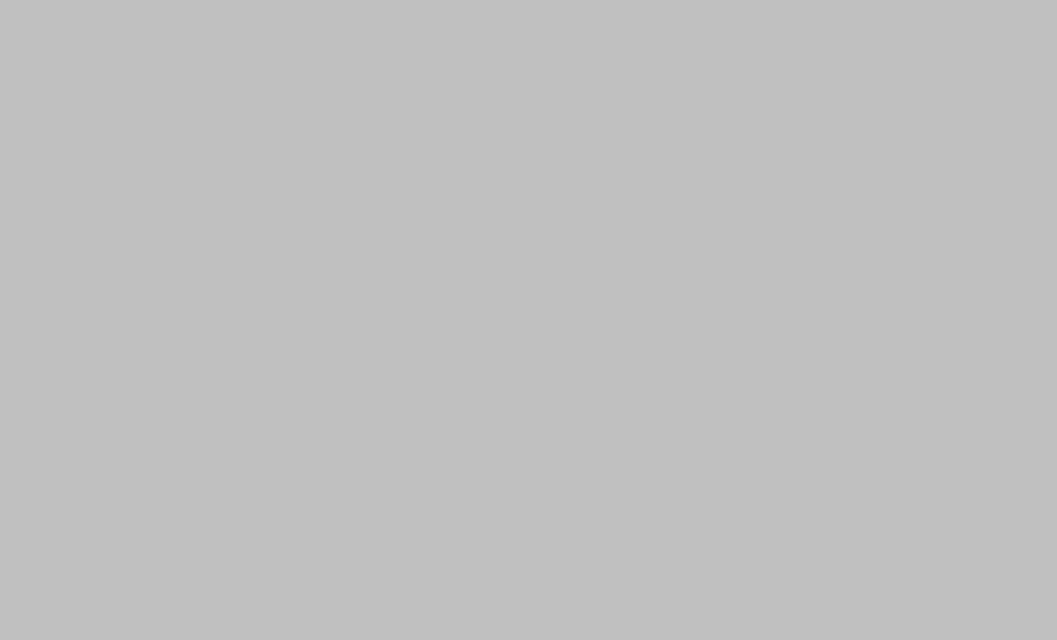
forbes.com
Decentralized Data Collection: A New Era for AI Development
This article examines the shift from centralized to decentralized data collection, highlighting blockchain's role in improving data diversity, transparency, and accessibility for AI; it also lists five key decentralized data platforms.
- How does blockchain technology enhance transparency and data diversity in decentralized data collection compared to centralized models?
- Decentralized data collection leverages blockchain technology to overcome limitations of centralized approaches. By offering incentives for data contributions and recording data handling on the blockchain, it promotes transparency, data diversity across regions and cultures, and user control over data usage.
- What are the potential long-term impacts of decentralized data collection on the ethical sourcing and accessibility of data for AI development?
- The future of AI development depends heavily on access to diverse, high-quality datasets. Decentralized data collection, still in its early stages, presents a scalable and ethical solution to address current limitations of centralized systems, promising wider data accessibility and improved AI model performance.
- What are the primary challenges of traditional data sourcing methods for AI development, and how does decentralized data collection offer a solution?
- Traditional centralized data collection, relying on single-point control, struggles with global data diversity and transparency. Decentralized methods, using blockchain, offer solutions by incentivizing global data contributions and enhancing transparency through distributed data storage and verifiable processes.
Cognitive Concepts
Framing Bias
The article is framed positively towards decentralized data collection, highlighting its advantages and minimizing potential shortcomings. The headline and introduction immediately establish the superiority of decentralized methods. The choice to include a shortlist of specific platforms reinforces this bias by implicitly endorsing these options, rather than offering a balanced comparison of all available technologies. The author's position as founder and CEO of one of the mentioned companies is a clear conflict of interest and further affects the framing.
Language Bias
The article uses positive and enthusiastic language when describing decentralized data collection ("strong alternative," "robust data strategies," "opening access to new, previously untapped data sources"). In contrast, the description of centralized systems is notably less favorable, using terms like "bottleneck" and "black boxes." This uneven tone influences the reader's perception.
Bias by Omission
The article focuses heavily on the benefits of decentralized data collection and offers a limited perspective on the challenges or drawbacks. While it mentions the "black box" nature of centralized systems, it doesn't delve into potential downsides of decentralized approaches such as security vulnerabilities, scalability issues, or the potential for data manipulation within a decentralized environment. The lack of critical analysis of decentralized systems could mislead the reader into believing it's a perfect solution.
False Dichotomy
The article presents a stark contrast between centralized and decentralized data collection, portraying them as mutually exclusive and binary opposites. It overlooks hybrid models or strategies that combine elements of both approaches to leverage their respective strengths. This simplification might cause readers to reject centralized systems outright without considering their potential value in certain contexts.
Sustainable Development Goals
Decentralized data collection platforms offer a more equitable approach to data sourcing by enabling participation from diverse regions and cultures, which were previously underrepresented in centralized models. This can lead to fairer representation in AI systems and algorithms, reducing biases and promoting inclusivity.