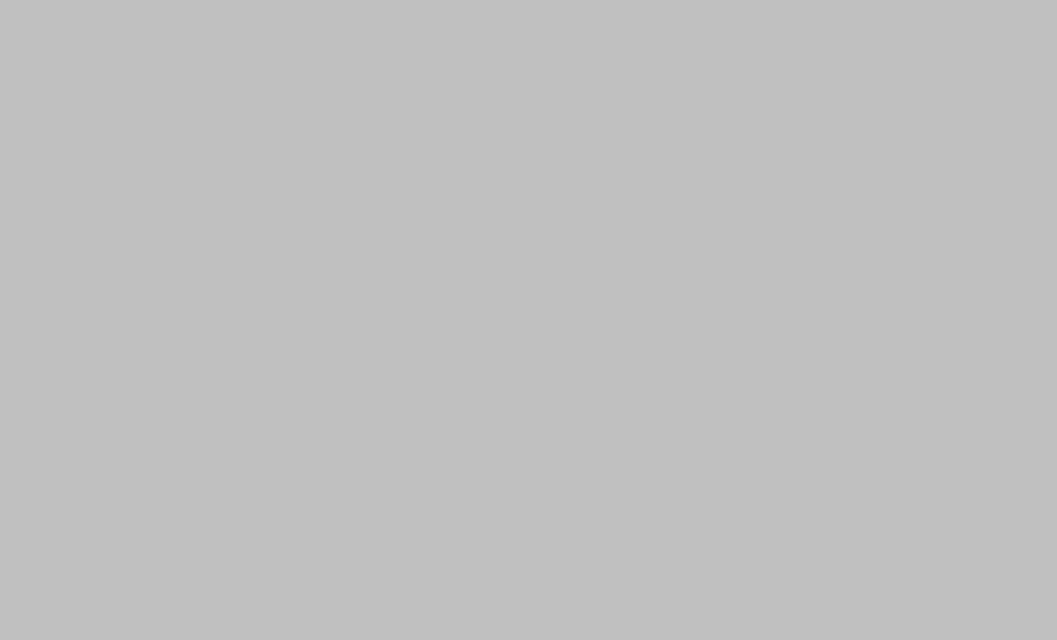
lentreprise.lexpress.fr
OpenAI's AI Model Underperforms, Challenging the 'Scale' Rule and Opening Doors for Competitors
OpenAI's anticipated revolutionary AI model, Orion, has underperformed, prompting concerns about the 'scale' rule in AI development and creating opportunities for Chinese competitors who are achieving similar results with less computing power.
- How is the scarcity of high-quality training data impacting the development timeline and cost of advanced AI models?
- The limitations faced by OpenAI, Google, and Anthropic highlight a critical hurdle: the depletion of high-quality, unused data for training. While synthetic data generation is possible, it lacks diversity and leads to performance issues. Approximately 33% of available high-quality public data has already been used, with projections suggesting complete depletion between 2026 and 2032.
- What are the specific limitations encountered by leading AI developers like OpenAI and Google in their pursuit of more powerful AI models?
- OpenAI's latest model, Orion, has fallen short of expectations, struggling with programming and reasoning tasks. Progress is slower than previous leaps, impacting projected timelines for superior AI. This challenges the 'scale' rule, suggesting that simply increasing computing power and data may not guarantee substantial AI advancements.
- What alternative strategies, beyond simply increasing scale, are being explored to overcome the limitations in AI development, and what is the potential impact of these strategies on the competitive landscape?
- The slowing progress of leading AI developers creates opportunities for competitors. Chinese companies like 01.ai and Deepseek are demonstrating progress, suggesting that innovative approaches rather than brute-force scaling may be the key to future advancements in AI. This shift necessitates a focus on data quality, specialized expertise, and novel model architectures.
Cognitive Concepts
Framing Bias
The article frames the narrative around the difficulties faced by major AI companies, emphasizing the slowdowns and challenges encountered in developing advanced AI models. This creates a sense of pessimism and may underplay the continuous progress and innovations occurring in the field. The headline, if there were one, could potentially reinforce this negative framing.
Language Bias
The language used is generally neutral, although phrases like "less réjouissantes" (less joyful/encouraging) and descriptions of the challenges as "difficulties" and setbacks subtly contribute to a more negative tone. More neutral alternatives could be used to describe the progress, such as "challenges", "obstacles", or "limitations".
Bias by Omission
The article focuses primarily on the challenges faced by leading AI companies like OpenAI, Google, and Anthropic in developing more powerful models, potentially omitting advancements or breakthroughs from smaller or less-known companies. The lack of detail on the progress of other AI models besides those mentioned could create a skewed perception of the overall field. There is no mention of the ethical considerations of increasingly powerful AI models.
False Dichotomy
The article presents a false dichotomy by suggesting that the 'rule of scale' (more data, more computing power equals better AI) is being challenged. While the article highlights difficulties, it doesn't fully explore alternative approaches or possibilities for progress beyond this rule. The implication is that either the rule is true and progress is slowing, or the rule is false and a new paradigm is needed, overlooking the possibility of incremental improvements or other factors.
Gender Bias
The article doesn't exhibit explicit gender bias. Key figures mentioned are predominantly male (Sam Altman, Dario Amodei, Kai-Fu Lee), but this may reflect the current demographic makeup of the AI industry rather than conscious bias by the author.
Sustainable Development Goals
The article discusses advancements and challenges in AI development, highlighting innovation in model architecture (e.g., decomposition of tasks, mixture of experts) and the race for more efficient AI models. This directly relates to SDG 9, which promotes building resilient infrastructure, promoting inclusive and sustainable industrialization, and fostering innovation.