Cohere's shift in strategy and does not delve into the potential drawbacks or challenges of this approach. It also omits discussion of Cohere's competitors beyond a brief mention of OpenAI and Anthropic, leaving out a broader competitive landscape analysis. Further, the article lacks details on the specific types of customization Cohere offers and the technical limitations of their approach. While some limitations are inherent due to article length and audience, the omissions affect the reader's ability to form a complete picture.
False Dichotomy
The article presents a somewhat simplistic dichotomy between focusing on larger foundation models versus smaller, customized models. It suggests that pursuing larger models is less efficient and less effective for enterprise clients, while overlooking potential benefits of foundation models that may outweigh the costs for specific applications. The presentation of this dichotomy might oversimplify a more complex and nuanced decision faced by AI companies.