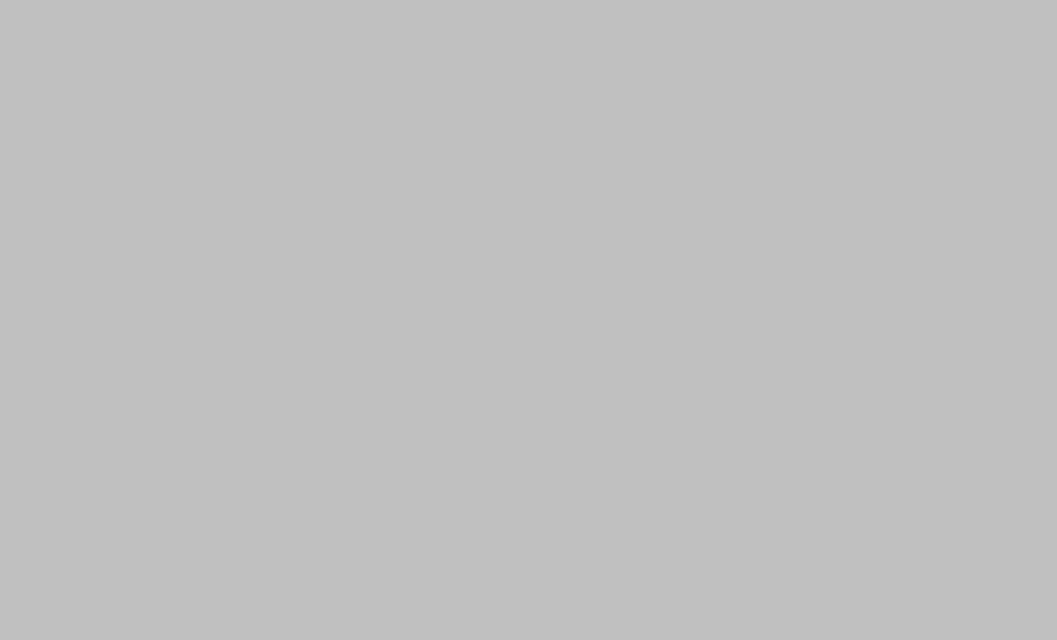
themarker.com
New AI Test Reveals Advanced Models' Significant Shortcomings
A new AI benchmark, the "Last Human Test," consisting of 3,000 complex questions in fields like philosophy and engineering, reveals that leading AI models achieve only 8.3% accuracy, questioning current methods of assessing AI intelligence.
- How was the "Last Human Test" designed, and what specific knowledge domains are evaluated to assess AI capabilities?
- The "Last Human Test" comprises 3,000 multiple-choice and short-answer questions crafted by experts in fields like philosophy and engineering. The test's difficulty stems from requiring advanced knowledge and nuanced understanding exceeding typical benchmarks. This approach aims to gauge AI's potential for automating complex intellectual tasks.
- What are the initial results of the "Last Human Test," and what does this indicate about the current capacity of advanced AI models?
- Researchers have developed a new benchmark, the "Last Human Test," to evaluate advanced AI models' ability to answer complex questions across various academic fields. Initial results show AI models performing poorly, with the best scoring only 8.3%. This highlights the challenge in measuring AI capabilities beyond standard tests.
- Given the limitations of current AI evaluation methods, what alternative approaches might better capture the actual progress and impact of advanced AI systems?
- The significant underperformance of leading AI models on the "Last Human Test" suggests current methods are inadequate for assessing genuine intelligence. Future evaluation might shift from standardized tests to analyzing economic impacts or counting breakthroughs in fields like math and science. This reflects a broader need for creative methods to track AI progress beyond test scores.
Cognitive Concepts
Framing Bias
The narrative emphasizes the difficulty of creating tests that AI cannot pass, framing AI's progress as a race against human ingenuity in test creation. This framing highlights the potential threat of advanced AI, potentially overlooking the many beneficial applications of AI and underplaying the ongoing efforts to develop safe and beneficial AI systems.
Language Bias
The language used is generally neutral and objective. However, phrases like "Last Human Test" and "AI becoming too smart to measure" are somewhat dramatic and might evoke a sense of alarm or concern in the reader, without adequately balancing these concerns with more positive perspectives of AI development.
Bias by Omission
The article focuses heavily on the new "Last Human Test" and its creators, potentially omitting discussion of other existing AI assessment methods and their limitations. While mentioning other tests like Frontier-Math and AGI-ARC, it doesn't delve into their strengths or weaknesses in comparison. This omission might give a skewed perspective on the overall progress and challenges in AI assessment.
False Dichotomy
The article presents a false dichotomy between AI surpassing human capabilities and the inability to measure AI intelligence. It implies that if AI can't be reliably tested, it must be too intelligent to measure. However, other factors such as the limitations of current testing methodologies or the multifaceted nature of intelligence are not fully explored.
Gender Bias
The article predominantly features male researchers and experts, including Dan Hendrycks, Kevin Zhou, and Elon Musk. While this likely reflects the current demographics of the field, it could benefit from including more female voices and perspectives to offer a more balanced representation.
Sustainable Development Goals
The article discusses the development of a new, more challenging benchmark for AI systems, pushing the boundaries of what AI can achieve. This indirectly relates to Quality Education by highlighting the advanced knowledge required to create and answer the questions in the test, which are at a postgraduate and doctoral level. The creation of these tests and the AI