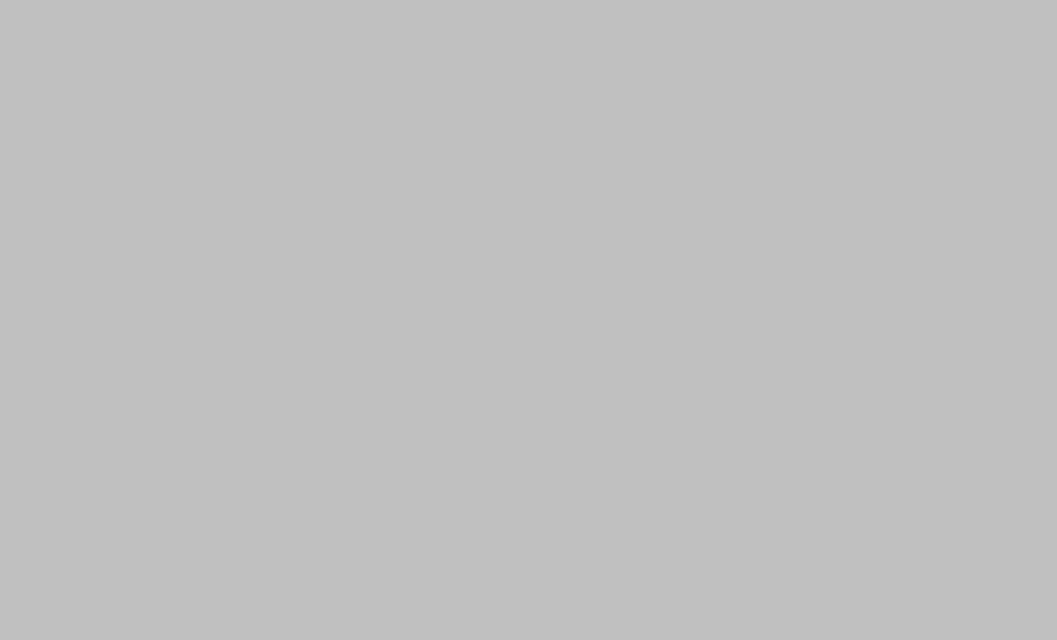
forbes.com
Industrial Manufacturing's Slow but Insightful AI Adoption
Despite a head start from Industry 4.0, only 16% of industrial manufacturing businesses have integrated AI, compared to 25% across all industries; however, their experience offers valuable lessons for other sectors navigating AI adoption challenges, emphasizing data quality, system integration, and a phased approach to scaling AI.
- What factors contribute to the relatively slow AI adoption rate in industrial manufacturing despite its existing digital infrastructure?
- While industrial manufacturing has a head start in AI adoption due to prior digital transformation (Industry 4.0), only 16% of businesses have integrated AI, compared to 25% across all industries. This suggests a slower-than-expected pace, possibly due to transformation fatigue or a cautious approach.
- What are the potential long-term implications of achieving AI autonomy in industrial manufacturing, and what broader economic or societal effects might result?
- The industrial manufacturing sector's AI journey progresses through automation, process transformation, and ultimately, autonomy. While most industries are in the initial stages, manufacturers demonstrate that autonomy is achievable with sustained investment in AI scaling, leading to improved efficiency, decision-making, and customer value.
- How does the experience of industrial manufacturers in overcoming challenges during Industry 4.0 inform their approach to AI integration, and what lessons can other sectors learn?
- Manufacturers' experience with Industry 4.0 provides valuable lessons for other sectors. Their expertise in data integration and handling legacy systems helps them navigate AI implementation challenges more effectively, emphasizing the importance of data quality and system integration over complete overhauls. This is a critical advantage compared to sectors without such experience.
Cognitive Concepts
Framing Bias
The article frames the manufacturing sector's AI adoption journey as a success story and a valuable blueprint for other industries. This positive framing, while warranted by the data presented, might downplay the difficulties faced by manufacturers and inadvertently set unrealistic expectations for other sectors. The headline itself emphasizes the manufacturing sector's head start, potentially overshadowing the challenges involved in AI implementation across all industries.
Language Bias
The language used is generally neutral and objective. However, phrases like "game-changing results" and "clear edge" are slightly promotional and could be replaced with more neutral alternatives. For example, "significant improvements" instead of "game-changing results" and "advantage" instead of "clear edge.
Bias by Omission
The article focuses heavily on the manufacturing sector's experience with AI adoption, potentially neglecting the unique challenges and successes of other industries. While it mentions that data silos, disconnected systems, and workforce readiness are universal barriers, it doesn't delve into the specifics of how these barriers manifest differently across sectors. This omission could limit the article's applicability and usefulness to readers outside the manufacturing industry.
False Dichotomy
The article doesn't present a false dichotomy, but it could benefit from acknowledging a wider range of approaches to AI adoption beyond the integration-focused strategy highlighted in the manufacturing context. While integration is presented as a practical solution, it may not be the optimal path for all businesses.
Sustainable Development Goals
The article highlights the advancements in AI adoption within industrial manufacturing, showcasing how manufacturers are leveraging AI for predictive maintenance, quality assurance, and energy management. These applications directly contribute to increased efficiency, improved sustainability, and economic growth, all of which are key aspects of SDG 9 (Industry, Innovation and Infrastructure). The experience gained by manufacturers in integrating AI can serve as a valuable blueprint for other sectors, further accelerating innovation and infrastructure development globally.