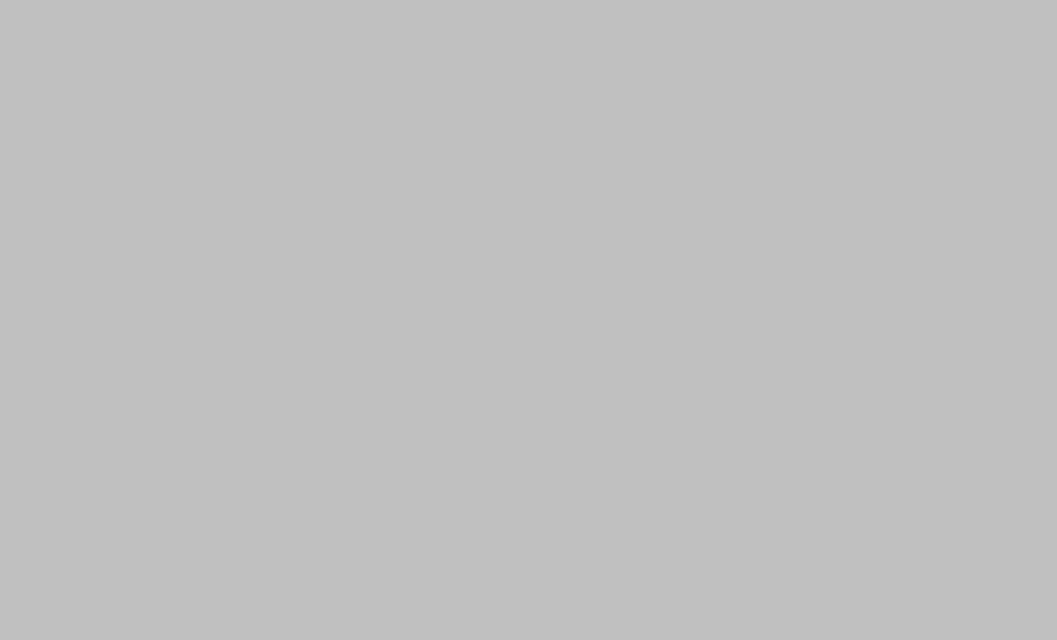
sueddeutsche.de
Digital Tools to Optimize Rural Public Transportation in Germany
Hof University leads a new project using digital tools and machine learning to optimize rural public transportation in Germany, integrating various transport modes to improve efficiency and accessibility, funded by the Federal Ministry of Digital and Transport with 1.8 million euros.
- How will the integration of different data sources contribute to creating an accurate and effective model for optimizing rural public transportation?
- The project uses a digital twin and machine learning to analyze anonymized data from various sources (census, vehicle movements, mobile phone usage, public transit data). This will create a model to simulate and optimize various scenarios, including changes to schedules and stops, aiming for better efficiency and coverage.
- What are the potential long-term impacts of this project on rural communities, considering accessibility, economic development, and social inclusion?
- The project's digital tools are designed to assist human planners, not replace them. The resulting system will allow for a more comprehensive rural public transportation network. The integration of various modes, potentially including autonomous vehicles, will be crucial for making rural areas more attractive and accessible.
- What are the primary challenges in providing effective public transportation in rural areas, and how does this project aim to address them using digital technology?
- A new study at Hof University aims to improve rural public transportation using digital tools. Current approaches, like on-demand services, are limited by geographical constraints, leading to many empty vehicles. The project will integrate various transport modes, including on-demand, scheduled, and ride-sharing services, to optimize routes and reduce wasted resources.
Cognitive Concepts
Framing Bias
The article frames the issue as a challenge to be solved through technological innovation. The emphasis is on the potential benefits of the new project and the positive impact on rural areas. While this framing is understandable given the context of the project announcement, it could be balanced by including perspectives on potential drawbacks or obstacles. The headline (if there was one) would likely heavily influence this framing, but we can not see it here.
Language Bias
The language used is generally neutral and objective. Terms like "uncomplicated" and "rapid" could be considered slightly positive, but they are appropriate within the context of describing desired improvements to public transport. There's no use of loaded language or emotionally charged terms.
Bias by Omission
The article focuses on the challenges of public transportation in rural areas and a new project to improve it. While it mentions the involvement of various stakeholders (TU Munich and unnamed companies), it doesn't detail their specific roles or contributions. This omission doesn't significantly affect the core message but could provide a richer understanding of the project's collaborative nature. The article also doesn't discuss potential downsides or challenges associated with using large datasets of personal mobility data, such as privacy concerns or potential biases in the data itself.
Sustainable Development Goals
The project aims to improve public transportation in rural areas using digital tools. This directly contributes to SDG 11 by enhancing sustainable urban mobility, making rural areas more accessible and attractive, and potentially reducing traffic congestion and greenhouse gas emissions from private vehicles.