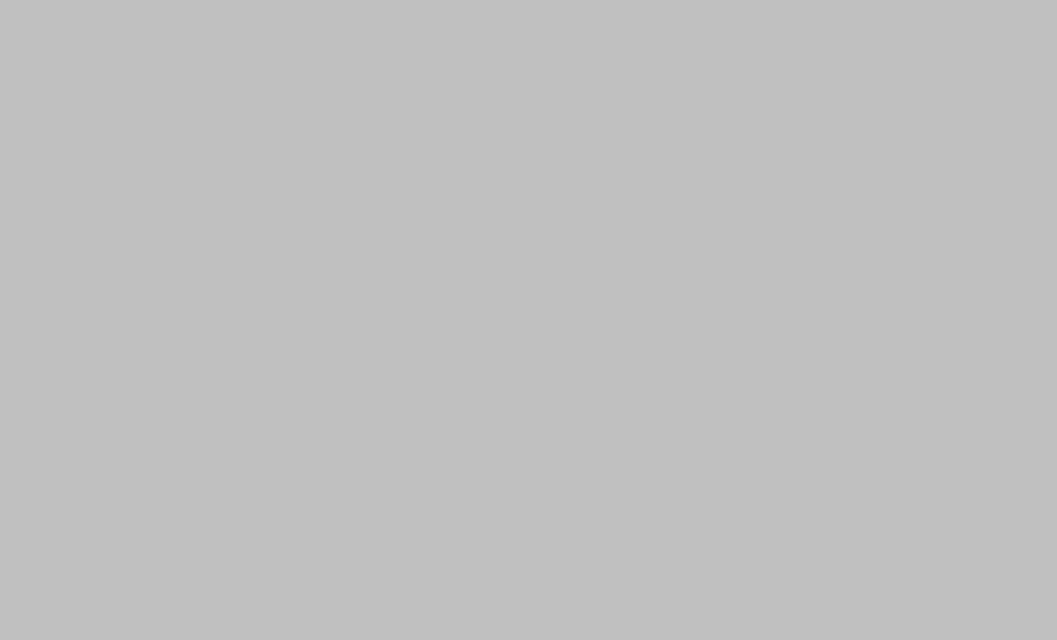
forbes.com
Human Data: The Key to Smarter AI in Business
AI's success hinges on high-quality data; recognition data—insights from employee appreciation—provides a richer, more authentic understanding of workplace dynamics than traditional methods, enabling AI to reveal hidden contributions and predict flight risks, ultimately transforming HR's strategic role.
- What is the primary limitation of current AI applications in business, and how does recognition data address this limitation?
- AI's effectiveness is directly tied to data quality; flawed data amplifies biases and limits AI's potential, as evidenced by the 80% failure rate of AI projects according to a Rand Corporation study. Using recognition data—insights from employee appreciation—offers a more nuanced understanding of workplace dynamics than traditional metrics like turnover rates.
- How does recognition data differ from traditional HR metrics, and what specific insights does it provide that traditional methods lack?
- Recognition data, derived from expressions of gratitude between employees, reveals the value and impact of work, unlike traditional performance reviews which focus solely on skills and objectives. This richer data allows AI to identify hidden contributors and predict flight risks, illustrated by the example of an AI identifying the CEO as a potential flight risk due to low recognition.
- What are the long-term implications of using AI and recognition data to analyze human interactions in the workplace, and how might this reshape the role of HR?
- Aggregating recognition data across numerous companies creates a massive dataset revealing best practices and quantifiable returns on HR investments. This allows HR to move from intuition to insight, becoming strategic partners in shaping business outcomes and driving sustainable growth, transforming the role of HR in the C-suite. The future of successful organizations will depend on effectively using human intelligence to augment human data.
Cognitive Concepts
Framing Bias
The framing is overwhelmingly positive towards recognition data and its use with AI. The potential downsides are minimized, and the narrative strongly promotes the benefits without adequately addressing potential concerns. The headline itself, "The Path to Smarter AI Starts with Human Data," sets a strongly positive and potentially biased frame.
Language Bias
The language used is generally positive and enthusiastic, employing phrases such as "treasure trove of insights," "giant leap forward," and "rocket fuel." While this enthusiastic tone is understandable given the topic, it contributes to the overall positive framing and may subtly influence the reader's perception. More neutral language could be used to present the information more objectively.
Bias by Omission
The article focuses heavily on the benefits of recognition data and AI, potentially omitting critical counterarguments or limitations. It doesn't discuss potential biases in recognition data itself (e.g., some employees might be more likely to receive recognition than others, regardless of performance) or the ethical implications of using AI to analyze employee interactions. The potential for misuse or misinterpretation of the data is not explored.
False Dichotomy
The article presents a somewhat false dichotomy between traditional HR data and recognition data, implying that only recognition data provides a true understanding of workplace dynamics. It oversimplifies the value of other types of data and fails to acknowledge that a holistic approach combining various data sources would likely provide a more complete picture.
Sustainable Development Goals
The article highlights how biases in data used to train AI systems can perpetuate societal inequalities. By focusing on human data, specifically recognition data, the approach aims to mitigate these biases and promote more equitable outcomes in the workplace. Recognition data, by its nature, is less susceptible to systemic biases present in traditional performance metrics, leading to a more inclusive assessment of employee contributions and value.