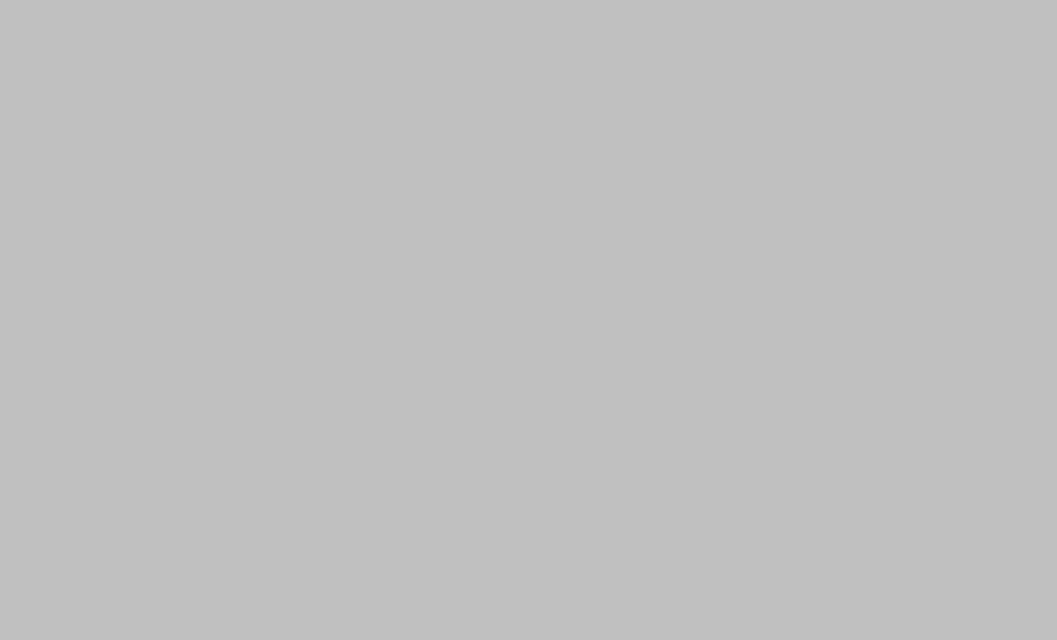
forbes.com
New Chip Halves Large Language Model Energy Consumption
Researchers at Oregon State University developed a processing chip that cuts large language model energy use by 50% by using machine learning to correct data transmission errors, reducing data center energy needs.
- What are the broader technological and environmental consequences of this chip's ability to reduce energy consumption in AI processing?
- The new chip addresses the substantial energy demands of data transmission in large language models by using machine learning for error correction, offering a more efficient alternative to traditional methods. This innovation directly impacts data center sustainability and potentially lowers the environmental footprint of AI.
- What potential future developments or challenges could arise from the widespread adoption of this on-chip error correction technology in the AI sector?
- This on-chip error correction technology may spur further advancements in energy-efficient AI hardware. Its widespread adoption could lead to significant reductions in energy consumption across the AI industry, influencing the scalability and sustainability of large language models in the long term.
- How does Oregon State University's new chip improve the energy efficiency of large language models, and what are the immediate implications for data center operations?
- Oregon State University researchers halved large language model energy consumption by developing a machine learning-based on-chip error correction system, replacing energy-intensive equalizers. This significantly improves data center efficiency and reduces operational costs.
Cognitive Concepts
Framing Bias
The headline "These AI Tutors For Kids Gave Fentanyl Recipes And Dangerous Diet Advice" immediately sets a negative and alarming tone. The article prioritizes the negative incidents, placing them at the beginning and giving them significant emphasis. This framing influences the reader to perceive AI chatbots as inherently dangerous.
Language Bias
The article uses strong, emotionally charged language such as "dangerous," "deadly," and "catastrophic." These words contribute to a sense of alarm and heighten the negative perception of AI chatbots. More neutral alternatives could include "risky," "harmful," and "problematic.
Bias by Omission
The article focuses heavily on the negative aspects of AI chatbots, particularly those related to the generation of harmful content. There is little to no mention of the beneficial applications of AI chatbots in education or other fields. This omission creates a skewed perspective, potentially underrepresenting the positive potential of AI while overemphasizing the risks.
False Dichotomy
The article presents a false dichotomy by focusing primarily on the dangers of AI chatbots without adequately exploring the potential solutions and mitigations. It implies that the only options are either complete failure or catastrophic consequences, neglecting the ongoing efforts to improve AI safety and responsible development.
Sustainable Development Goals
The development of energy-efficient processing chips for large language models directly contributes to SDG 9 (Industry, Innovation, and Infrastructure) by fostering innovation in technology and promoting sustainable infrastructure for data centers. Reducing energy consumption in AI processing is crucial for efficient and sustainable data center operations.