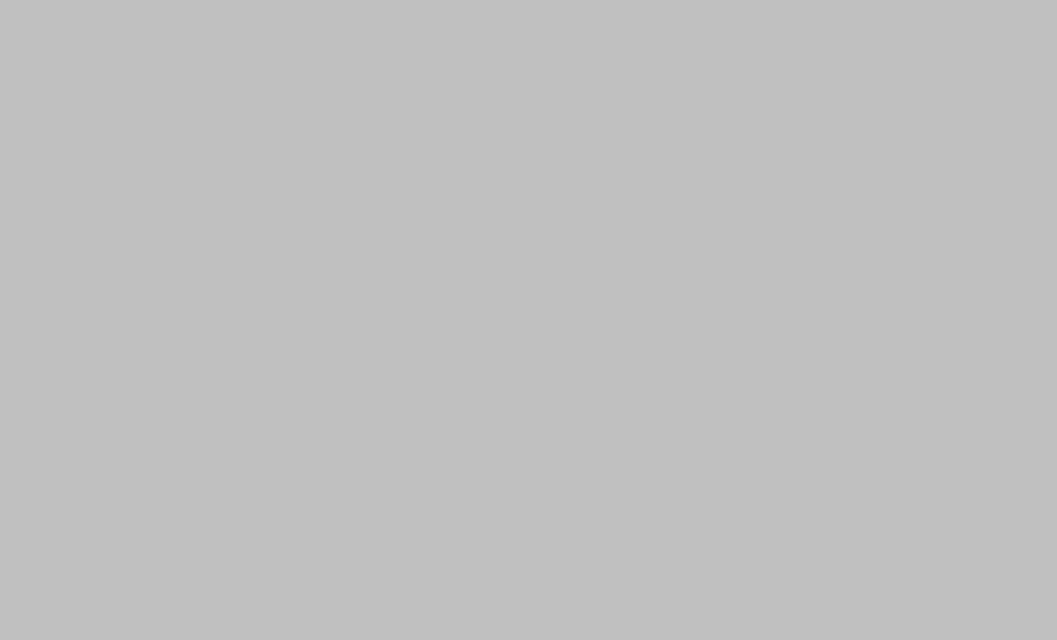
taz.de
DeepSeek's Low-Cost AI Rivals Top Models, Sending Shockwaves Through Tech
DeepSeek's new AI model, DeepSeek-R1, rivals leading models despite a \$5.6 million development cost, causing an \$18% drop in Nvidia's stock value and challenging prevailing AI development cost norms; the model uses a unique training method that could be more transparent but also more susceptible to bias.
- How does DeepSeek-R1's training methodology differ from established methods, and what are the associated risks and benefits?
- The success of DeepSeek-R1 challenges the prevailing notion of exorbitant AI development costs. While companies like OpenAI, Microsoft, and others invest billions, DeepSeek's significantly lower budget demonstrates an alternative approach to AI development. Nvidia, a major chip manufacturer crucial for AI training, experienced an \$18% drop in stock value, representing the largest single-day loss in stock market history.
- What are the immediate implications of DeepSeek-R1's success, considering its low development cost and competitive performance?
- DeepSeek, a relatively unknown Chinese company, unveiled its AI model, DeepSeek-R1, which performed comparably to established models despite a development cost of only \$5.6 million. This has caused a significant drop in the stock values of some chip manufacturers.
- What are the long-term implications of DeepSeek's decision to open-source the code but not the training data, particularly concerning potential biases and censorship?
- DeepSeek-R1's training methodology, which omits human oversight of intermediate steps, allows the AI to develop its own evaluation methods. While this leads to transparency and improved problem-solving, it also introduces risks regarding potential biases and deceptive behavior. The decision to open-source the code but not the training data raises concerns about potential censorship and underlying biases influencing the AI's responses.
Cognitive Concepts
Framing Bias
The article frames DeepSeek's success as a challenge to the established narrative of expensive AI development. The headline and opening paragraphs emphasize DeepSeek's low cost and surprising performance, setting a critical tone towards high-budget AI projects. This framing could lead readers to question the value of large investments in AI.
Language Bias
The article uses loaded language, such as "Schock" (shock) and "absurd" when describing the cost of other AI projects. These terms inject subjective opinions. The description of DeepSeek's approach as "synthetischer" (synthetic) could also be considered loaded, as this word is rarely used in neutral AI discussion. Neutral alternatives could include "novel", "unconventional", or "different".
Bias by Omission
The article omits discussion of DeepSeek's data sources beyond mentioning that they did not release their training data, and that the responses seem affected by Chinese censorship. This omission is significant because training data heavily influences AI bias, and the lack of transparency prevents a full evaluation of the model's potential biases.
False Dichotomy
The article presents a false dichotomy by contrasting DeepSeek's low development cost with the massive investments of other companies, implying that high costs are inherently necessary for successful AI development. This ignores the possibility of alternative development strategies and other contributing factors to AI success.
Gender Bias
The article uses gender-neutral language (e.g., Nutzer:innen) which is positive. However, a more thorough analysis would require examining the gender distribution in the sources cited and whether gender played a role in the portrayal of individuals involved.
Sustainable Development Goals
The article highlights that a relatively small Chinese company, DeepSeek, developed a competitive AI model with significantly less funding than large established companies. This challenges the prevailing narrative of massive investments required for AI development and could potentially reduce the financial barrier to entry for smaller players, fostering more inclusive innovation in the field.