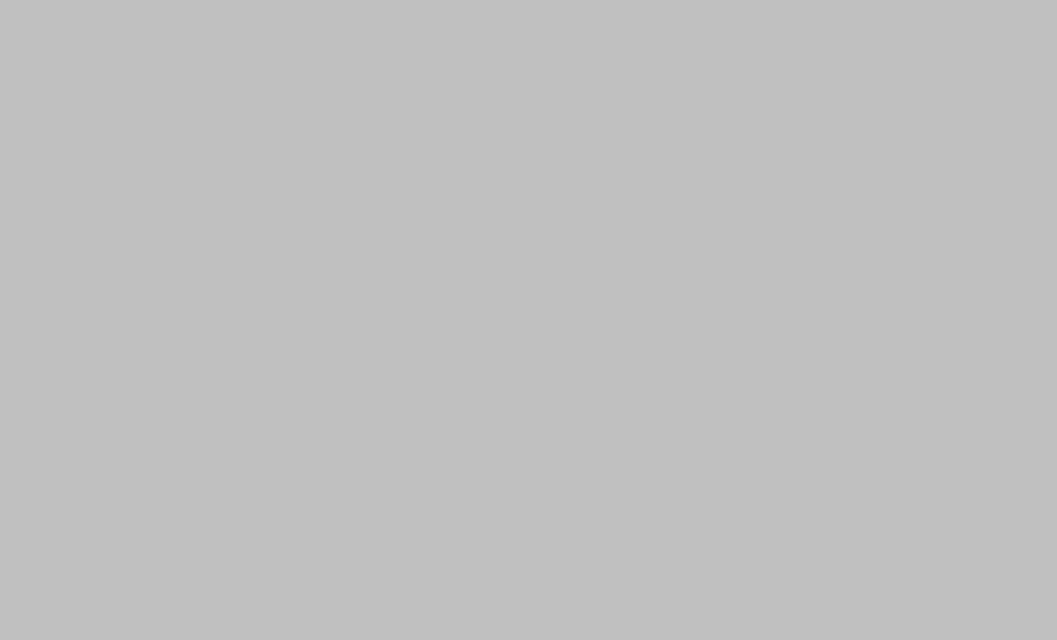
cnbc.com
Data Infrastructure and Cultural Barriers Hinder Business AI Adoption
Despite significant investment, many businesses struggle with AI adoption due to insufficient data infrastructure and cultural barriers; a survey of 500 U.S. senior business leaders reveals 83% need stronger data infrastructure for faster AI implementation, while two-thirds see a lack of infrastructure as a major obstacle.
- How does the lack of a robust data infrastructure impact the successful implementation of AI initiatives?
- The biggest challenge to AI adoption is building a robust data infrastructure capable of supporting AI initiatives. This requires a cohesive enterprise strategy rather than a project-by-project approach, enabling data integration and creating a "flywheel" effect where each new initiative is easier. Poor data quality, stemming from siloed and unreliable information, further compounds the problem.
- What are the primary obstacles hindering businesses from maximizing their return on investment in artificial intelligence?
- Businesses are investing heavily in AI, but many lack the infrastructure and skills for optimal returns. A survey of 500 U.S. senior business leaders showed that 83% would see faster AI adoption with stronger data infrastructure, and two-thirds cite infrastructure as a current impediment. This lack of infrastructure leads to poor data quality, hindering AI effectiveness.
- What strategies can organizations employ to overcome cultural resistance to AI adoption and ensure responsible AI integration?
- Future success with AI hinges on addressing data infrastructure and cultural hurdles. Organizations must invest in robust data governance, automation for data cleaning, and upskilling initiatives to build AI literacy and overcome anxieties around job displacement. Open communication is crucial to fostering a culture that embraces AI, rather than fearing it.
Cognitive Concepts
Framing Bias
The article frames AI adoption as primarily a challenge-filled journey, emphasizing difficulties more than successes. The headline (if there was one) likely would reflect this. The lead paragraphs highlight obstacles to AI adoption before discussing potential benefits. This framing may disproportionately influence reader perception of AI's overall impact.
Language Bias
The language used is generally neutral and informative. Terms like "messy, siloed, and unreliable information" are descriptive, but could be softened to "complex and fragmented data." The phrase "AI fatigue" is slightly loaded but accurately reflects the cited research.
Bias by Omission
The article focuses heavily on the challenges of AI adoption, particularly data infrastructure and skills gaps. While it mentions the potential benefits of AI, it doesn't delve into specific examples of successful AI implementations or counterarguments to the challenges presented. This omission could lead readers to underestimate the positive aspects of AI and overemphasize the difficulties. The lack of diverse voices beyond the two experts quoted also limits the scope of perspectives.
False Dichotomy
The article doesn't explicitly present false dichotomies, but it implicitly frames AI adoption as a binary choice: either overcome significant hurdles or remain behind. The nuanced reality of incremental progress and varied levels of AI integration is understated.
Sustainable Development Goals
The article discusses the challenges and opportunities of AI adoption in businesses. Successful AI integration can drive innovation, boost operational efficiency, and create new opportunities, aligning with SDG 9 (Industry, Innovation, and Infrastructure) which promotes building resilient infrastructure, promoting inclusive and sustainable industrialization and fostering innovation. Overcoming hurdles like data infrastructure limitations and skills gaps is crucial for realizing AI's potential to improve industrial processes and drive economic growth.