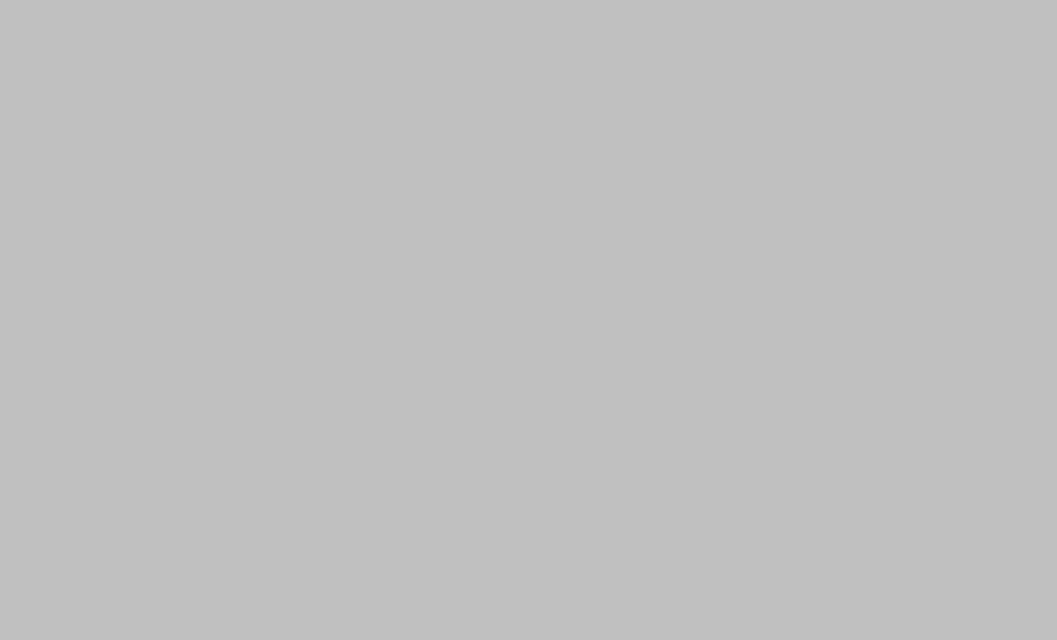
forbes.com
Large Language Models Fall Short in Analogical Reasoning
A new study reveals that large language models significantly underperform humans in analogical reasoning, highlighting a critical limitation in their ability to generalize from diverse data and affecting their applicability in various fields, unlike human adaptability to unfamiliar contexts.
- What are the underlying reasons for the disparity between human and AI performance in analogical reasoning tasks?
- The inability of large language models to effectively reason by analogy stems from their dependence on training data; they struggle to generalize to unfamiliar contexts. This contrasts sharply with human cognitive abilities, which allow for effective reasoning even with limited prior knowledge. This has implications for AI's applicability in diverse and unpredictable scenarios.
- How do the limitations of large language models in analogical reasoning affect their application in fields like science and law?
- Large language models like GPT-3 and GPT-4 underperform humans in analogical reasoning, significantly hindering their ability to generalize from diverse situations, as shown in a recent study. This limitation affects various fields, including science and law, where analogical reasoning is crucial. The study also reveals that model performance is heavily reliant on training data similarity, unlike human adaptability.
- What approaches can be adopted to enhance the analogical reasoning capabilities of large language models, improving their generalization ability and real-world applicability?
- Future AI development must address the limitations in analogical reasoning to enhance the adaptability and robustness of these models. Focusing on improving generalization capabilities beyond the scope of training data is crucial for broader real-world applications. This may involve exploring new architectural designs or training methodologies.
Cognitive Concepts
Framing Bias
The article presents a generally positive framing of technological advancements, emphasizing the potential benefits of brain-computer interfaces and new vaccines while downplaying or omitting potential risks or drawbacks. The headline structure also prioritizes intriguing advancements over cautionary notes.
Language Bias
The language used is largely neutral and descriptive, avoiding overly sensationalized or emotionally charged terms. However, phrases like "hack your brain, but in a good way" might be considered slightly informal and promotional, potentially influencing reader perception.
Bias by Omission
The article focuses heavily on technological advancements and omits discussions of the ethical implications of brain-computer interfaces or the potential societal impact of widespread adoption of such technologies. Additionally, the section on the salmonella vaccine lacks details on potential side effects or limitations of the research, which could be considered an omission.
False Dichotomy
The article presents a false dichotomy by framing brain-computer interfaces as having only two options: invasive implants or non-invasive methods with limitations, neglecting potentially alternative approaches.
Sustainable Development Goals
The article discusses advancements in medical technology with the potential to improve human health. The development of nanoparticles to connect brains to computers offers a potential treatment for neurological diseases and improved coordination with prosthetic limbs. A new vaccine approach for food poisoning shows promise in combating gut bacteria. Additionally, a new blood test for Alzheimer's disease aids in diagnosis and disease progression assessment.