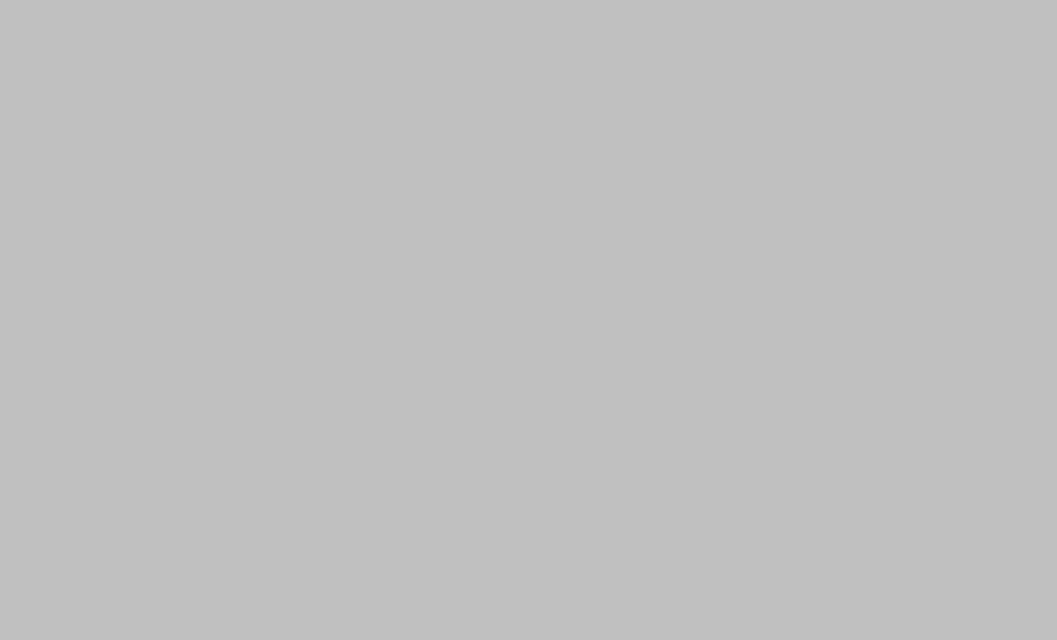
forbes.com
Pipeshift Secures $2.5M to Simplify Open-Source LLM Deployment
Pipeshift, a San Francisco startup, secured $2.5 million in seed funding to launch a platform simplifying the deployment of open-source large language models (LLMs) for enterprises, addressing the complexity and cost barriers to adoption.
- How does Pipeshift's approach compare to using proprietary LLMs, and what are the potential advantages and disadvantages of each?
- The platform's value proposition is to reduce the time, cost, and expertise needed for enterprises to utilize open-source LLMs. This allows businesses to maintain privacy, control, and potentially lower costs compared to proprietary LLMs. Early adoption by clients like NetApp shows its practicality and cost-effectiveness.
- What are the potential long-term implications of Pipeshift's platform for the GenAI market, and what challenges might it face in sustaining its growth?
- Pipeshift's success hinges on the growing preference for open-source LLMs among enterprises. By streamlining the deployment process, Pipeshift positions itself to capitalize on the anticipated increase in GenAI adoption in 2025 and beyond, potentially changing the landscape of how businesses leverage AI technologies. The company's focus on ease of use and flexibility allows businesses to adapt to the rapidly evolving GenAI field.
- What is the primary innovation offered by Pipeshift's platform, and how does it address a key challenge in the enterprise adoption of open-source LLMs?
- Pipeshift, a San Francisco-based startup, has raised $2.5 million in seed funding to develop its platform for deploying open-source large language models (LLMs). The platform aims to simplify the complex process of training, deploying, and scaling LLMs, making it more accessible to enterprises.
Cognitive Concepts
Framing Bias
The narrative strongly favors Pipeshift's platform, highlighting its benefits and downplaying potential challenges. The positive quotes from customers and investors reinforce this positive framing. The headline (if there was one, it's not included in the text) would likely further emphasize the Pipeshift story.
Language Bias
The language used is largely positive and enthusiastic about Pipeshift and its platform. Words like "revolutionize," "democratize," and "impressive" suggest a bias towards a positive portrayal. More neutral alternatives would be to use words like 'improve', 'facilitate', and 'efficient'.
Bias by Omission
The article focuses heavily on Pipeshift and its platform, potentially omitting other companies offering similar solutions or alternative approaches to deploying open-source LLMs. There is no discussion of potential downsides to open-source LLMs, such as potential security vulnerabilities or lack of consistent support.
False Dichotomy
The article presents a false dichotomy between proprietary and open-source LLMs, simplifying the choice for enterprises. It doesn't explore hybrid approaches or other options that might exist.
Gender Bias
The article includes several women in leadership positions (Anu Mangaly), but their contributions are presented in the context of their work at their respective companies, rather than focusing on their individual achievements or gender.
Sustainable Development Goals
By providing an infrastructure platform that simplifies the deployment and scaling of open-source LLMs, Pipeshift democratizes access to advanced AI technologies. This reduces the barrier to entry for smaller companies and startups that may lack the resources to develop and deploy such technologies independently, thus promoting a more equitable technological landscape. This aligns with SDG 10, which aims to reduce inequality within and among countries.