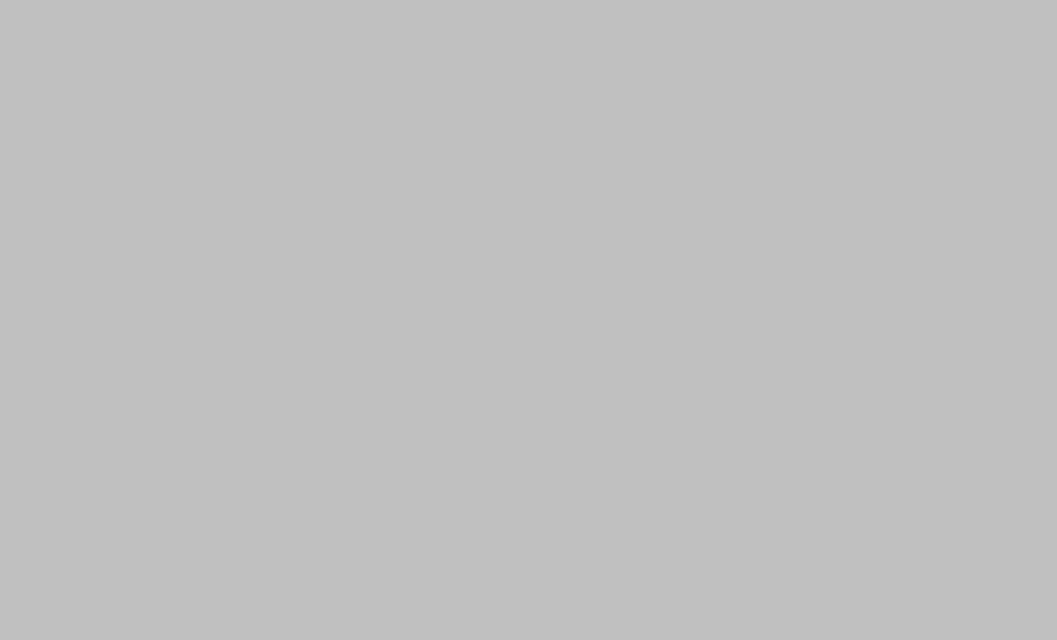
theguardian.com
Musk: AI Has Exhausted Human Knowledge, Turning to Synthetic Data
Elon Musk asserts that AI companies have exhausted the sum of human knowledge for training models, necessitating a shift to synthetic data, a process already underway, but raising concerns about accuracy and the risk of 'model collapse'.
- How does the increasing reliance on synthetic data affect the accuracy and potential biases of AI models?
- Musk's assertion connects the finite nature of readily available data with the increasing reliance on AI-generated content. The transition to synthetic data, while necessary, introduces challenges related to accuracy and the risk of 'hallucinations'—inaccurate outputs from AI models. This necessitates careful validation and quality control methods.
- What are the immediate implications of AI companies exhausting publicly available data for training AI models?
- Elon Musk claims AI companies have exhausted the sum of human knowledge for training models, necessitating a shift to synthetic data. This shift is already underway, with companies like Meta and Microsoft using synthetic data for model fine-tuning. The consequence is a potential for model collapse.
- What are the long-term risks and challenges associated with the widespread use of synthetic data for training AI, and how can they be mitigated?
- The over-reliance on synthetic data for AI model training poses a significant risk of model collapse, leading to diminished returns and biased outputs. The increasing presence of AI-generated content online further compounds this issue, creating a feedback loop where AI models train on increasingly artificial data. This could significantly hinder the future development and reliability of AI models.
Cognitive Concepts
Framing Bias
The framing centers heavily on Elon Musk's statements and concerns about the limitations of real-world data and the risks of synthetic data. While other companies' use of synthetic data is mentioned, the narrative's emphasis is on Musk's perspective and the potential downsides. The headline, while not explicitly biased, could benefit from a more neutral phrasing to reflect the broader implications of the issue. This framing may lead readers to overemphasize the negative aspects of synthetic data without a balanced view of its potential benefits.
Language Bias
The language used is largely neutral, employing objective terms and direct quotes. However, terms like "exhausted" and "hallucinations" when discussing AI data and output are subjective and may influence reader perception. More neutral wording such as "depleted" and "inaccurate outputs" could be considered.
Bias by Omission
The article focuses primarily on Elon Musk's claims and the potential risks of synthetic data. It mentions other companies using synthetic data but doesn't delve into the specifics of their approaches or the scale of their usage. The perspectives of AI researchers beyond Andrew Duncan are absent, potentially limiting a comprehensive understanding of the issue. The article could benefit from including diverse viewpoints on the potential benefits and challenges of synthetic data, as well as exploring alternative solutions to the data exhaustion problem. However, given the article's length and focus, the omissions may be unintentional rather than a deliberate bias.
False Dichotomy
The article presents a somewhat simplistic eitheor scenario: either we use synthetic data, or we run out of training data for AI models. It doesn't adequately explore the potential for alternative solutions, such as improved data curation, more efficient data usage techniques, or focusing on different types of data. This simplification could misrepresent the complexity of the problem and limit the reader's understanding of possible solutions.
Sustainable Development Goals
The exhaustion of real-world data for AI training and the increased reliance on synthetic data raise concerns about the quality and accuracy of information used in educational settings. AI-generated content, if inaccurate or biased, could negatively impact the learning process and perpetuate misinformation. The potential for "model collapse" further exacerbates this concern, suggesting a decline in the quality of AI-generated educational resources.