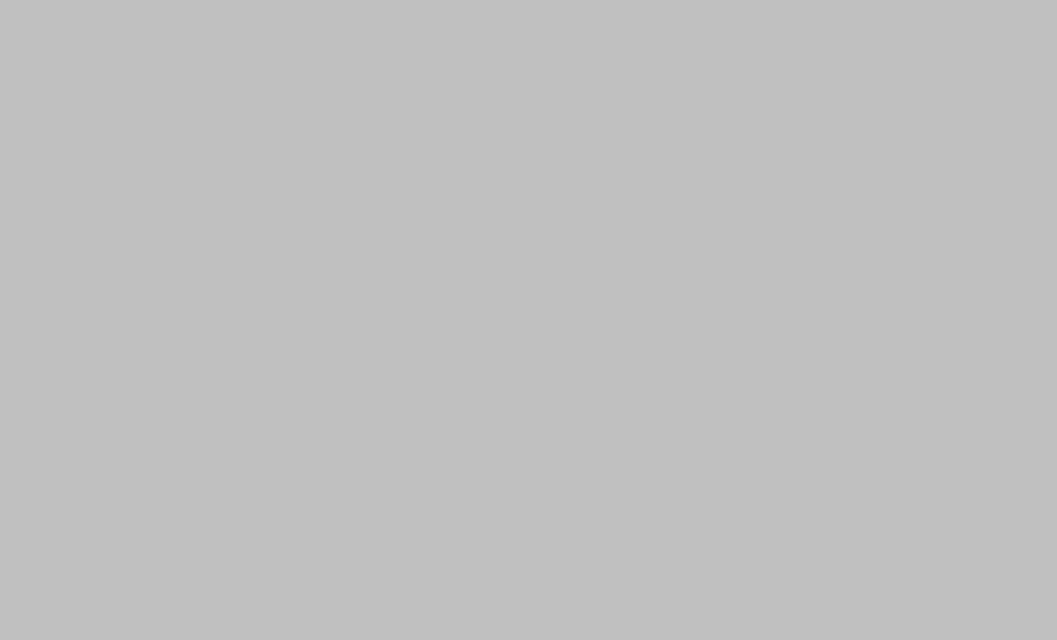
lexpress.fr
OpenAI's Orion Model Falls Short of Expectations, Slowing Progress Towards Human-Level AI
OpenAI's new language model, Orion, has failed to meet expectations, struggling with programming and reasoning tasks, slowing progress towards a human-level AI and highlighting the limitations of the scaling rule in AI development, with costs expected to reach tens of billions by 2026.
- What are the specific performance shortcomings of OpenAI's Orion model, and how do these affect the timeline for achieving human-level AI?
- OpenAI's latest language model, Orion, has fallen short of expectations, struggling with coding and reasoning tasks. Progress is evident but less significant than previous advancements. This impacts OpenAI's projected timeline for a human-level AI.
- How does the increasing cost of training large language models, potentially reaching tens of billions of dollars, influence the trajectory of AI development?
- The slowdown in progress challenges the "scale" rule in AI development, which posits that increased computing power, data, and model size inevitably lead to greater performance. This is evidenced by delays from competitors like Google (Gemini) and Anthropic (Claude 3.5 Opus). The cost of training these models is also escalating rapidly, reaching billions of dollars in the coming years.
- What innovative approaches beyond increased scale, such as post-training refinement and specialized models, are being employed to overcome current limitations in AI development?
- The limitations stem from the diminishing availability of high-quality, unexploited data for training. Synthetic data generation, while easy, lacks diversity. OpenAI is investing in agreements with content publishers for exclusive data, a slower and more expensive approach than web scraping. Innovative post-training techniques using specialized experts and "mixture-of-experts" models are being explored.
Cognitive Concepts
Framing Bias
The headline (if there was one) and introduction likely framed the story around the challenges and setbacks in AI development, potentially emphasizing the negative aspects to the exclusion of more positive developments or different approaches. The article's structure and emphasis on declining returns and increased costs could create a sense of pessimism towards the future of AI development and progress.
Language Bias
The article employs relatively neutral language but uses phrases like "less réjouissantes" (less cheerful) which may subtly influence reader perception towards a more negative view of AI progress. The phrase "butant sur" (stumbling upon) when describing AI models struggles is also more negative than necessary. More neutral language could replace them.
Bias by Omission
The article focuses heavily on the challenges faced by leading AI companies like OpenAI, Google, and Anthropic, but omits discussion of potential breakthroughs or successes from smaller or less-known AI research groups. This omission could lead readers to believe that progress in the field is universally stalled, neglecting potentially significant advancements elsewhere. The lack of diverse perspectives from within the AI community is also a notable omission.
False Dichotomy
The article presents a false dichotomy between the "rule of scale" (more data and computing power equals more progress) and the current limitations faced by major AI companies. It implies that either the rule of scale is fundamentally flawed or that current AI development is encountering an insurmountable barrier, overlooking potential intermediate solutions or alternative approaches to AI development.