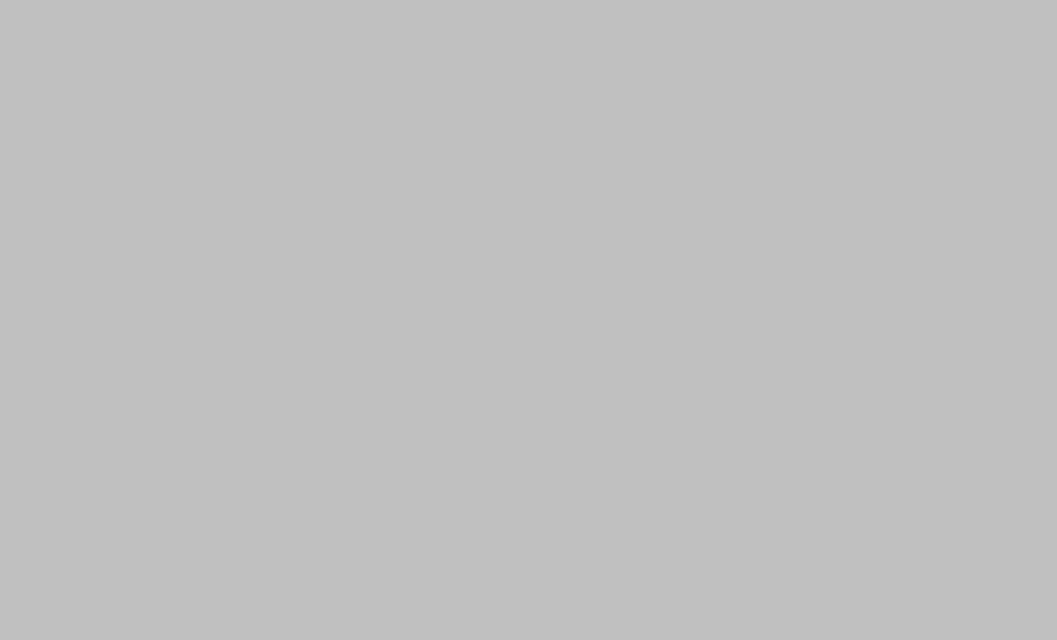
forbes.com
Sutskever's 'Data Depletion' Claim: Inaccurate and Misleading
Ilya Sutskever's claim that AI has exhausted its data supply is inaccurate; the article argues that data scarcity is context-dependent and solvable through methods like synthetic data generation, highlighting the ongoing human role in data creation.
- How can the challenges of data scarcity in specific AI applications be mitigated?
- The core issue isn't data depletion but the scarcity of useful, high-quality data for specific AI tasks. This scarcity, though it resembles resource depletion, is relative and can be addressed through methods like synthetic data generation, data augmentation, and transfer learning.
- What is the main inaccuracy in Sutskever's statement about data being the finite 'fossil fuel' of AI?
- Ilya Sutskever's claim that "Data is the fossil fuel of AI, and we used it all!" is inaccurate. While high-quality data is crucial for AI, its availability isn't universally finite. The article highlights that data scarcity is context-dependent, varying across domains and applications.
- What are the key future challenges and considerations in transforming raw data into useful, high-quality datasets for AI development?
- Future AI development hinges less on data renewability and more on effectively transforming raw data into high-quality, task-specific datasets. This involves addressing biases, ethical considerations, and ensuring data relevance, highlighting the crucial role of data curation and preprocessing.
Cognitive Concepts
Framing Bias
The framing is balanced. While the article challenges Sutskever's statement, it does so by presenting a well-reasoned argument and exploring various perspectives on data scarcity in AI. The headline (if any) would be crucial to assess the framing's potential impact.
Bias by Omission
The analysis does not present significant bias by omission. While the article focuses on the limitations of current AI data, it acknowledges the existence of methods like synthetic data generation and transfer learning to address data scarcity. The limitations of these methods are also discussed, preventing a misleadingly optimistic view.
Sustainable Development Goals
The article highlights the unsustainable nature of viewing data as a finite resource like fossil fuels. It emphasizes the need for responsible data management, including data augmentation, synthetic data generation, and transfer learning to address data scarcity. This aligns with SDG 12, which promotes sustainable consumption and production patterns, responsible resource management, and the reduction of waste.