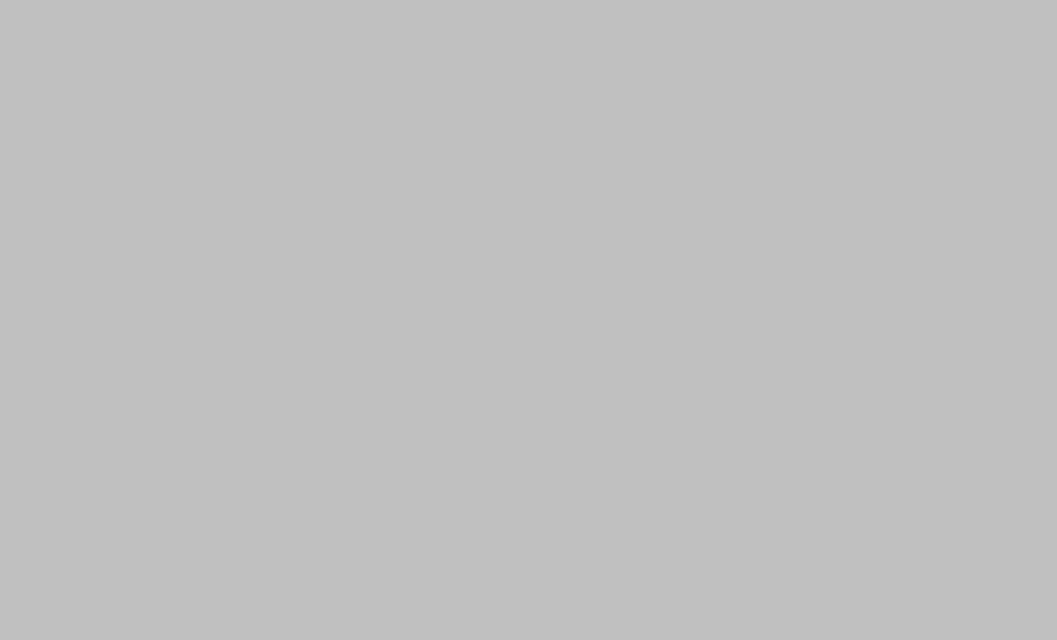
forbes.com
DeepSeek Ban Exposes Generative AI's Privacy Risks
The U.S. government banned DeepSeek from federal devices due to data routing concerns to China, highlighting broader generative AI privacy vulnerabilities and prompting calls for proactive solutions like Privacy-Enhancing Technologies (PETs) to protect sensitive data.
- What are the immediate implications of the U.S. government's ban on DeepSeek, and how does it impact the broader adoption of generative AI?
- The U.S. government's ban on DeepSeek from government devices highlights critical privacy vulnerabilities in generative AI. Kurt Rohloff, CTO of Duality Technologies, points to the fact that 58.6% of consumers are extremely or very concerned about AI privacy violations, emphasizing the need for proactive solutions. This concern is especially relevant in sectors like healthcare, finance, and government where data breaches have severe consequences.
- How do the inherent vulnerabilities of generative AI models contribute to data privacy risks, and what are the potential consequences in various sectors?
- The DeepSeek ban reveals a systemic issue: most generative AI systems lack the privacy architecture needed to meet regulatory requirements across various sectors. Rohloff explains that the models' ability to learn from all ingested data—user prompts, documents, and behavioral cues—creates a significant attack surface, potentially leading to the leakage of sensitive information. This is further underscored by the average $9.8 million cost of a data breach in the healthcare industry.
- What innovative solutions, such as Privacy-Enhancing Technologies, can address the systemic privacy challenges in generative AI, and what role should leadership play in their adoption?
- The future of secure AI hinges on integrating Privacy-Enhancing Technologies (PETs), particularly Fully Homomorphic Encryption (FHE), into system design. FHE allows computations on encrypted data without decryption, preventing data exposure at rest, in transit, and in use. This approach enables secure cross-organizational collaboration, ensuring compliance with data privacy regulations and mitigating the risk of model compromise, thus rebuilding trust in AI systems.
Cognitive Concepts
Framing Bias
The framing emphasizes the risks and vulnerabilities of generative AI, highlighting negative consequences and security breaches. While this is important, the overwhelmingly negative tone might skew public perception and overshadow potential benefits or mitigating strategies. The headline (if any) would further influence this perception. The use of strong quotes about catastrophic risks further reinforces this framing.
Language Bias
The language used is generally strong and emphasizes negative consequences, using words like "vulnerabilities," "catastrophic," and "risks." While accurate, the consistent use of such language creates a tone of alarm that could be toned down for more neutral reporting. For example, instead of "catastrophic consequences," a more neutral phrasing might be "significant consequences.
Bias by Omission
The article focuses heavily on the concerns of Kurt Rohloff and the risks of generative AI, but it could benefit from including perspectives from AI developers, government officials involved in the DeepSeek ban, or representatives of DeepSeek itself. This would provide a more balanced view and allow readers to consider different viewpoints on the security and regulatory challenges. The article also doesn't mention potential benefits of generative AI, which could be seen as a bias by omission.
False Dichotomy
The article presents a somewhat simplistic eitheor framing, suggesting that either strong encryption (like FHE) is the solution or current practices lead to catastrophic failure. The reality is likely more nuanced, with multiple approaches and layers of security potentially necessary.
Gender Bias
The article primarily focuses on the perspective of Kurt Rohloff, a male CTO. While there is no overt gender bias, the lack of female voices in the discussion of AI security could subtly reinforce existing gender imbalances in the tech field. Including female experts' perspectives would enhance balance and diversity.
Sustainable Development Goals
The article highlights the disproportionate impact of AI data breaches on different sectors (e.g., healthcare facing higher costs). Addressing AI security through Privacy-Enhancing Technologies (PETs) like Fully Homomorphic Encryption (FHE) can help mitigate these risks and reduce the economic inequality resulting from data breaches impacting vulnerable populations more severely. By enabling secure data collaboration across organizations, FHE can promote fairer access to data and insights, which can reduce inequalities in healthcare, finance, and other sectors.