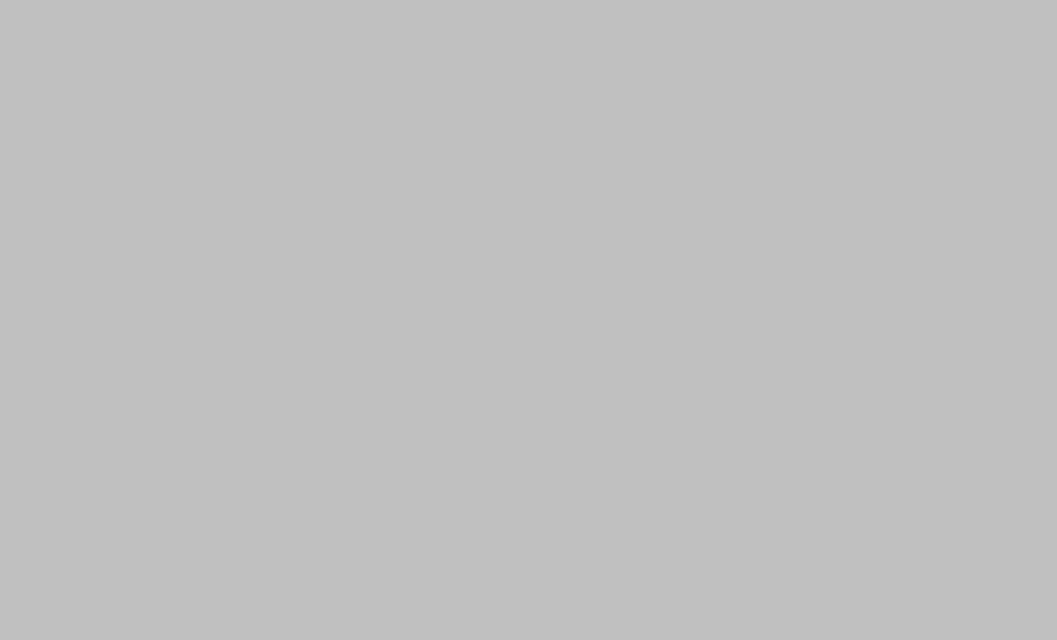
forbes.com
Multimodal AI Image Generation: A Paradigm Shift
New AI image generation models use multimodal control, integrating text prompt generation and image creation into a single system, resulting in more coherent and contextually aware images unlike previous diffusion models that generated images based solely on training data.
- What are the long-term implications of multimodal AI image generation on various professions and creative fields?
- The shift to multimodal image generation signifies a paradigm shift in AI capabilities. These models exhibit a deeper understanding of context and can reason about image content, potentially automating complex tasks like creating presentations or marketing materials based on a simple textual description, with implications for many professions.
- What challenges did traditional image generation models present, and how do multimodal models overcome these limitations?
- Multimodal models offer superior control and understanding. Unlike older diffusion models that simply added noise and denoised images based on training data, these new models can interpret complex prompts, such as requests to exclude elements or preserve specific features while altering others, resulting in more accurate and nuanced image generation.
- How do new multimodal AI image generation models differ from previous methods, and what are the immediate practical implications?
- New AI image generation models are fundamentally different from their predecessors. Previously, AI generated images by sending text prompts to separate image generation tools; now, multimodal models integrate these processes, leading to more coherent and contextually aware results.
Cognitive Concepts
Framing Bias
The article frames the new multimodal AI image generation models very positively, highlighting their capabilities and potential benefits while downplaying any limitations or challenges. The headline and introductory paragraphs emphasize the revolutionary nature of these models, setting a positive tone that might overshadow potential drawbacks.
Language Bias
The language used is generally neutral, but terms like "smarter pictures" and "revolutionary" carry positive connotations and may subtly influence reader perception. The phrase "obsolete very quickly" presents a rather strong and potentially biased assertion.
Bias by Omission
The article focuses primarily on the advancements in AI image generation, neglecting potential downsides or ethical concerns. There is no discussion of the environmental impact of training these large models, or the potential for misuse in creating deepfakes or other forms of misinformation. This omission limits the reader's ability to form a fully informed opinion.
False Dichotomy
The article presents a dichotomy between 'traditional' and 'multimodal' image generation models, implying a clear-cut superiority of the latter. However, it overlooks nuances and potential future developments that could blur this distinction.
Sustainable Development Goals
The development of multimodal image generation models represents a significant advancement in AI and image processing. These models have the potential to revolutionize various industries by enabling more efficient and effective content creation, design, and communication. This aligns with SDG 9 which promotes building resilient infrastructure, promoting inclusive and sustainable industrialization and fostering innovation.