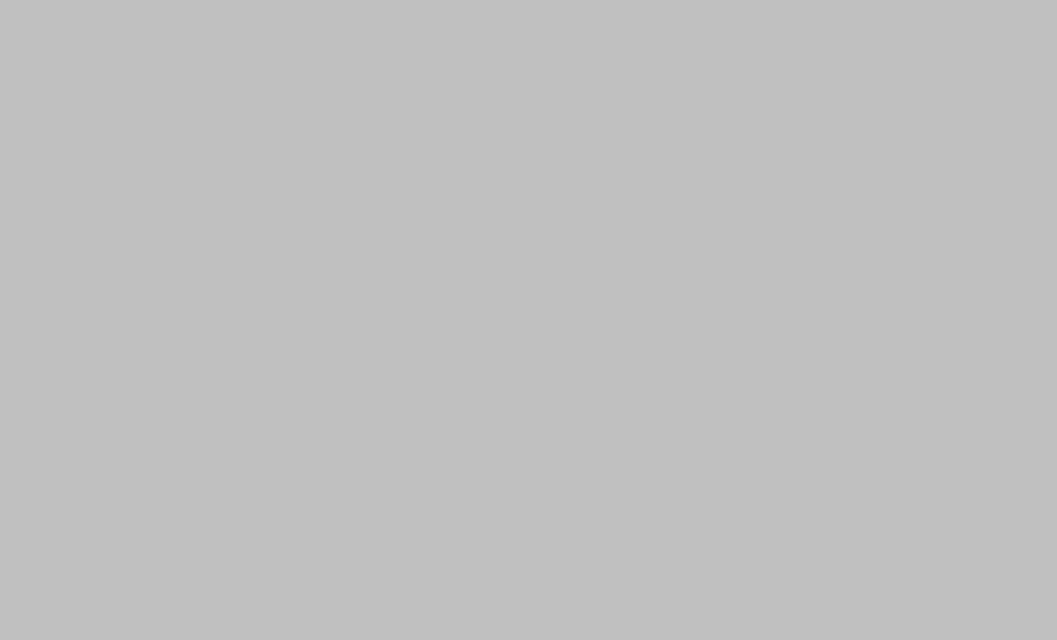
forbes.com
Dynex's qdLLM: A Quantum-Inspired LLM Achieving 10x Speed and 90% Reduced Resource Use
Dynex's Quantum Diffusion Large Language Model (qdLLM), a finalist in the SXSW 2025 innovation awards, uses a diffusion model and decentralized GPUs to achieve claimed 10x speed and 90% reduction in GPU resource use compared to existing LLMs, potentially revolutionizing AI efficiency.
- What are the key innovations in Dynex's qdLLM, and how do they compare to existing large language models in terms of speed, efficiency, and output quality?
- Dynex, a Liechtenstein-based firm, unveiled its Quantum Diffusion Large Language Model (qdLLM), a finalist in the SXSW 2025 innovation awards. The qdLLM uses a diffusion model for parallel output generation, unlike traditional sequential transformer models, claiming faster and more efficient outputs.
- What are the potential long-term implications of Dynex's technology for the AI landscape, considering the current state of quantum computing and its projected development trajectory?
- Dynex's decentralized network of GPUs emulates quantum behavior, enabling scalability to one million algorithmic qubits. The company plans to introduce a room-temperature neuromorphic quantum chip, Apollo, by 2025, for broader integration. The claimed 90% smaller model size, 10x speed increase, and 90% reduction in GPU resource usage suggest significant energy efficiency improvements compared to current LLMs.
- How does Dynex's use of decentralized GPU networks to emulate quantum computing differ from other approaches in quantum-enhanced AI, and what are its potential advantages and limitations?
- The qdLLM's parallel processing, inspired by quantum computing principles, contrasts with sequential methods like those used by GPT-4. Dynex integrates quantum annealing for improved token selection, aiming for better coherence and reduced computational overhead. This approach is similar to efforts by Stanford, Google DeepMind, and others exploring diffusion-based transformers but differentiates itself through decentralized hardware emulation of quantum behavior.
Cognitive Concepts
Framing Bias
The article presents Dynex and its qdLLM in a very positive light, highlighting its speed, efficiency, and potential advantages. While it mentions competing technologies, the overall framing strongly favors Dynex's claims. The use of quotes from Dynex's co-founder further reinforces this positive perspective. A more balanced presentation of competing technologies and independent verification would be beneficial.
Language Bias
The article uses language that is largely neutral, although phrases like "quiet evolution," "dominating AI," and "power of quantum" carry some positive connotations. While not overtly biased, these choices subtly shape the reader's perception of Dynex's technology. More neutral alternatives could be used for a more objective presentation.
Bias by Omission
The article focuses heavily on Dynex and its qdLLM, potentially omitting other companies or research groups working on similar quantum-AI hybrid approaches. While acknowledging limitations in scope, a broader overview of the field would strengthen the analysis. Specific examples of omitted research or companies are needed for a more complete assessment.
False Dichotomy
The article presents a somewhat simplified view of the future of AI, contrasting traditional methods with Dynex's quantum-inspired approach. It doesn't fully explore the potential for various hybrid models or other advancements that might coexist with quantum computing in the AI landscape. A more nuanced exploration of potential parallel developments would improve the analysis.
Sustainable Development Goals
By increasing the efficiency and reducing the energy consumption of AI model training, Dynex's quantum-inspired approach could make AI development more accessible to researchers and organizations with fewer resources, thus potentially reducing the inequality of access to cutting-edge technology.