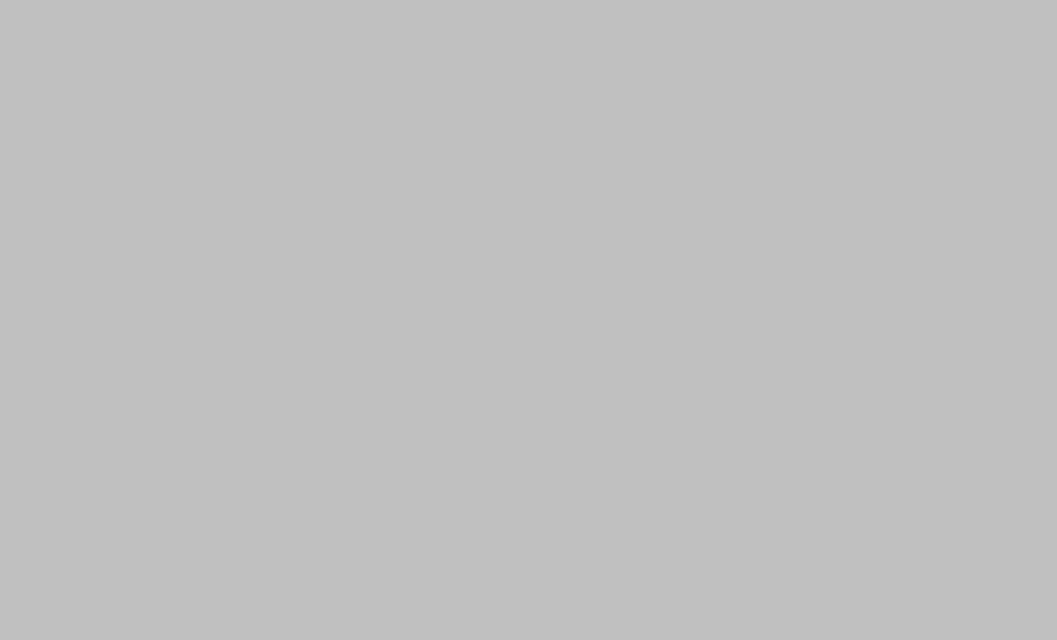
forbes.com
Experienced LLM Users Exceed AI in Detecting AI-Generated Text
A University of Maryland, Microsoft, and University of Massachusetts Amherst study found that experienced users of large language models (LLMs) can detect AI-generated text with 99.3% accuracy, rivaling the best AI detection software, such as Pangram, highlighting the potential for hybrid human-AI detection systems.
- What is the most significant finding of the study regarding human capabilities in detecting AI-generated text?
- A new study reveals that experienced users of large language models (LLMs) can effectively detect AI-generated text, even after it's been modified to evade automated detection systems. This finding challenges the prevalent assumption that humans are incapable of distinguishing between AI-written and human-written content. The accuracy rate of these experienced users approaches 99.3%, rivaling the best AI detection software.
- Considering the cost and time involved in using human reviewers, what practical applications and hybrid approaches could leverage their expertise while mitigating the drawbacks?
- The research highlights the potential for hybrid AI detection systems that combine automated tools with human review, especially in high-stakes situations like academic integrity or intellectual property disputes. While human review is more expensive and time-consuming ($2.82 per article and approximately a week for 60 articles), the ability to provide detailed explanations and achieve near-perfect accuracy makes it a valuable addition. This combined approach would enhance accuracy and offer a robust solution for detecting AI-generated content, safeguarding against misuse and upholding academic integrity.
- How does the accuracy of experienced human reviewers compare to that of the best AI detection software available, and what are the limitations of relying solely on either method?
- The study involved five human reviewers who frequently use LLMs. They evaluated human-written text, AI-generated text, and AI-generated text that had been edited to bypass AI detection tools. The human reviewers consistently outperformed most automated systems, demonstrating the potential for human expertise in this domain. This superior performance is particularly notable in identifying modified AI-generated text, which often confounds automated detectors.
Cognitive Concepts
Framing Bias
The framing emphasizes the surprising finding that experienced human reviewers can effectively detect AI-generated text, even outperforming some automated systems. This positive framing of human capabilities might overshadow the practical limitations and costs associated with employing human reviewers, which are also discussed. The headline 'people may not be useless after all' reflects this positive spin.
Language Bias
The language used is generally neutral and objective. However, phrases like "labor of human love" and "diluted every form of written communication" inject a somewhat emotional tone. While not overtly biased, these choices could subtly influence the reader's perception of the issue. More neutral alternatives could include 'human-created content' and 'affected written communication'.
Bias by Omission
The analysis focuses heavily on the accuracy of AI detection and the capabilities of human reviewers, potentially overlooking other relevant aspects of the issue, such as the ethical implications of AI-generated content or the impact on different sectors beyond academia. While the limitations of relying solely on AI detection systems are highlighted, a broader discussion of potential solutions beyond human review could strengthen the analysis. For instance, the article could discuss the development of more sophisticated AI detection methods or educational strategies to mitigate the use of AI in academic settings.
False Dichotomy
The article presents a somewhat false dichotomy by framing the debate as a choice between solely relying on automated AI detection systems or using expensive human reviewers. It overlooks the potential for hybrid approaches, combining automated systems with human review for specific cases, or exploring alternative solutions such as improved educational practices or the development of more robust AI detection techniques. This simplifies the complexity of the problem.
Sustainable Development Goals
The article discusses the widespread use of AI-generated text in academic settings, leading to concerns about the integrity of educational assessments and the devaluation of genuine student learning. AI-crafted homework and test answers are cited as a significant problem, undermining the meaning of earning an education. The research highlights the potential of experienced human reviewers to detect AI-generated text, offering a possible solution to this issue but acknowledges the cost and time constraints involved.