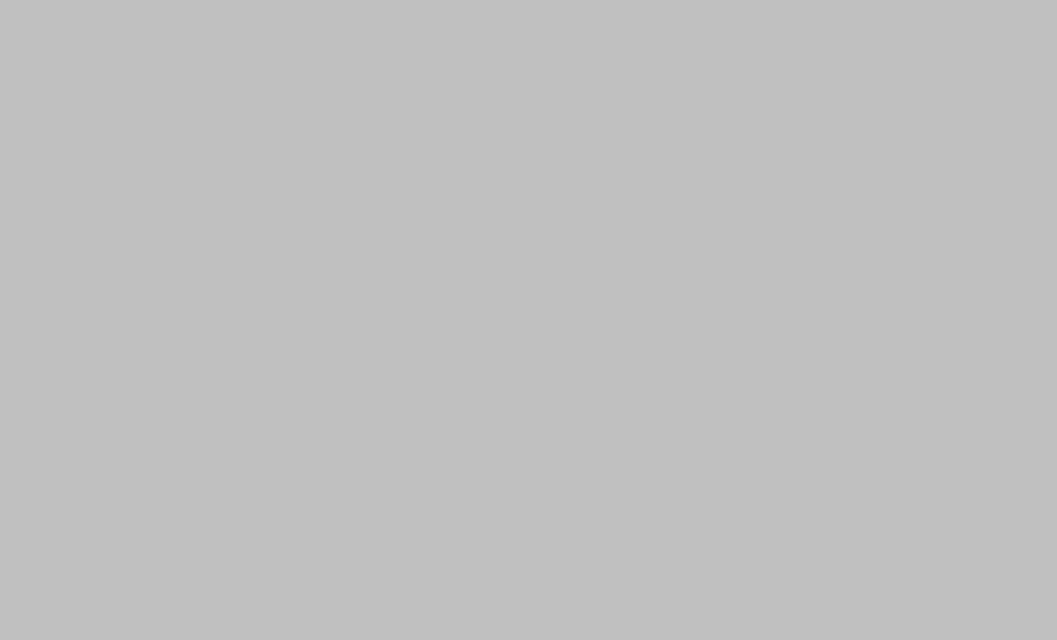
themarker.com
Flawed Automation Leads to Significant Errors in Israeli Tech Employment Data
Israel's Innovation Authority's automated system for gathering employment data from Facebook resulted in significant errors, including misclassifying a company with 20,000 employees as having 300 and listing a defunct company, demonstrating the dangers of relying solely on automated systems without manual verification.
- How did the lack of manual verification contribute to the errors, and what broader systemic issues does this highlight regarding quality control in automated data analysis processes?
- The incident reveals risks associated with relying solely on automated systems for data analysis, particularly when dealing with complex datasets. The lack of manual verification by the Innovation Authority compounded the problem, leading to substantially inaccurate conclusions about employment data in the Israeli tech sector.
- What are the immediate consequences of the flawed automation used by Israel's Innovation Authority to collect employment data, and what does this reveal about the potential pitfalls of relying solely on automated systems?
- An automated system used by Israel's Innovation Authority to collect employment data from Facebook produced significant errors, including misclassifying a company with 20,000 employees as having only 300 and including a company that had been closed for 25 years. Better code could have prevented some errors, highlighting the potential for inaccuracies in automated systems.
- What are the potential future implications of this incident, particularly in light of the growing reliance on automated systems and the increasing use of AI in data analysis, and what steps should be taken to mitigate the risks?
- This case underscores the urgent need for robust quality control measures when employing automation in data-driven tasks. The incident foreshadows potential challenges with increasingly common automated systems, especially given the rapid development of AI, and the importance of human oversight in preventing potentially significant errors.
Cognitive Concepts
Framing Bias
The narrative strongly frames the issues around political interference and potential abuse of power, emphasizing negative consequences of political decisions on businesses and academic institutions. The focus is predominantly on the actions and statements of Trump and the impact on various organizations, potentially overshadowing other important aspects of the discussed issues.
Language Bias
The text uses strong, opinionated language such as "טראמפ הורה לשלול לקרבס את הסיווג הביטחוני" (Trump ordered the revocation of Krebs' security clearance) and "טמבלים שמאלנים" (leftist idiots) which are loaded terms that reveal a bias against Trump's opponents and left-leaning perspectives. Neutral alternatives could focus on factual descriptions rather than subjective judgments. The overall tone is strongly critical of certain political figures and actions.
Bias by Omission
The provided text focuses heavily on political bias and the impact of political decisions on business and technology, potentially omitting other forms of bias present in the mentioned news articles or neglecting alternative viewpoints within the discussed issues. The analysis lacks a comprehensive examination of bias within each individual news article.
False Dichotomy
The text frequently presents false dichotomies, such as framing the debate around DEI initiatives as solely a matter of professional merit versus political correctness, overlooking nuanced arguments and potential benefits of DEI programs. Similarly, the discussion of Trump's actions presents a simplified 'loyalty vs. professionalism' dichotomy, ignoring the complexity of the situations and potential legal considerations.
Sustainable Development Goals
The article highlights how biases in automated systems and political pressures can negatively impact opportunities for underrepresented groups in the workforce and education. The examples of flawed automation in hiring, the suppression of DEI initiatives, and the politicization of hiring decisions all illustrate a widening gap in opportunities and a reinforcement of existing inequalities.