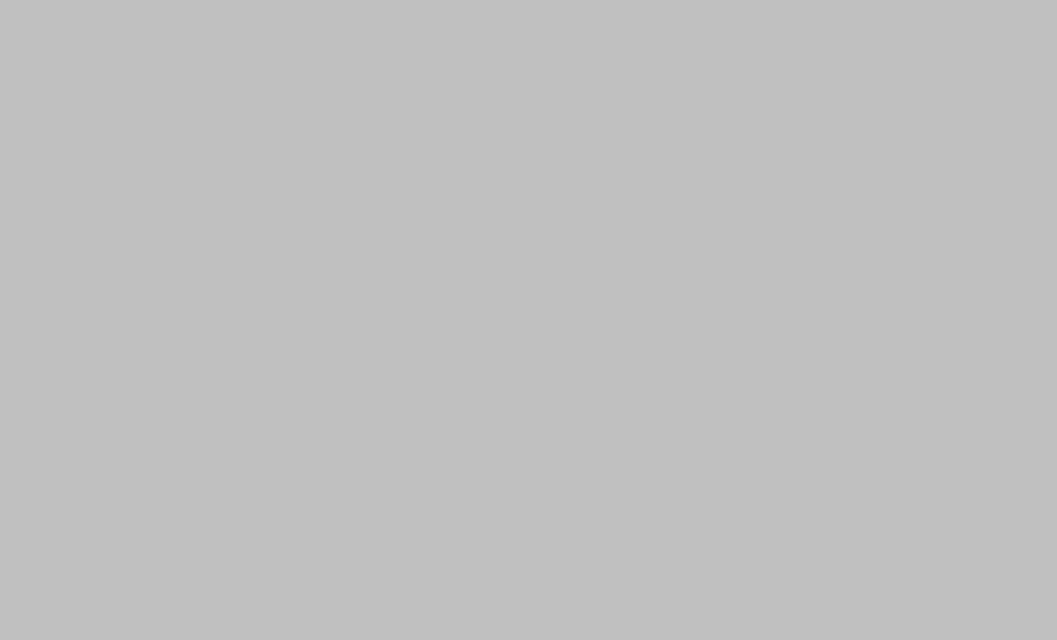
forbes.com
Debunking Three AI Myths: Prompt Engineering, Detectors, and True Literacy
Three widely held assumptions about artificial intelligence—that prompt engineering is a career, AI detectors are reliable, and using AI equals understanding AI—are challenged, with 2025 predicted as the year these misconceptions will be debunked due to practical limitations and the increasing sophistication of AI.
- How do the internal workings of AI models render AI detection tools unreliable, and what alternative methods are emerging to address this issue?
- The article debunks three common misconceptions surrounding AI. Firstly, prompt engineering's perceived career potential is challenged by the increasing ease of use of LLMs. Secondly, the inherent limitations of AI detection tools are highlighted, with their failure rate rising. Finally, it distinguishes between simply using AI and understanding its underlying mechanisms, arguing that true AI literacy necessitates the latter for professionals whose jobs are increasingly automated.
- What are the three major misconceptions about AI highlighted in the article, and what are their immediate implications for the job market and public perception of AI?
- Prompt engineering is not a viable career path, similar to searching on Google. AI detectors are unreliable due to the internal mechanisms of AI generation, and their flaws are becoming increasingly apparent. The assumption that using AI equals understanding AI is also false; true AI literacy requires understanding how AI systems function.
- What is the critical difference between using AI tools and achieving true AI literacy, and why is the latter increasingly important for maintaining professional competitiveness in the rapidly evolving AI landscape?
- The year 2025 will likely witness a shift in perspective regarding AI. The unreliability of prompt engineering as a profession and AI detection tools will become widely accepted. Furthermore, the growing importance of understanding AI's inner workings, beyond mere usage, will be recognized as crucial for maintaining professional relevance in the face of rapid technological advancements.
Cognitive Concepts
Framing Bias
The narrative frames the author's predictions as certainties, using phrases like "I expect 2025 is the year when." This framing presents personal opinions as objective truths and could mislead readers into accepting these predictions without critical evaluation. The title itself sets a predictive tone, potentially influencing the reader's perception before engaging with the content.
Language Bias
The language used is generally neutral, but phrases like "safe bets" and "thoroughly debunked" express a degree of confidence that might be perceived as subjective. The author's own experience is used to support the argument, which lacks broader empirical evidence.
Bias by Omission
The analysis lacks diverse perspectives on the future of AI and prompt engineering. It focuses heavily on the author's predictions and doesn't include counterarguments or expert opinions on the discussed trends. The potential for prompt engineering as a career, beyond the author's opinion, is not explored.
False Dichotomy
The article presents a false dichotomy between 'using AI' and 'understanding AI.' It oversimplifies the complexities of AI literacy and fails to acknowledge that different levels of understanding might be necessary for different roles and individuals.
Sustainable Development Goals
The article highlights the misconception that using AI tools equates to AI literacy. It emphasizes the need for a deeper understanding of AI functionality, particularly for those whose jobs are impacted by AI advancements. This aligns with SDG 4 (Quality Education) by advocating for a more comprehensive and relevant education in AI, moving beyond simple tool usage to encompass a functional understanding of the technology. This ensures individuals can adapt to the changing job market and contribute meaningfully in an AI-driven world.