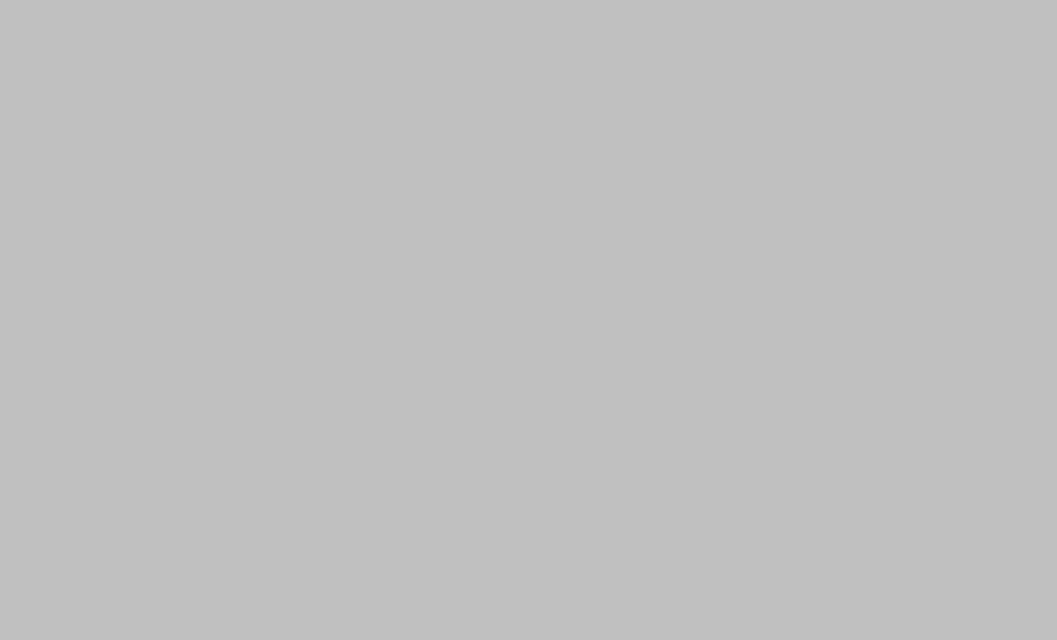
forbes.com
Green Computing Startups Tackle AI's Energy Footprint
Data centers consume 1% of global electricity and 0.6% of greenhouse emissions, projected to increase significantly by 2030; a new report highlights European startups developing GaN semiconductors and quantum computing to address this, though scaling up faces material supply and manufacturing challenges.
- What are the most impactful near-term solutions to mitigate the growing energy consumption of AI computing, considering both technological and geopolitical factors?
- The environmental impact of AI computing's energy demands is substantial, with data centers currently consuming 1% of global electricity and contributing 0.6% of greenhouse emissions, figures projected to rise significantly by 2030. New material innovations like Gallium Nitride (GaN) semiconductors offer efficiency gains up to 50% compared to silicon, potentially cutting global CO2 emissions by 2.6 gigatons annually by 2050. However, GaN's scalability is hampered by manufacturing limitations and supply chain issues, particularly gallium's concentrated production in China.
- How do material innovations, such as GaN semiconductors, and next-generation computing paradigms, like quantum computing, offer potential solutions to reduce AI's environmental footprint, and what are their limitations?
- The World Fund report highlights European startups developing alternative materials and computing paradigms to address AI's energy consumption. Funding in green computing is soaring, with 65 startups raising $1.5 billion. While solutions like GaN and quantum computing promise significant efficiency gains and emission reductions (up to 7 gigatons annually by 2035 for quantum computing), their widespread adoption faces technological and geopolitical hurdles, including material scarcity and supply chain complexities.
- What are the key geopolitical and technological hurdles that could hinder the widespread adoption of novel computing architectures and materials aimed at reducing AI's energy consumption, and what strategies can address these challenges?
- Despite promising innovations, the short-to-mid-term solution for AI's energy needs likely involves a combination of increased reliance on cleaner energy sources and incremental efficiency gains from existing technologies like GaN and SiC. Software optimizations, such as server virtualization, can also yield immediate, substantial energy savings. The long-term success hinges on overcoming the scalability challenges of next-generation technologies while simultaneously accelerating the transition towards cleaner energy sources.
Cognitive Concepts
Framing Bias
The article frames the problem as a technological challenge, emphasizing the potential of innovative materials and computing paradigms. While acknowledging limitations, the positive potential of these solutions is highlighted more prominently than the challenges and uncertainties involved.
Language Bias
The language used is largely neutral, although phrases like "frantic pace of development" and "rushing advances in artificial intelligence" might subtly suggest a negative connotation associated with the rapid growth of AI.
Bias by Omission
The article focuses primarily on technological solutions and omits discussion of policy interventions (e.g., carbon taxes, regulations) that could play a significant role in mitigating the environmental impact of data centers. It also doesn't delve into the social and economic implications of transitioning to new technologies.
False Dichotomy
The article presents a false dichotomy by framing the choice as solely between relying on fossil fuels and adopting entirely new technologies, neglecting the possibility of incremental improvements and a combination of approaches.
Sustainable Development Goals
The article highlights the environmental impact of data centers and explores potential solutions like GaN semiconductors and quantum computing to reduce energy consumption and greenhouse gas emissions. These innovations, if successfully implemented at scale, could significantly mitigate the carbon footprint of AI computing and contribute to climate change mitigation goals. The text also mentions the potential for significant emission reductions through the development of next-generation high-performance batteries. The urgency is underscored by the rapid growth of AI-related energy demands.