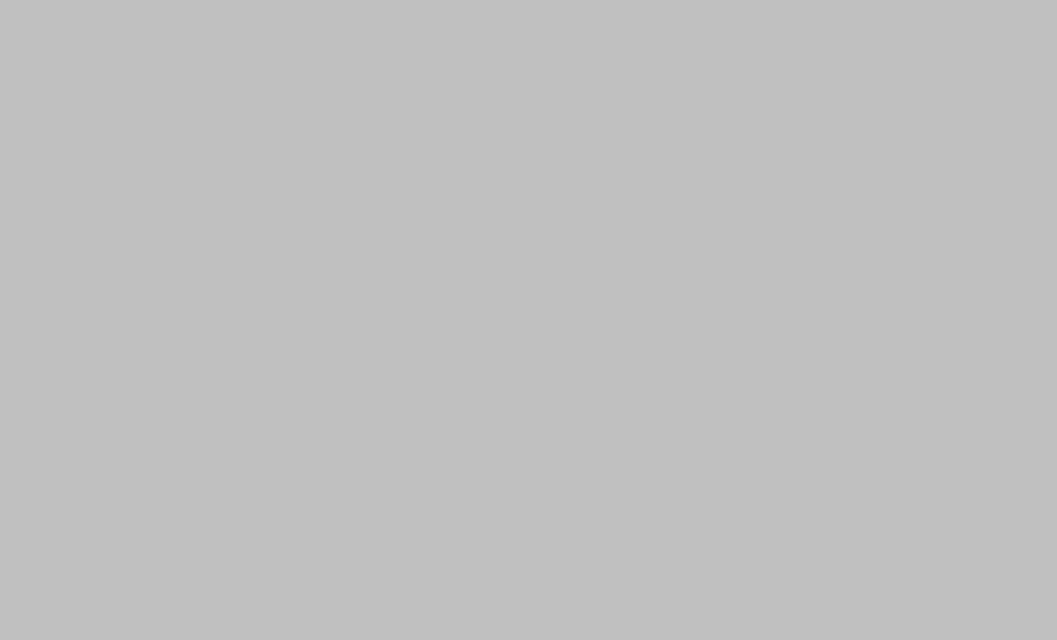
forbes.com
Enterprise AI Maturity: Overcoming Data Challenges
Poor data quality, fragmented ownership, and misaligned user experiences hinder early AI initiatives; success demands a cultural shift toward viewing data platforms as strategic enterprise assets, requiring integrated governance, balanced data integration, and foundational investments in data quality and infrastructure.
- How can organizations balance the benefits of centralized data with the need for strategic data fragmentation to maintain compliance, security, and operational agility?
- Achieving AI readiness necessitates viewing data platforms as enterprise assets, not mere technology projects. This requires a cultural shift, embedding data literacy and stewardship across all roles, fostering collaboration instead of siloed optimization. The focus should shift from data ownership to creating enterprise-wide value.
- What are the primary obstacles hindering successful early-stage AI initiatives within enterprises, and what fundamental changes are necessary to overcome these challenges?
- Early AI implementations often fail due to poor data quality, fragmented ownership, and misaligned user experiences, not technological limitations. Modernizing infrastructure without addressing these issues worsens inefficiencies. Successfully deploying AI requires reliable, consistent data flowing through systems aligned with business operations.
- What long-term strategic implications does establishing a robust, AI-ready data platform have for an organization's competitive advantage and adaptability in the evolving technological landscape?
- Organizations should integrate data governance into business units, embed data quality into performance management, and establish standardized data definitions. A balanced approach to data integration, using data mesh architectures and targeted derivative data products, maximizes benefits while managing risks. Prioritizing robust master data management and modern data infrastructure ensures a reliable AI foundation.
Cognitive Concepts
Framing Bias
The article frames the path to enterprise AI maturity as heavily reliant on establishing robust data platforms. This framing is consistent throughout, emphasizing the importance of data governance, strategic integration, and foundational investments. While not explicitly biased, this emphasis might downplay other contributing factors to AI success, such as talent acquisition or regulatory compliance.
Bias by Omission
The article focuses primarily on organizational and strategic aspects of building AI-ready data platforms, neglecting the technological details of specific AI models or algorithms. While this focus is understandable given the article's target audience, the omission of technical specifics might limit the understanding of readers with a strong technical background. There is no discussion of the various types of AI models and algorithms, or the technical challenges in implementing them.
Sustainable Development Goals
The article emphasizes the importance of building robust data platforms as a foundation for successful AI initiatives. This directly relates to SDG 9 (Industry, Innovation, and Infrastructure) by highlighting the need for modernizing infrastructure (data infrastructure in this case) to support innovation and technological advancement in the field of AI. Investing in data management, integration tools, and user experiences contributes to the development of a more efficient and effective technological infrastructure.