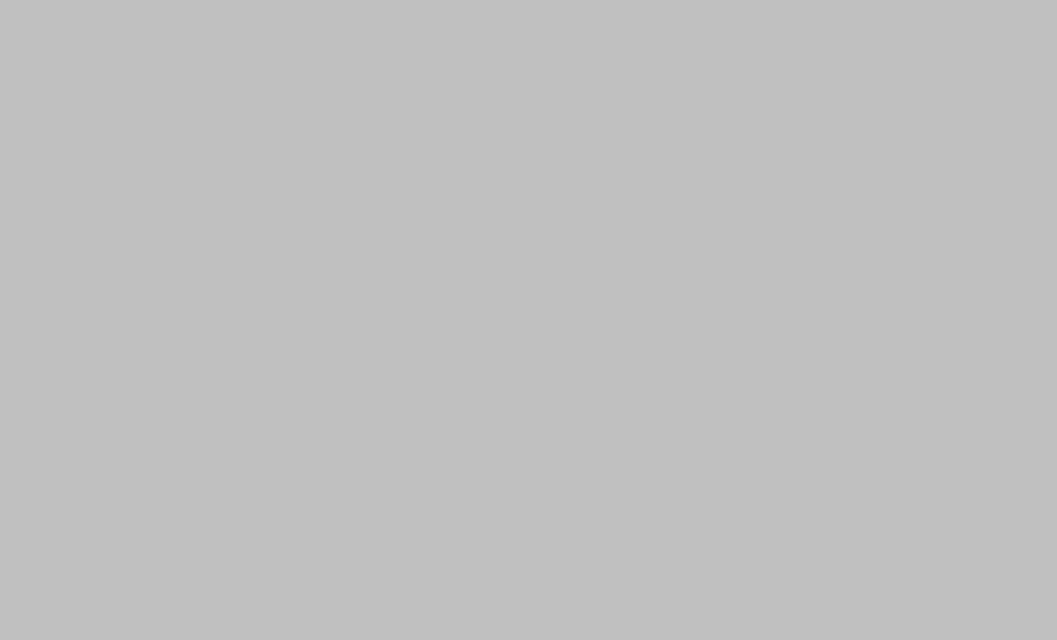
forbes.com
Lichtman's Simple Model Accurately Predicts Trump's 2024 Victory
Allan Lichtman's 13-factor system accurately predicted Donald Trump's 2024 presidential victory, highlighting the importance of key factors like voter perception of the economy and candidate charisma over complex polling data, despite some past inaccuracies.
- How did Lichtman's model's minor inaccuracies in 2000, 2016, and 2020 inform its improved prediction in 2024, and what adjustments were key to this improvement?
- Lichtman's model, while occasionally faltering (2000, 2016, 2020), accurately predicted Trump's win by assessing factors like economic sentiment (voter perception of real wages, not just recession), and candidate charisma. These factors, often overlooked in complex polling, proved pivotal in determining the outcome.
- What key factors, overlooked by traditional polling methods, accurately predicted Donald Trump's 2024 victory, and what do these factors reveal about the limitations of complex election forecasting?
- Allan Lichtman's 13-factor system, a "fast and frugal" heuristic, correctly predicted Donald Trump's 2024 presidential victory, highlighting the system's accuracy despite minor past inaccuracies. The system's success underscores the importance of identifying key factors, even with simple models, over complex analyses that may overlook crucial voter sentiments.
- What broader implications does the success of Lichtman's "fast and frugal" heuristic have for future election forecasting, particularly regarding the integration of voter psychology and economic indicators?
- The 2024 election demonstrates that simple predictive models, focusing on key factors and voter sentiment, can outperform complex analyses. Lichtman's near-perfect track record, even with minor adjustments needed, highlights the importance of understanding voter psychology and incorporating nuanced economic indicators reflecting real-world impact on voters.
Cognitive Concepts
Framing Bias
The article frames Lichtman's model as overwhelmingly successful, highlighting its accuracy while downplaying the instances where it failed. The emphasis on the model's predictive power might lead readers to overestimate its reliability and overlook other influential factors. The headline itself, implying foreknowledge of Trump's victory, biases the narrative before the reader begins.
Language Bias
The article uses language that subtly favors Lichtman's model. Words such as "predictable," "almost perfect," and "clear" create a positive impression, while descriptions of the model's failures are less emphatic. The author's strong endorsement of the "fast and frugal" approach to forecasting could also be seen as a form of loaded language. More neutral language might involve replacing words such as "clear" with "apparent," and using less evaluative language in describing the model's accuracy.
Bias by Omission
The analysis focuses heavily on Lichtman's model and its successes and failures, neglecting other factors that might have influenced the election outcome. While the author mentions voter sentiment and economic conditions, a deeper exploration of other relevant factors (e.g., social media influence, campaign strategies) is absent. The omission of these factors limits a comprehensive understanding of the election's outcome, potentially misleading the reader into overemphasizing Lichtman's model's predictive power.
False Dichotomy
The article presents a false dichotomy by implying that only Lichtman's model or complex models can predict election outcomes, ignoring the possibility of other methods and combinations of approaches.
Sustainable Development Goals
The article highlights how economic factors, specifically inflation and declining real wages, disproportionately affected working-class and Latino voters. This led to a significant portion of these groups supporting Trump, exacerbating existing inequalities and hindering progress towards reducing income inequality. The failure to accurately gauge voter sentiment on the economy, due to biases in political analysis, further contributed to this negative impact on the SDG.