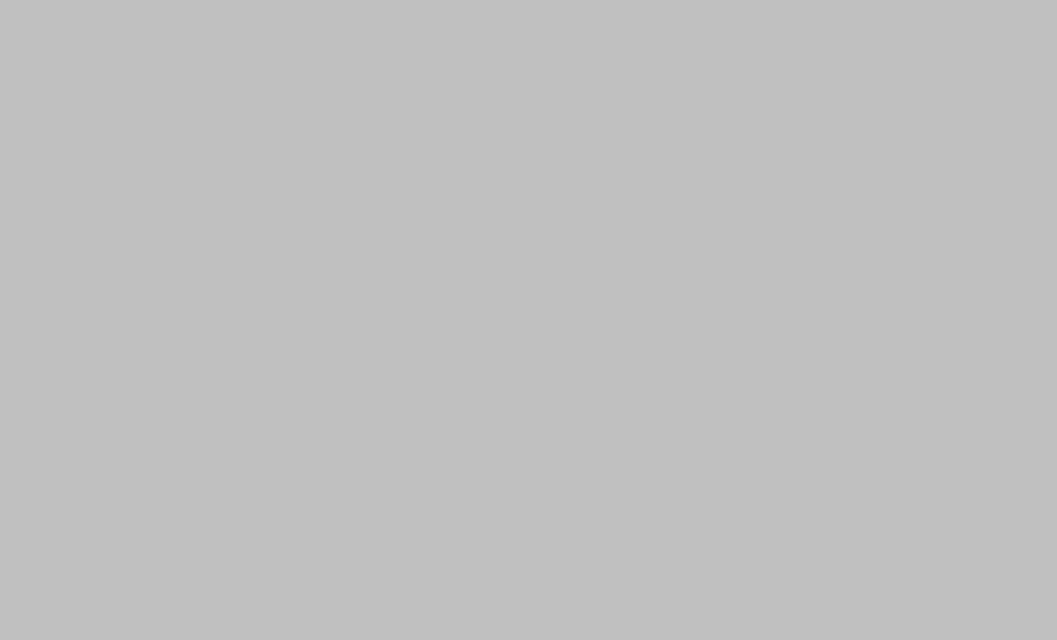
t24.com.tr
Large Language Model Hallucinations: Causes, Consequences, and Mitigation Strategies
Large language models, while convenient, frequently produce hallucinations—fabricating information or contradicting input. This issue manifests in factual errors and context inconsistencies, affecting reliability and highlighting the need for user verification. A study revealed error rates of 30-90% in academic references generated by these models.
- What are the primary types of hallucinations exhibited by large language models, and what are their potential consequences?
- Large language models like ChatGPT and DeepSeek offer quick answers, but they are prone to hallucinations—generating fabricated or inconsistent content. This can manifest as factual inaccuracies, such as incorrectly stating the UEFA Cup winner, or inconsistencies like altering dates in a news report.
- How do the inherent limitations of large language models, such as information loss during data processing, contribute to the generation of hallucinations?
- These hallucinations are categorized into 'factual' and 'coherence' types. Factual hallucinations contradict verifiable facts, while coherence hallucinations deviate from user instructions or context. A study revealed that these models produce erroneous references in academic papers, with error rates between 30% and 90%.
- What practical strategies, beyond increasing model parameters, can mitigate the risk of hallucination in large language models, while considering limitations in computation and data access?
- Addressing this issue is complex. While increasing model parameters and training duration can reduce hallucinations, the computational cost is substantial. Retrieval Augmented Generation (RAG) offers another approach, using external sources but faces limitations due to accessibility and computational costs. User verification remains crucial for accuracy.
Cognitive Concepts
Framing Bias
The framing is largely negative, emphasizing the dangers and unreliability of AI hallucinations. The headline uses the term "yalancılar" (liars), setting a critical tone from the outset. While the article later uses the more neutral term "hallucination," the initial framing could unduly influence the reader's perception.
Language Bias
The language used is generally objective, but some terms could be considered loaded. For instance, referring to AI as "yalancılar" (liars) is emotionally charged. Using more neutral terms like "inaccurate" or "producing fabricated information" would improve objectivity. The repeated use of "yalancılar" and similar terms reinforces the negative framing.
Bias by Omission
The article focuses primarily on the problem of AI hallucinations, offering solutions and examples. However, it omits discussion of potential benefits or alternative perspectives on the issue, such as the potential for AI to improve fact-checking or other applications that mitigate the risks of hallucination. The omission of these perspectives might lead to a skewed understanding of the overall impact of AI.
False Dichotomy
The article presents a somewhat false dichotomy by focusing heavily on the limitations of AI while not fully exploring the potential solutions and ongoing research to improve AI accuracy. While acknowledging that hallucinations cannot be completely eliminated, it could benefit from a more balanced representation of the ongoing efforts to mitigate the issue.
Sustainable Development Goals
The article discusses the issue of AI hallucinations, where large language models generate false information. This impacts the reliability of information available for educational purposes, potentially hindering quality education and the ability to access accurate knowledge. The examples provided illustrate how these models can produce incorrect information, even in academic contexts, leading to the spread of misinformation in education and research.